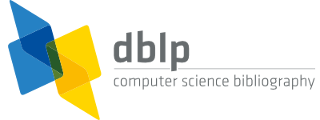


default search action
Alekh Agarwal
Person information
Refine list

refinements active!
zoomed in on ?? of ?? records
view refined list in
export refined list as
2020 – today
- 2025
- [i87]Alekh Agarwal, Christoph Dann, Teodor V. Marinov:
Design Considerations in Offline Preference-based RL. CoRR abs/2502.06861 (2025) - [i86]Jincheng Mei, Bo Dai, Alekh Agarwal, Sharan Vaswani, Anant Raj, Csaba Szepesvári, Dale Schuurmans:
Small steps no more: Global convergence of stochastic gradient bandits for arbitrary learning rates. CoRR abs/2502.07141 (2025) - 2024
- [j13]Aditya Modi, Jinglin Chen, Akshay Krishnamurthy, Nan Jiang, Alekh Agarwal:
Model-Free Representation Learning and Exploration in Low-Rank MDPs. J. Mach. Learn. Res. 25: 6:1-6:76 (2024) - [c88]Jacob D. Abernethy, Alekh Agarwal, Teodor Vanislavov Marinov, Manfred K. Warmuth:
A Mechanism for Sample-Efficient In-Context Learning for Sparse Retrieval Tasks. ALT 2024: 3-46 - [c87]Kaiwen Wang, Rahul Kidambi, Ryan Sullivan, Alekh Agarwal, Christoph Dann, Andrea Michi, Marco Gelmi, Yunxuan Li, Raghav Gupta, Kumar Dubey, Alexandre Ramé, Johan Ferret, Geoffrey Cideron, Le Hou, Hongkun Yu, Amr Ahmed, Aranyak Mehta, Léonard Hussenot, Olivier Bachem, Edouard Leurent:
Conditional Language Policy: A General Framework For Steerable Multi-Objective Finetuning. EMNLP (Findings) 2024: 2153-2186 - [c86]Alekh Agarwal, Jian Qian, Alexander Rakhlin, Tong Zhang:
The Non-linear F-Design and Applications to Interactive Learning. ICML 2024 - [c85]Gokul Swamy, Christoph Dann, Rahul Kidambi, Steven Wu, Alekh Agarwal:
A Minimaximalist Approach to Reinforcement Learning from Human Feedback. ICML 2024 - [c84]Kaiwen Wang, Owen Oertell, Alekh Agarwal, Nathan Kallus, Wen Sun:
More Benefits of Being Distributional: Second-Order Bounds for Reinforcement Learning. ICML 2024 - [c83]Wang Zhu, Alekh Agarwal, Mandar Joshi, Robin Jia, Jesse Thomason, Kristina Toutanova:
Efficient End-to-End Visual Document Understanding with Rationale Distillation. NAACL-HLT 2024: 8401-8424 - [c82]Jincheng Mei, Bo Dai, Alekh Agarwal, Sharan Vaswani, Anant Raj, Csaba Szepesvári, Dale Schuurmans:
Small steps no more: Global convergence of stochastic gradient bandits for arbitrary learning rates. NeurIPS 2024 - [i85]Ahmad Beirami, Alekh Agarwal, Jonathan Berant, Alexander D'Amour, Jacob Eisenstein, Chirag Nagpal, Ananda Theertha Suresh:
Theoretical guarantees on the best-of-n alignment policy. CoRR abs/2401.01879 (2024) - [i84]Gokul Swamy, Christoph Dann, Rahul Kidambi, Zhiwei Steven Wu, Alekh Agarwal:
A Minimaximalist Approach to Reinforcement Learning from Human Feedback. CoRR abs/2401.04056 (2024) - [i83]Kaiwen Wang, Owen Oertell, Alekh Agarwal, Nathan Kallus, Wen Sun:
More Benefits of Being Distributional: Second-Order Bounds for Reinforcement Learning. CoRR abs/2402.07198 (2024) - [i82]Jincheng Mei, Zixin Zhong, Bo Dai, Alekh Agarwal, Csaba Szepesvári, Dale Schuurmans:
Stochastic Gradient Succeeds for Bandits. CoRR abs/2402.17235 (2024) - [i81]Teodor V. Marinov, Alekh Agarwal, Mircea Trofin:
Offline Imitation Learning from Multiple Baselines with Applications to Compiler Optimization. CoRR abs/2403.19462 (2024) - [i80]Adam Fisch, Jacob Eisenstein, Vicky Zayats, Alekh Agarwal, Ahmad Beirami, Chirag Nagpal, Petet Shaw, Jonathan Berant:
Robust Preference Optimization through Reward Model Distillation. CoRR abs/2405.19316 (2024) - [i79]Kaiwen Wang, Rahul Kidambi, Ryan Sullivan, Alekh Agarwal, Christoph Dann, Andrea Michi, Marco Gelmi, Yunxuan Li, Raghav Gupta, Avinava Dubey, Alexandre Ramé, Johan Ferret, Geoffrey Cideron, Le Hou, Hongkun Yu, Amr Ahmed, Aranyak Mehta, Léonard Hussenot, Olivier Bachem, Edouard Leurent:
Conditioned Language Policy: A General Framework for Steerable Multi-Objective Finetuning. CoRR abs/2407.15762 (2024) - [i78]Amrith Setlur, Chirag Nagpal, Adam Fisch, Xinyang Geng, Jacob Eisenstein, Rishabh Agarwal, Alekh Agarwal, Jonathan Berant, Aviral Kumar:
Rewarding Progress: Scaling Automated Process Verifiers for LLM Reasoning. CoRR abs/2410.08146 (2024) - [i77]Navodita Sharma, Vishnu Vinod, Abhradeep Thakurta, Alekh Agarwal, Borja Balle, Christoph Dann, Aravindan Raghuveer:
Preserving Expert-Level Privacy in Offline Reinforcement Learning. CoRR abs/2411.13598 (2024) - 2023
- [c81]Alekh Agarwal, Yujia Jin, Tong Zhang:
VOQL: Towards Optimal Regret in Model-free RL with Nonlinear Function Approximation. COLT 2023: 987-1063 - [c80]Alekh Agarwal, Yuda Song, Wen Sun, Kaiwen Wang, Mengdi Wang, Xuezhou Zhang:
Provable Benefits of Representational Transfer in Reinforcement Learning. COLT 2023: 2114-2187 - [c79]Jonathan Lee, Alekh Agarwal, Christoph Dann, Tong Zhang:
Learning in POMDPs is Sample-Efficient with Hindsight Observability. ICML 2023: 18733-18773 - [c78]Jincheng Mei, Zixin Zhong, Bo Dai, Alekh Agarwal, Csaba Szepesvári, Dale Schuurmans:
Stochastic Gradient Succeeds for Bandits. ICML 2023: 24325-24360 - [c77]Jincheng Mei, Bo Dai, Alekh Agarwal, Mohammad Ghavamzadeh, Csaba Szepesvári, Dale Schuurmans:
Ordering-based Conditions for Global Convergence of Policy Gradient Methods. NeurIPS 2023 - [i76]Jonathan N. Lee, Alekh Agarwal, Christoph Dann, Tong Zhang:
Learning in POMDPs is Sample-Efficient with Hindsight Observability. CoRR abs/2301.13857 (2023) - [i75]Alekh Agarwal, Claudio Gentile, Teodor V. Marinov:
Leveraging User-Triggered Supervision in Contextual Bandits. CoRR abs/2302.03784 (2023) - [i74]Alekh Agarwal, H. Brendan McMahan, Zheng Xu:
An Empirical Evaluation of Federated Contextual Bandit Algorithms. CoRR abs/2303.10218 (2023) - [i73]Jacob D. Abernethy, Alekh Agarwal, Teodor V. Marinov, Manfred K. Warmuth:
A Mechanism for Sample-Efficient In-Context Learning for Sparse Retrieval Tasks. CoRR abs/2305.17040 (2023) - [i72]Alexander Goldberg, Ivan Stelmakh, Kyunghyun Cho, Alice H. Oh, Alekh Agarwal, Danielle Belgrave, Nihar B. Shah:
Peer Reviews of Peer Reviews: A Randomized Controlled Trial and Other Experiments. CoRR abs/2311.09497 (2023) - [i71]Wang Zhu, Alekh Agarwal, Mandar Joshi, Robin Jia, Jesse Thomason, Kristina Toutanova:
