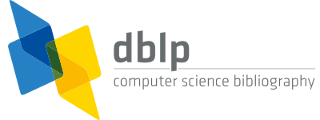


default search action
David Duvenaud
Person information
- affiliation: University of Toronto, Department of Computer Science, Canada
- affiliation (former): Harvard University, School of Engineering and Applied Sciences, Cambridge, MA, USA
- affiliation (PhD): University of Cambridge, Department of Engineering, UK
Refine list

refinements active!
zoomed in on ?? of ?? records
view refined list in
export refined list as
2020 – today
- 2024
- [c55]Mrinank Sharma, Meg Tong, Tomasz Korbak, David Duvenaud, Amanda Askell, Samuel R. Bowman, Esin Durmus, Zac Hatfield-Dodds, Scott R. Johnston, Shauna Kravec, Timothy Maxwell, Sam McCandlish, Kamal Ndousse, Oliver Rausch, Nicholas Schiefer, Da Yan, Miranda Zhang, Ethan Perez:
Towards Understanding Sycophancy in Language Models. ICLR 2024 - [c54]Daniel D. Johnson, Daniel Tarlow, David Duvenaud, Chris J. Maddison:
Experts Don't Cheat: Learning What You Don't Know By Predicting Pairs. ICML 2024 - [i56]Evan Hubinger, Carson Denison, Jesse Mu, Mike Lambert, Meg Tong, Monte MacDiarmid, Tamera Lanham, Daniel M. Ziegler, Tim Maxwell, Newton Cheng, Adam S. Jermyn, Amanda Askell, Ansh Radhakrishnan, Cem Anil, David Duvenaud, Deep Ganguli, Fazl Barez, Jack Clark, Kamal Ndousse, Kshitij Sachan, Michael Sellitto, Mrinank Sharma, Nova DasSarma, Roger Grosse, Shauna Kravec, Yuntao Bai, Zachary Witten, Marina Favaro, Jan Brauner, Holden Karnofsky, Paul F. Christiano, Samuel R. Bowman, Logan Graham, Jared Kaplan, Sören Mindermann, Ryan Greenblatt, Buck Shlegeris, Nicholas Schiefer, Ethan Perez:
Sleeper Agents: Training Deceptive LLMs that Persist Through Safety Training. CoRR abs/2401.05566 (2024) - [i55]Daniel D. Johnson, Daniel Tarlow, David Duvenaud, Chris J. Maddison:
Experts Don't Cheat: Learning What You Don't Know By Predicting Pairs. CoRR abs/2402.08733 (2024) - [i54]James Requeima, John Bronskill, Dami Choi, Richard E. Turner, David Duvenaud:
LLM Processes: Numerical Predictive Distributions Conditioned on Natural Language. CoRR abs/2405.12856 (2024) - [i53]Carson Denison, Monte MacDiarmid, Fazl Barez, David Duvenaud, Shauna Kravec, Samuel Marks, Nicholas Schiefer, Ryan Soklaski, Alex Tamkin, Jared Kaplan, Buck Shlegeris, Samuel R. Bowman, Ethan Perez, Evan Hubinger:
Sycophancy to Subterfuge: Investigating Reward-Tampering in Large Language Models. CoRR abs/2406.10162 (2024) - 2023
- [c53]Dami Choi, Yonadav Shavit, David Kristjanson Duvenaud:
Tools for Verifying Neural Models' Training Data. NeurIPS 2023 - [i52]Dami Choi, Yonadav Shavit, David Duvenaud:
Tools for Verifying Neural Models' Training Data. CoRR abs/2307.00682 (2023) - [i51]Mrinank Sharma, Meg Tong, Tomasz Korbak, David Duvenaud, Amanda Askell, Samuel R. Bowman, Newton Cheng, Esin Durmus, Zac Hatfield-Dodds, Scott R. Johnston, Shauna Kravec, Timothy Maxwell, Sam McCandlish, Kamal Ndousse, Oliver Rausch, Nicholas Schiefer, Da Yan, Miranda Zhang, Ethan Perez:
Towards Understanding Sycophancy in Language Models. CoRR abs/2310.13548 (2023) - [i50]Jack Richter-Powell, Luca A. Thiede, Alán Aspuru-Guzik, David Duvenaud:
Sorting Out Quantum Monte Carlo. CoRR abs/2311.05598 (2023) - 2022
- [c52]Winnie Xu, Ricky T. Q. Chen, Xuechen Li, David Duvenaud:
Infinitely Deep Bayesian Neural Networks with Stochastic Differential Equations. AISTATS 2022: 721-738 - [c51]Jonathan P. Lorraine, David Acuna, Paul Vicol, David Duvenaud:
Complex Momentum for Optimization in Games. AISTATS 2022: 7742-7765 - [c50]Paul Vicol, Jonathan P. Lorraine, Fabian Pedregosa, David Duvenaud, Roger B. Grosse:
On Implicit Bias in Overparameterized Bilevel Optimization. ICML 2022: 22234-22259 - [i49]Paul Vicol, Jonathan Lorraine, Fabian Pedregosa, David Duvenaud, Roger B. Grosse:
On Implicit Bias in Overparameterized Bilevel Optimization. CoRR abs/2212.14032 (2022) - [i48]David Duvenaud, Markus Heinonen, Michael Tiemann, Max Welling:
Differential Equations and Continuous-Time Deep Learning (Dagstuhl Seminar 22332). Dagstuhl Reports 12(8): 20-30 (2022) - 2021
- [j2]Adam Paszke, Daniel D. Johnson, David Duvenaud, Dimitrios Vytiniotis, Alexey Radul, Matthew J. Johnson, Jonathan Ragan-Kelley, Dougal Maclaurin:
Getting to the point: index sets and parallelism-preserving autodiff for pointful array programming. Proc. ACM Program. Lang. 5(ICFP): 1-29 (2021) - [c49]Will Sussman Grathwohl, Jacob Jin Kelly, Milad Hashemi, Mohammad Norouzi, Kevin Swersky, David Duvenaud:
No MCMC for me: Amortized sampling for fast and stable training of energy-based models. ICLR 2021 - [c48]Aniruddh Raghu, Maithra Raghu, Simon Kornblith, David Duvenaud, Geoffrey E. Hinton:
Teaching with Commentaries. ICLR 2021 - [c47]Will Grathwohl, Kevin Swersky, Milad Hashemi, David Duvenaud, Chris J. Maddison:
Oops I Took A Gradient: Scalable Sampling for Discrete Distributions. ICML 2021: 3831-3841 - [c46]Aniruddh Raghu, Jonathan Lorraine, Simon Kornblith, Matthew McDermott, David Duvenaud:
Meta-learning to Improve Pre-training. NeurIPS 2021: 23231-23244 - [i47]Will Grathwohl, Kevin Swersky, Milad Hashemi, David Duvenaud, Chris J. Maddison:
Oops I Took A Gradient: Scalable Sampling for Discrete Distributions. CoRR abs/2102.04509 (2021) - [i46]Winnie Xu, Ricky T. Q. Chen, Xuechen Li, David Duvenaud:
Infinitely Deep Bayesian Neural Networks with Stochastic Differential Equations. CoRR abs/2102.06559 (2021) - [i45]Jonathan Lorraine
, David Acuna, Paul Vicol, David Duvenaud:
Complex Momentum for Learning in Games. CoRR abs/2102.08431 (2021) - [i44]Adam Paszke, Daniel D. Johnson, David Duvenaud, Dimitrios Vytiniotis, Alexey Radul, Matthew J. Johnson, Jonathan Ragan-Kelley, Dougal Maclaurin:
Getting to the Point. Index Sets and Parallelism-Preserving Autodiff for Pointful Array Programming. CoRR abs/2104.05372 (2021) - [i43]Aniruddh Raghu, Jonathan Lorraine, Simon Kornblith, Matthew McDermott, David Duvenaud:
Meta-Learning to Improve Pre-Training. CoRR abs/2111.01754 (2021) - 2020
- [c45]Jonathan Lorraine, Paul Vicol, David Duvenaud:
Optimizing Millions of Hyperparameters by Implicit Differentiation. AISTATS 2020: 1540-1552 - [c44]Xuechen Li, Ting-Kam Leonard Wong, Ricky T. Q. Chen, David Duvenaud:
Scalable Gradients for Stochastic Differential Equations. AISTATS 2020: 3870-3882 - [c43]Ricky T. Q. Chen, Dami Choi, Lukas Balles, David Duvenaud, Philipp Hennig:
Self-Tuning Stochastic Optimization with Curvature-Aware Gradient Filtering. ICBINB@NeurIPS 2020: 60-69 - [c42]Will Grathwohl, Kuan-Chieh Wang, Jörn-Henrik Jacobsen, David Duvenaud, Mohammad Norouzi, Kevin Swersky:
Your classifier is secretly an energy based model and you should treat it like one. ICLR 2020 - [c41]Yucen Luo, Alex Beatson, Mohammad Norouzi, Jun Zhu, David Duvenaud, Ryan P. Adams, Ricky T. Q. Chen:
SUMO: Unbiased Estimation of Log Marginal Probability for Latent Variable Models. ICLR 2020 - [c40]Will Grathwohl, Kuan-Chieh Wang, Jörn-Henrik Jacobsen, David Duvenaud, Richard S. Zemel:
Learning the Stein Discrepancy for Training and Evaluating Energy-Based Models without Sampling. ICML 2020: 3732-3747 - [c39]Jacob Kelly, Jesse Bettencourt, Matthew J. Johnson, David Duvenaud:
Learning Differential Equations that are Easy to Solve. NeurIPS 2020 - [c38]Sana Tonekaboni, Shalmali Joshi, Kieran Campbell, David Duvenaud, Anna Goldenberg:
What went wrong and when? Instance-wise feature importance for time-series black-box models. NeurIPS 2020 - [i42]Xuechen Li, Ting-Kam Leonard Wong, Ricky T. Q. Chen, David Duvenaud:
Scalable Gradients for Stochastic Differential Equations. CoRR abs/2001.01328 (2020) - [i41]Will Grathwohl, Kuan-Chieh Wang, Jörn-Henrik Jacobsen, David Duvenaud, Richard S. Zemel:
Cutting out the Middle-Man: Training and Evaluating Energy-Based Models without Sampling. CoRR abs/2002.05616 (2020) - [i40]Sana Tonekaboni, Shalmali Joshi, David Duvenaud, Anna Goldenberg:
What went wrong and when? Instance-wise Feature Importance for Time-series Models. CoRR abs/2003.02821 (2020) - [i39]Yucen Luo, Alex Beatson, Mohammad Norouzi, Jun Zhu, David Duvenaud, Ryan P. Adams, Ricky T. Q. Chen:
SUMO: Unbiased Estimation of Log Marginal Probability for Latent Variable Models. CoRR abs/2004.00353 (2020) - [i38]Jacob Kelly, Jesse Bettencourt, Matthew James Johnson, David Duvenaud:
Learning Differential Equations that are Easy to Solve. CoRR abs/2007.04504 (2020) - [i37]Fartash Faghri, David Duvenaud, David J. Fleet, Jimmy Ba:
A Study of Gradient Variance in Deep Learning. CoRR abs/2007.04532 (2020) - [i36]Will Grathwohl, Jacob Kelly, Milad Hashemi, Mohammad Norouzi, Kevin Swersky, David Duvenaud:
No MCMC for me: Amortized sampling for fast and stable training of energy-based models. CoRR abs/2010.04230 (2020) - [i35]Aniruddh Raghu, Maithra Raghu, Simon Kornblith, David Duvenaud, Geoffrey E. Hinton:
Teaching with Commentaries. CoRR abs/2011.03037 (2020) - [i34]Ricky T. Q. Chen, Dami Choi, Lukas Balles, David Duvenaud, Philipp Hennig:
Self-Tuning Stochastic Optimization with Curvature-Aware Gradient Filtering. CoRR abs/2011.04803 (2020)
2010 – 2019
- 2019
- [j1]Lawson Fulton, Vismay Modi, David Duvenaud, David I. W. Levin, Alec Jacobson:
Latent-space Dynamics for Reduced Deformable Simulation. Comput. Graph. Forum 38(2): 379-391 (2019) - [c37]Xuechen Li, Ting-Kam Leonard Wong, Ricky T. Q. Chen, David Duvenaud:
Scalable Gradients and Variational Inference for Stochastic Differential Equations. AABI 2019: 1-28 - [c36]Kawin Ethayarajh, David Duvenaud, Graeme Hirst:
Understanding Undesirable Word Embedding Associations. ACL (1) 2019: 1696-1705 - [c35]Kawin Ethayarajh, David Duvenaud, Graeme Hirst:
Towards Understanding Linear Word Analogies. ACL (1) 2019: 3253-3262 - [c34]Chun-Hao Chang, Elliot Creager, Anna Goldenberg, David Duvenaud:
Explaining Image Classifiers by Counterfactual Generation. ICLR (Poster) 2019 - [c33]Will Grathwohl, Ricky T. Q. Chen, Jesse Bettencourt, Ilya Sutskever, David Duvenaud:
FFJORD: Free-Form Continuous Dynamics for Scalable Reversible Generative Models. ICLR 2019 - [c32]Matthew MacKay, Paul Vicol, Jonathan Lorraine, David Duvenaud, Roger B. Grosse:
Self-Tuning Networks: Bilevel Optimization of Hyperparameters using Structured Best-Response Functions. ICLR (Poster) 2019 - [c31]Jens Behrmann, Will Grathwohl, Ricky T. Q. Chen, David Duvenaud, Jörn-Henrik Jacobsen:
Invertible Residual Networks. ICML 2019: 573-582 - [c30]Renjie Liao, Yujia Li, Yang Song, Shenlong Wang, William L. Hamilton, David Duvenaud, Raquel Urtasun, Richard S. Zemel:
Efficient Graph Generation with Graph Recurrent Attention Networks. NeurIPS 2019: 4257-4267 - [c29]Yulia Rubanova, Tian Qi Chen, David Duvenaud:
Latent Ordinary Differential Equations for Irregularly-Sampled Time Series. NeurIPS 2019: 5321-5331 - [c28]Tian Qi Chen, Jens Behrmann, David Duvenaud, Jörn-Henrik Jacobsen:
Residual Flows for Invertible Generative Modeling. NeurIPS 2019: 9913-9923 - [c27]Tian Qi Chen, David Duvenaud:
Neural Networks with Cheap Differential Operators. NeurIPS 2019: 9961-9971 - [i33]Matthew MacKay, Paul Vicol, Jonathan Lorraine, David Duvenaud, Roger B. Grosse:
Self-Tuning Networks: Bilevel Optimization of Hyperparameters using Structured Best-Response Functions. CoRR abs/1903.03088 (2019) - [i32]Ricky T. Q. Chen, Jens Behrmann, David Duvenaud, Jörn-Henrik Jacobsen:
Residual Flows for Invertible Generative Modeling. CoRR abs/1906.02735 (2019) - [i31]Yulia Rubanova, Ricky T. Q. Chen, David Duvenaud:
Latent ODEs for Irregularly-Sampled Time Series. CoRR abs/1907.03907 (2019) - [i30]Kawin Ethayarajh, David Duvenaud, Graeme Hirst:
Understanding Undesirable Word Embedding Associations. CoRR abs/1908.06361 (2019) - [i29]Renjie Liao, Yujia Li, Yang Song, Shenlong Wang, Charlie Nash, William L. Hamilton, David Duvenaud, Raquel Urtasun, Richard S. Zemel:
Efficient Graph Generation with Graph Recurrent Attention Networks. CoRR abs/1910.00760 (2019) - [i28]Jonathan Lorraine
, Paul Vicol, David Duvenaud:
Optimizing Millions of Hyperparameters by Implicit Differentiation. CoRR abs/1911.02590 (2019) - [i27]Will Grathwohl, Kuan-Chieh Wang, Jörn-Henrik Jacobsen, David Duvenaud, Mohammad Norouzi, Kevin Swersky:
Your Classifier is Secretly an Energy Based Model and You Should Treat it Like One. CoRR abs/1912.03263 (2019) - [i26]Ricky T. Q. Chen, David Duvenaud:
Neural Networks with Cheap Differential Operators. CoRR abs/1912.03579 (2019) - 2018
- [c26]Tian Qi Chen, Xuechen Li, Roger B. Grosse, David Duvenaud:
Isolating Sources of Disentanglement in Variational Autoencoders. ICLR (Workshop) 2018 - [c25]Will Grathwohl, Dami Choi, Yuhuai Wu, Geoffrey Roeder, David Duvenaud:
Backpropagation through the Void: Optimizing control variates for black-box gradient estimation. ICLR (Poster) 2018 - [c24]Zachary Nado, Jasper Snoek, Roger B. Grosse, David Duvenaud, Bowen Xu, James Martens:
Stochastic Gradient Langevin dynamics that Exploit Neural Network Structure. ICLR (Workshop) 2018 - [c23]Chris Cremer, Xuechen Li, David Duvenaud:
Inference Suboptimality in Variational Autoencoders. ICML 2018: 1086-1094 - [c22]Guodong Zhang, Shengyang Sun, David Duvenaud, Roger B. Grosse:
Noisy Natural Gradient as Variational Inference. ICML 2018: 5847-5856 - [c21]Tian Qi Chen, Xuechen Li, Roger B. Grosse, David Duvenaud:
Isolating Sources of Disentanglement in Variational Autoencoders. NeurIPS 2018: 2615-2625 - [c20]Tian Qi Chen, Yulia Rubanova, Jesse Bettencourt, David Duvenaud:
Neural Ordinary Differential Equations. NeurIPS 2018: 6572-6583 - [i25]Chris Cremer, Xuechen Li, David Duvenaud:
Inference Suboptimality in Variational Autoencoders. CoRR abs/1801.03558 (2018) - [i24]Tian Qi Chen, Xuechen Li, Roger B. Grosse, David Duvenaud:
Isolating Sources of Disentanglement in Variational Autoencoders. CoRR abs/1802.04942 (2018) - [i23]Jonathan Lorraine
, David Duvenaud:
Stochastic Hyperparameter Optimization through Hypernetworks. CoRR abs/1802.09419 (2018) - [i22]Tian Qi Chen, Yulia Rubanova, Jesse Bettencourt, David Duvenaud:
Neural Ordinary Differential Equations. CoRR abs/1806.07366 (2018) - [i21]Elias Tragas, Calvin Luo, Maxime Gazeau, Kevin Luk, David Duvenaud:
Scalable Recommender Systems through Recursive Evidence Chains. CoRR abs/1807.02150 (2018) - [i20]Chun-Hao Chang, Elliot Creager, Anna Goldenberg, David Duvenaud:
Explaining Image Classifiers by Adaptive Dropout and Generative In-filling. CoRR abs/1807.08024 (2018) - [i19]George-Alexandru Adam, Petr Smirnov, Anna Goldenberg, David Duvenaud, Benjamin Haibe-Kains:
Stochastic Combinatorial Ensembles for Defending Against Adversarial Examples. CoRR abs/1808.06645 (2018) - [i18]Will Grathwohl, Ricky T. Q. Chen, Jesse Bettencourt, Ilya Sutskever, David Duvenaud:
FFJORD: Free-form Continuous Dynamics for Scalable Reversible Generative Models. CoRR abs/1810.01367 (2018) - [i17]Kawin Ethayarajh, David Duvenaud, Graeme Hirst:
Towards Understanding Linear Word Analogies. CoRR abs/1810.04882 (2018) - [i16]Jens Behrmann, David Duvenaud, Jörn-Henrik Jacobsen:
Invertible Residual Networks. CoRR abs/1811.00995 (2018) - 2017
- [c19]Chris Cremer, Quaid Morris, David Duvenaud:
Reinterpreting Importance-Weighted Autoencoders. ICLR (Workshop) 2017 - [c18]Geoffrey Roeder, Yuhuai Wu, David Duvenaud:
Sticking the Landing: Simple, Lower-Variance Gradient Estimators for Variational Inference. NIPS 2017: 6925-6934 - [i15]Geoffrey Roeder, Yuhuai Wu, David Duvenaud:
Sticking the Landing: An Asymptotically Zero-Variance Gradient Estimator for Variational Inference. CoRR abs/1703.09194 (2017) - [i14]Will Grathwohl, Dami Choi, Yuhuai Wu, Geoffrey Roeder, David Duvenaud:
Backpropagation through the Void: Optimizing control variates for black-box gradient estimation. CoRR abs/1711.00123 (2017) - [i13]Guodong Zhang, Shengyang Sun, David Duvenaud, Roger B. Grosse:
Noisy Natural Gradient as Variational Inference. CoRR abs/1712.02390 (2017) - [i12]Nathan Killoran, Leo J. Lee, Andrew Delong, David Duvenaud, Brendan J. Frey:
Generating and designing DNA with deep generative models. CoRR abs/1712.06148 (2017) - 2016
- [c17]David Duvenaud, Dougal Maclaurin, Ryan P. Adams:
Early Stopping as Nonparametric Variational Inference. AISTATS 2016: 1070-1077 - [c16]Cheng-Zhi Anna Huang, David Duvenaud, Krzysztof Z. Gajos:
ChordRipple: Recommending Chords to Help Novice Composers Go Beyond the Ordinary. IUI 2016: 241-250 - [c15]Matthew J. Johnson, David Duvenaud, Alexander B. Wiltschko, Ryan P. Adams, Sandeep R. Datta:
Composing graphical models with neural networks for structured representations and fast inference. NIPS 2016: 2946-2954 - [c14]Eric Schulz, Josh Tenenbaum, David Duvenaud, Maarten Speekenbrink, Samuel J. Gershman:
Probing the Compositionality of Intuitive Functions. NIPS 2016: 3729-3737 - [i11]Rafael Gómez-Bombarelli, David Duvenaud, José Miguel Hernández-Lobato, Jorge Aguilera-Iparraguirre, Timothy D. Hirzel, Ryan P. Adams, Alán Aspuru-Guzik:
Automatic chemical design using a data-driven continuous representation of molecules. CoRR abs/1610.02415 (2016) - 2015
- [c13]Dougal Maclaurin, David Duvenaud, Ryan P. Adams:
Gradient-based Hyperparameter Optimization through Reversible Learning. ICML 2015: 2113-2122 - [c12]David Duvenaud, Dougal Maclaurin, Jorge Aguilera-Iparraguirre, Rafael Gómez-Bombarelli, Timothy Hirzel, Alán Aspuru-Guzik, Ryan P. Adams:
Convolutional Networks on Graphs for Learning Molecular Fingerprints. NIPS 2015: 2224-2232 - [i10]Dougal Maclaurin, David Duvenaud, Ryan P. Adams:
Gradient-based Hyperparameter Optimization through Reversible Learning. CoRR abs/1502.03492 (2015) - [i9]Dougal Maclaurin, David Duvenaud, Ryan P. Adams:
Early Stopping is Nonparametric Variational Inference. CoRR abs/1504.01344 (2015) - [i8]David Duvenaud, Dougal Maclaurin, Jorge Aguilera-Iparraguirre, Rafael Gómez-Bombarelli, Timothy Hirzel, Alán Aspuru-Guzik, Ryan P. Adams:
Convolutional Networks on Graphs for Learning Molecular Fingerprints. CoRR abs/1509.09292 (2015) - 2014
- [c11]James Robert Lloyd, David Duvenaud, Roger B. Grosse, Joshua B. Tenenbaum, Zoubin Ghahramani:
Automatic Construction and Natural-Language Description of Nonparametric Regression Models. AAAI 2014: 1242-1250 - [c10]David Duvenaud, Oren Rippel, Ryan P. Adams, Zoubin Ghahramani:
Avoiding pathologies in very deep networks. AISTATS 2014: 202-210 - [c9]Cheng-Zhi Anna Huang, David Duvenaud, Kenneth C. Arnold, Brenton Partridge, Josiah W. Oberholtzer, Krzysztof Z. Gajos:
Active learning of intuitive control knobs for synthesizers using gaussian processes. IUI 2014: 115-124 - [c8]Michael Schober, David Duvenaud, Philipp Hennig:
Probabilistic ODE Solvers with Runge-Kutta Means. NIPS 2014: 739-747 - [i7]James Robert Lloyd, David Duvenaud, Roger B. Grosse, Joshua B. Tenenbaum, Zoubin Ghahramani:
Automatic Construction and Natural-Language Description of Nonparametric Regression Models. CoRR abs/1402.4304 (2014) - [i6]David Duvenaud, Oren Rippel, Ryan P. Adams, Zoubin Ghahramani:
Avoiding pathologies in very deep networks. CoRR abs/1402.5836 (2014) - [i5]Michael Schober, David Duvenaud, Philipp Hennig:
Probabilistic ODE Solvers with Runge-Kutta Means. CoRR abs/1406.2582 (2014) - [i4]Tomoharu Iwata, David Duvenaud, Zoubin Ghahramani:
Warped Mixtures for Nonparametric Cluster Shapes. CoRR abs/1408.2061 (2014) - [i3]Roger B. Grosse, David Kristjanson Duvenaud:
Testing MCMC code. CoRR abs/1412.5218 (2014) - 2013
- [c7]David Duvenaud, James Robert Lloyd, Roger B. Grosse, Joshua B. Tenenbaum, Zoubin Ghahramani:
Structure Discovery in Nonparametric Regression through Compositional Kernel Search. ICML (3) 2013: 1166-1174 - [c6]Tomoharu Iwata, David Duvenaud, Zoubin Ghahramani:
Warped Mixtures for Nonparametric Cluster Shapes. UAI 2013 - [i2]David Duvenaud, James Robert Lloyd, Roger B. Grosse, Joshua B. Tenenbaum, Zoubin Ghahramani:
Structure Discovery in Nonparametric Regression through Compositional Kernel Search. CoRR abs/1302.4922 (2013) - 2012
- [c5]Michael A. Osborne, David Duvenaud, Roman Garnett, Carl E. Rasmussen, Stephen J. Roberts, Zoubin Ghahramani:
Active Learning of Model Evidence Using Bayesian Quadrature. NIPS 2012: 46-54 - [c4]Ferenc Huszar, David Duvenaud:
Optimally-Weighted Herding is Bayesian Quadrature. UAI 2012: 377-386 - 2011
- [c3]David Duvenaud, Benjamin M. Marlin
, Kevin P. Murphy:
Multiscale Conditional Random Fields for Semi-supervised Labeling and Classification. CRV 2011: 371-378 - [c2]David Duvenaud, Hannes Nickisch, Carl Edward Rasmussen:
Additive Gaussian Processes. NIPS 2011: 226-234 - [i1]David Duvenaud, Hannes Nickisch, Carl Edward Rasmussen:
Additive Gaussian Processes. CoRR abs/1112.4394 (2011) - 2010
- [c1]David Duvenaud, Daniel Eaton, Kevin P. Murphy, Mark Schmidt:
Causal learning without DAGs. NIPS Causality: Objectives and Assessment 2010: 177-190
Coauthor Index

manage site settings
To protect your privacy, all features that rely on external API calls from your browser are turned off by default. You need to opt-in for them to become active. All settings here will be stored as cookies with your web browser. For more information see our F.A.Q.
Unpaywalled article links
Add open access links from to the list of external document links (if available).
Privacy notice: By enabling the option above, your browser will contact the API of unpaywall.org to load hyperlinks to open access articles. Although we do not have any reason to believe that your call will be tracked, we do not have any control over how the remote server uses your data. So please proceed with care and consider checking the Unpaywall privacy policy.
Archived links via Wayback Machine
For web page which are no longer available, try to retrieve content from the of the Internet Archive (if available).
Privacy notice: By enabling the option above, your browser will contact the API of archive.org to check for archived content of web pages that are no longer available. Although we do not have any reason to believe that your call will be tracked, we do not have any control over how the remote server uses your data. So please proceed with care and consider checking the Internet Archive privacy policy.
Reference lists
Add a list of references from ,
, and
to record detail pages.
load references from crossref.org and opencitations.net
Privacy notice: By enabling the option above, your browser will contact the APIs of crossref.org, opencitations.net, and semanticscholar.org to load article reference information. Although we do not have any reason to believe that your call will be tracked, we do not have any control over how the remote server uses your data. So please proceed with care and consider checking the Crossref privacy policy and the OpenCitations privacy policy, as well as the AI2 Privacy Policy covering Semantic Scholar.
Citation data
Add a list of citing articles from and
to record detail pages.
load citations from opencitations.net
Privacy notice: By enabling the option above, your browser will contact the API of opencitations.net and semanticscholar.org to load citation information. Although we do not have any reason to believe that your call will be tracked, we do not have any control over how the remote server uses your data. So please proceed with care and consider checking the OpenCitations privacy policy as well as the AI2 Privacy Policy covering Semantic Scholar.
OpenAlex data
Load additional information about publications from .
Privacy notice: By enabling the option above, your browser will contact the API of openalex.org to load additional information. Although we do not have any reason to believe that your call will be tracked, we do not have any control over how the remote server uses your data. So please proceed with care and consider checking the information given by OpenAlex.
last updated on 2024-09-04 00:28 CEST by the dblp team
all metadata released as open data under CC0 1.0 license
see also: Terms of Use | Privacy Policy | Imprint