Efficient End-to-End Visual Document Understanding with Rationale Distillation. CoRR abs/2311.09612 (2023) - [i70]Jacob Eisenstein, Chirag Nagpal, Alekh Agarwal, Ahmad Beirami, Alex D'Amour, Dj Dvijotham, Adam Fisch, Katherine A. Heller, Stephen Pfohl, Deepak Ramachandran, Peter Shaw, Jonathan Berant:
Helping or Herding? Reward Model Ensembles Mitigate but do not Eliminate Reward Hacking. CoRR abs/2312.09244 (2023) - 2022
- [c76]Alekh Agarwal, Tong Zhang:
Minimax Regret Optimization for Robust Machine Learning under Distribution Shift. COLT 2022: 2704-2729 - [c75]Alekh Agarwal, Tong Zhang:
Non-Linear Reinforcement Learning in Large Action Spaces: Structural Conditions and Sample-efficiency of Posterior Sampling. COLT 2022: 2776-2814 - [c74]Yonathan Efroni, Dipendra Misra, Akshay Krishnamurthy, Alekh Agarwal, John Langford:
Provably Filtering Exogenous Distractors using Multistep Inverse Dynamics. ICLR 2022 - [c73]Ching-An Cheng, Tengyang Xie, Nan Jiang, Alekh Agarwal:
Adversarially Trained Actor Critic for Offline Reinforcement Learning. ICML 2022: 3852-3878 - [c72]Xuezhou Zhang, Yuda Song, Masatoshi Uehara, Mengdi Wang, Alekh Agarwal, Wen Sun:
Efficient Reinforcement Learning in Block MDPs: A Model-free Representation Learning approach. ICML 2022: 26517-26547 - [c71]Alekh Agarwal, Tong Zhang:
Model-based RL with Optimistic Posterior Sampling: Structural Conditions and Sample Complexity. NeurIPS 2022 - [c70]Jinglin Chen, Aditya Modi, Akshay Krishnamurthy, Nan Jiang, Alekh Agarwal:
On the Statistical Efficiency of Reward-Free Exploration in Non-Linear RL. NeurIPS 2022 - [i69]Xuezhou Zhang, Yuda Song, Masatoshi Uehara, Mengdi Wang, Alekh Agarwal, Wen Sun:
Efficient Reinforcement Learning in Block MDPs: A Model-free Representation Learning Approach. CoRR abs/2202.00063 (2022) - [i68]Ching-An Cheng, Tengyang Xie, Nan Jiang, Alekh Agarwal:
Adversarially Trained Actor Critic for Offline Reinforcement Learning. CoRR abs/2202.02446 (2022) - [i67]Alekh Agarwal, Tong Zhang:
Minimax Regret Optimization for Robust Machine Learning under Distribution Shift. CoRR abs/2202.05436 (2022) - [i66]Alekh Agarwal, Tong Zhang:
Non-Linear Reinforcement Learning in Large Action Spaces: Structural Conditions and Sample-efficiency of Posterior Sampling. CoRR abs/2203.08248 (2022) - [i65]Alekh Agarwal, Yuda Song
, Wen Sun, Kaiwen Wang, Mengdi Wang
, Xuezhou Zhang:
Provable Benefits of Representational Transfer in Reinforcement Learning. CoRR abs/2205.14571 (2022) - [i64]Alekh Agarwal, Tong Zhang:
Model-based RL with Optimistic Posterior Sampling: Structural Conditions and Sample Complexity. CoRR abs/2206.07659 (2022) - [i63]Jinglin Chen, Aditya Modi, Akshay Krishnamurthy, Nan Jiang, Alekh Agarwal:
On the Statistical Efficiency of Reward-Free Exploration in Non-Linear RL. CoRR abs/2206.10770 (2022) - [i62]Alekh Agarwal, Yujia Jin, Tong Zhang:
VOQL: Towards Optimal Regret in Model-free RL with Nonlinear Function Approximation. CoRR abs/2212.06069 (2022) - 2021
- [j12]Alekh Agarwal, Sham M. Kakade, Jason D. Lee, Gaurav Mahajan:
On the Theory of Policy Gradient Methods: Optimality, Approximation, and Distribution Shift. J. Mach. Learn. Res. 22: 98:1-98:76 (2021) - [j11]Alberto Bietti, Alekh Agarwal, John Langford:
A Contextual Bandit Bake-off. J. Mach. Learn. Res. 22: 133:1-133:49 (2021) - [c69]Juan C. Perdomo, Max Simchowitz, Alekh Agarwal, Peter L. Bartlett:
Towards a Dimension-Free Understanding of Adaptive Linear Control. COLT 2021: 3681-3770 - [c68]Andrea Zanette, Ching-An Cheng, Alekh Agarwal:
Cautiously Optimistic Policy Optimization and Exploration with Linear Function Approximation. COLT 2021: 4473-4525 - [c67]Fei Feng, Wotao Yin, Alekh Agarwal, Lin Yang
:
Provably Correct Optimization and Exploration with Non-linear Policies. ICML 2021: 3263-3273 - [c66]Tengyang Xie, Ching-An Cheng, Nan Jiang, Paul Mineiro, Alekh Agarwal:
Bellman-consistent Pessimism for Offline Reinforcement Learning. NeurIPS 2021: 6683-6694 - [i61]Aditya Modi, Jinglin Chen, Akshay Krishnamurthy, Nan Jiang, Alekh Agarwal:
Model-free Representation Learning and Exploration in Low-rank MDPs. CoRR abs/2102.07035 (2021) - [i60]Juan C. Perdomo, Max Simchowitz, Alekh Agarwal, Peter L. Bartlett:
Towards a Dimension-Free Understanding of Adaptive Linear Control. CoRR abs/2103.10620 (2021) - [i59]Fei Feng, Wotao Yin, Alekh Agarwal, Lin F. Yang:
Provably Correct Optimization and Exploration with Non-linear Policies. CoRR abs/2103.11559 (2021) - [i58]Andrea Zanette, Ching-An Cheng, Alekh Agarwal:
Cautiously Optimistic Policy Optimization and Exploration with Linear Function Approximation. CoRR abs/2103.12923 (2021) - [i57]Tengyang Xie, Ching-An Cheng, Nan Jiang, Paul Mineiro, Alekh Agarwal:
Bellman-consistent Pessimism for Offline Reinforcement Learning. CoRR abs/2106.06926 (2021) - [i56]Yonathan Efroni, Dipendra Misra, Akshay Krishnamurthy, Alekh Agarwal, John Langford:
Provable RL with Exogenous Distractors via Multistep Inverse Dynamics. CoRR abs/2110.08847 (2021) - 2020
- [c65]Aditya Modi, Debadeepta Dey, Alekh Agarwal, Adith Swaminathan, Besmira Nushi, Sean Andrist, Eric Horvitz:
Metareasoning in Modular Software Systems: On-the-Fly Configuration Using Reinforcement Learning with Rich Contextual Representations. AAAI 2020: 5207-5215 - [c64]Alekh Agarwal, Sham M. Kakade, Jason D. Lee, Gaurav Mahajan:
Optimality and Approximation with Policy Gradient Methods in Markov Decision Processes. COLT 2020: 64-66 - [c63]Alekh Agarwal, Sham M. Kakade, Lin F. Yang
:
Model-Based Reinforcement Learning with a Generative Model is Minimax Optimal. COLT 2020: 67-83 - [c62]Chen-Yu Wei, Haipeng Luo, Alekh Agarwal:
Taking a hint: How to leverage loss predictors in contextual bandits? COLT 2020: 3583-3634 - [c61]Jordan T. Ash, Chicheng Zhang, Akshay Krishnamurthy, John Langford, Alekh Agarwal:
Deep Batch Active Learning by Diverse, Uncertain Gradient Lower Bounds. ICLR 2020 - [c60]Yao Liu, Adith Swaminathan, Alekh Agarwal, Emma Brunskill:
Provably Good Batch Off-Policy Reinforcement Learning Without Great Exploration. NeurIPS 2020 - [c59]Alekh Agarwal, Mikael Henaff, Sham M. Kakade, Wen Sun:
PC-PG: Policy Cover Directed Exploration for Provable Policy Gradient Learning. NeurIPS 2020 - [c58]Alekh Agarwal, Sham M. Kakade, Akshay Krishnamurthy, Wen Sun:
FLAMBE: Structural Complexity and Representation Learning of Low Rank MDPs. NeurIPS 2020 - [c57]Ching-An Cheng, Andrey Kolobov, Alekh Agarwal:
Policy Improvement via Imitation of Multiple Oracles. NeurIPS 2020 - [c56]Matteo Turchetta, Andrey Kolobov, Shital Shah, Andreas Krause, Alekh Agarwal:
Safe Reinforcement Learning via Curriculum Induction. NeurIPS 2020 - [i55]Chen-Yu Wei, Haipeng Luo, Alekh Agarwal:
Taking a hint: How to leverage loss predictors in contextual bandits? CoRR abs/2003.01922 (2020) - [i54]Alekh Agarwal, John Langford, Chen-Yu Wei:
Federated Residual Learning. CoRR abs/2003.12880 (2020) - [i53]Dilip Arumugam, Debadeepta Dey, Alekh Agarwal, Asli Celikyilmaz, Elnaz Nouri, Bill Dolan:
Reparameterized Variational Divergence Minimization for Stable Imitation. CoRR abs/2006.10810 (2020) - [i52]Alekh Agarwal, Sham M. Kakade, Akshay Krishnamurthy, Wen Sun:
FLAMBE: Structural Complexity and Representation Learning of Low Rank MDPs. CoRR abs/2006.10814 (2020) - [i51]Matteo Turchetta, Andrey Kolobov, Shital Shah, Andreas Krause, Alekh Agarwal:
Safe Reinforcement Learning via Curriculum Induction. CoRR abs/2006.12136 (2020) - [i50]Ziming Li, Julia Kiseleva, Alekh Agarwal, Maarten de Rijke, Ryen W. White:
Optimizing Interactive Systems via Data-Driven Objectives. CoRR abs/2006.12999 (2020) - [i49]Ching-An Cheng, Andrey Kolobov, Alekh Agarwal:
Policy Improvement from Multiple Experts. CoRR abs/2007.00795 (2020) - [i48]Yao Liu, Adith Swaminathan, Alekh Agarwal, Emma Brunskill:
Provably Good Batch Reinforcement Learning Without Great Exploration. CoRR abs/2007.08202 (2020) - [i47]Alekh Agarwal, Mikael Henaff, Sham M. Kakade, Wen Sun:
PC-PG: Policy Cover Directed Exploration for Provable Policy Gradient Learning. CoRR abs/2007.08459 (2020)
2010 – 2019
- 2019
- [j10]Akshay Krishnamurthy, Alekh Agarwal, Tzu-Kuo Huang, Hal Daumé III, John Langford:
Active Learning for Cost-Sensitive Classification. J. Mach. Learn. Res. 20: 65:1-65:50 (2019) - [c55]Wen Sun, Nan Jiang, Akshay Krishnamurthy, Alekh Agarwal, John Langford:
Model-based RL in Contextual Decision Processes: PAC bounds and Exponential Improvements over Model-free Approaches. COLT 2019: 2898-2933 - [c54]Aditya Grover, Jiaming Song, Ashish Kapoor, Kenneth Tran, Alekh Agarwal, Eric Horvitz, Stefano Ermon:
Bias Correction of Learned Generative Models via Likelihood-free Importance Weighting. DGS@ICLR 2019 - [c53]Alekh Agarwal, Miroslav Dudík, Zhiwei Steven Wu:
Fair Regression: Quantitative Definitions and Reduction-Based Algorithms. ICML 2019: 120-129 - [c52]Simon S. Du, Akshay Krishnamurthy, Nan Jiang, Alekh Agarwal, Miroslav Dudík, John Langford:
Provably efficient RL with Rich Observations via Latent State Decoding. ICML 2019: 1665-1674 - [c51]Chicheng Zhang, Alekh Agarwal, Hal Daumé III, John Langford, Sahand Negahban:
Warm-starting Contextual Bandits: Robustly Combining Supervised and Bandit Feedback. ICML 2019: 7335-7344 - [c50]Aditya Grover, Jiaming Song, Ashish Kapoor, Kenneth Tran, Alekh Agarwal, Eric Horvitz, Stefano Ermon:
Bias Correction of Learned Generative Models using Likelihood-Free Importance Weighting. NeurIPS 2019: 11056-11068 - [c49]Yao Liu, Adith Swaminathan, Alekh Agarwal, Emma Brunskill:
Off-Policy Policy Gradient with Stationary Distribution Correction. UAI 2019: 1180-1190 - [i46]Chicheng Zhang, Alekh Agarwal, Hal Daumé III, John Langford, Sahand N. Negahban:
Warm-starting Contextual Bandits: Robustly Combining Supervised and Bandit Feedback. CoRR abs/1901.00301 (2019) - [i45]Simon S. Du, Akshay Krishnamurthy, Nan Jiang, Alekh Agarwal, Miroslav Dudík, John Langford:
Provably efficient RL with Rich Observations via Latent State Decoding. CoRR abs/1901.09018 (2019) - [i44]Yao Liu, Adith Swaminathan, Alekh Agarwal, Emma Brunskill:
Off-Policy Policy Gradient with State Distribution Correction. CoRR abs/1904.08473 (2019) - [i43]Aditya Modi, Debadeepta Dey, Alekh Agarwal, Adith Swaminathan, Besmira Nushi, Sean Andrist, Eric Horvitz:
Metareasoning in Modular Software Systems: On-the-Fly Configuration using Reinforcement Learning with Rich Contextual Representations. CoRR abs/1905.05179 (2019) - [i42]Alekh Agarwal, Miroslav Dudík, Zhiwei Steven Wu:
Fair Regression: Quantitative Definitions and Reduction-based Algorithms. CoRR abs/1905.12843 (2019) - [i41]Jordan T. Ash, Chicheng Zhang, Akshay Krishnamurthy, John Langford, Alekh Agarwal:
Deep Batch Active Learning by Diverse, Uncertain Gradient Lower Bounds. CoRR abs/1906.03671 (2019) - [i40]Alekh Agarwal, Sham M. Kakade, Lin F. Yang:
On the Optimality of Sparse Model-Based Planning for Markov Decision Processes. CoRR abs/1906.03804 (2019) - [i39]Aditya Grover, Jiaming Song, Alekh Agarwal, Kenneth Tran, Ashish Kapoor, Eric Horvitz, Stefano Ermon:
Bias Correction of Learned Generative Models using Likelihood-Free Importance Weighting. CoRR abs/1906.09531 (2019) - [i38]Alekh Agarwal, Sham M. Kakade, Jason D. Lee, Gaurav Mahajan:
Optimality and Approximation with Policy Gradient Methods in Markov Decision Processes. CoRR abs/1908.00261 (2019) - 2018
- [c48]Haipeng Luo, Chen-Yu Wei, Alekh Agarwal, John Langford:
Efficient Contextual Bandits in Non-stationary Worlds. COLT 2018: 1739-1776 - [c47]Nan Jiang, Alekh Agarwal:
Open Problem: The Dependence of Sample Complexity Lower Bounds on Planning Horizon. COLT 2018: 3395-3398 - [c46]Alekh Agarwal, Alina Beygelzimer, Miroslav Dudík, John Langford, Hanna M. Wallach:
A Reductions Approach to Fair Classification. ICML 2018: 60-69 - [c45]Dylan J. Foster, Alekh Agarwal, Miroslav Dudík, Haipeng Luo, Robert E. Schapire:
Practical Contextual Bandits with Regression Oracles. ICML 2018: 1534-1543 - [c44]Hoang Minh Le, Nan Jiang, Alekh Agarwal, Miroslav Dudík, Yisong Yue, Hal Daumé III:
Hierarchical Imitation and Reinforcement Learning. ICML 2018: 2923-2932 - [c43]Christoph Dann, Nan Jiang, Akshay Krishnamurthy, Alekh Agarwal, John Langford, Robert E. Schapire:
On Oracle-Efficient PAC RL with Rich Observations. NeurIPS 2018: 1429-1439 - [i37]Alberto Bietti, Alekh Agarwal, John Langford:
Practical Evaluation and Optimization of Contextual Bandit Algorithms. CoRR abs/1802.04064 (2018) - [i36]Hoang Minh Le, Nan Jiang, Alekh Agarwal, Miroslav Dudík, Yisong Yue, Hal Daumé III:
Hierarchical Imitation and Reinforcement Learning. CoRR abs/1803.00590 (2018) - [i35]Christoph Dann, Nan Jiang, Akshay Krishnamurthy, Alekh Agarwal, John Langford, Robert E. Schapire:
On Polynomial Time PAC Reinforcement Learning with Rich Observations. CoRR abs/1803.00606 (2018) - [i34]Dylan J. Foster, Alekh Agarwal, Miroslav Dudík, Haipeng Luo, Robert E. Schapire:
Practical Contextual Bandits with Regression Oracles. CoRR abs/1803.01088 (2018) - [i33]Alekh Agarwal, Alina Beygelzimer, Miroslav Dudík, John Langford, Hanna M. Wallach:
A Reductions Approach to Fair Classification. CoRR abs/1803.02453 (2018) - [i32]Wen Sun, Nan Jiang, Akshay Krishnamurthy, Alekh Agarwal, John Langford:
Model-Based Reinforcement Learning in Contextual Decision Processes. CoRR abs/1811.08540 (2018) - 2017
- [j9]Alekh Agarwal
, Animashree Anandkumar, Praneeth Netrapalli:
A Clustering Approach to Learning Sparsely Used Overcomplete Dictionaries. IEEE Trans. Inf. Theory 63(1): 575-592 (2017) - [c42]Alekh Agarwal, Akshay Krishnamurthy, John Langford, Haipeng Luo, Robert E. Schapire:
Open Problem: First-Order Regret Bounds for Contextual Bandits. COLT 2017: 4-7 - [c41]Alekh Agarwal, Haipeng Luo, Behnam Neyshabur, Robert E. Schapire:
Corralling a Band of Bandit Algorithms. COLT 2017: 12-38 - [c40]Nan Jiang, Akshay Krishnamurthy, Alekh Agarwal, John Langford, Robert E. Schapire:
Contextual Decision Processes with low Bellman rank are PAC-Learnable. ICML 2017: 1704-1713 - [c39]Akshay Krishnamurthy, Alekh Agarwal, Tzu-Kuo Huang, Hal Daumé III, John Langford:
Active Learning for Cost-Sensitive Classification. ICML 2017: 1915-1924 - [c38]Yu-Xiang Wang, Alekh Agarwal, Miroslav Dudík:
Optimal and Adaptive Off-policy Evaluation in Contextual Bandits. ICML 2017: 3589-3597 - [c37]Adith Swaminathan, Akshay Krishnamurthy, Alekh Agarwal, Miroslav Dudík, John Langford, Damien Jose, Imed Zitouni:
Off-policy evaluation for slate recommendation. NIPS 2017: 3632-3642 - [i31]Akshay Krishnamurthy, Alekh Agarwal, Tzu-Kuo Huang, Hal Daumé III, John Langford:
Active Learning for Cost-Sensitive Classification. CoRR abs/1703.01014 (2017) - [i30]Haipeng Luo, Alekh Agarwal, John Langford:
Efficient Contextual Bandits in Non-stationary Worlds. CoRR abs/1708.01799 (2017) - 2016
- [j8]Alekh Agarwal, Animashree Anandkumar, Prateek Jain, Praneeth Netrapalli:
Learning Sparsely Used Overcomplete Dictionaries via Alternating Minimization. SIAM J. Optim. 26(4): 2775-2799 (2016) - [c36]Haipeng Luo, Alekh Agarwal, Nicolò Cesa-Bianchi, John Langford:
Efficient Second Order Online Learning by Sketching. NIPS 2016: 902-910 - [c35]Akshay Krishnamurthy, Alekh Agarwal, John Langford:
PAC Reinforcement Learning with Rich Observations. NIPS 2016: 1840-1848 - [c34]Akshay Krishnamurthy, Alekh Agarwal, Miroslav Dudík:
Contextual semibandits via supervised learning oracles. NIPS 2016: 2388-2396 - [i29]Haipeng Luo, Alekh Agarwal, Nicolò Cesa-Bianchi, John Langford:
Efficient Second Order Online Learning via Sketching. CoRR abs/1602.02202 (2016) - [i28]Akshay Krishnamurthy, Alekh Agarwal, John Langford:
Contextual-MDPs for PAC-Reinforcement Learning with Rich Observations. CoRR abs/1602.02722 (2016) - [i27]David Abel, Alekh Agarwal, Fernando Diaz, Akshay Krishnamurthy, Robert E. Schapire:
Exploratory Gradient Boosting for Reinforcement Learning in Complex Domains. CoRR abs/1603.04119 (2016) - [i26]Adith Swaminathan, Akshay Krishnamurthy, Alekh Agarwal, Miroslav Dudík, John Langford, Damien Jose, Imed Zitouni:
Off-policy evaluation for slate recommendation. CoRR abs/1605.04812 (2016) - [i25]Alekh Agarwal, Sarah Bird, Markus Cozowicz, Luong Hoang, John Langford, Stephen Lee, Jiaji Li, I. Dan Melamed, Gal Oshri, Oswaldo Ribas, Siddhartha Sen, Alex Slivkins:
A Multiworld Testing Decision Service. CoRR abs/1606.03966 (2016) - [i24]Nan Jiang, Akshay Krishnamurthy, Alekh Agarwal, John Langford, Robert E. Schapire:
Contextual Decision Processes with Low Bellman Rank are PAC-Learnable. CoRR abs/1610.09512 (2016) - [i23]Yu-Xiang Wang, Alekh Agarwal, Miroslav Dudík:
Optimal and Adaptive Off-policy Evaluation in Contextual Bandits. CoRR abs/1612.01205 (2016) - [i22]Alekh Agarwal, Haipeng Luo, Behnam Neyshabur, Robert E. Schapire:
Corralling a Band of Bandit Algorithms. CoRR abs/1612.06246 (2016) - 2015
- [c33]Alekh Agarwal, Léon Bottou:
A Lower Bound for the Optimization of Finite Sums. ICML 2015: 78-86 - [c32]Kai-Wei Chang, Akshay Krishnamurthy, Alekh Agarwal, Hal Daumé III, John Langford:
Learning to Search Better than Your Teacher. ICML 2015: 2058-2066 - [c31]Tzu-Kuo Huang, Alekh Agarwal, Daniel J. Hsu, John Langford, Robert E. Schapire:
Efficient and Parsimonious Agnostic Active Learning. NIPS 2015: 2755-2763 - [c30]Vasilis Syrgkanis, Alekh Agarwal, Haipeng Luo, Robert E. Schapire:
Fast Convergence of Regularized Learning in Games. NIPS 2015: 2989-2997 - [i21]Kai-Wei Chang, Akshay Krishnamurthy, Alekh Agarwal, Hal Daumé III, John Langford:
Learning to Search Better Than Your Teacher. CoRR abs/1502.02206 (2015) - [i20]Akshay Krishnamurthy, Alekh Agarwal, Miroslav Dudík:
Efficient Contextual Semi-Bandit Learning. CoRR abs/1502.05890 (2015) - [i19]Tzu-Kuo Huang, Alekh Agarwal, Daniel J. Hsu, John Langford, Robert E. Schapire:
Efficient and Parsimonious Agnostic Active Learning. CoRR abs/1506.08669 (2015) - [i18]Vasilis Syrgkanis, Alekh Agarwal, Haipeng Luo, Robert E. Schapire:
Fast Convergence of Regularized Learning in Games. CoRR abs/1507.00407 (2015) - 2014
- [j7]Alekh Agarwal, Olivier Chapelle, Miroslav Dudík, John Langford:
A reliable effective terascale linear learning system. J. Mach. Learn. Res. 15(1): 1111-1133 (2014) - [c29]Alekh Agarwal, Sahand N. Negahban, Martin J. Wainwright
:
Stochastic optimization and sparse statistical recovery: An optimal algorithm for high dimensions. CISS 2014: 1-2 - [c28]Alekh Agarwal, Animashree Anandkumar, Prateek Jain, Praneeth Netrapalli, Rashish Tandon:
Learning Sparsely Used Overcomplete Dictionaries. COLT 2014: 123-137 - [c27]Alekh Agarwal, Ashwinkumar Badanidiyuru, Miroslav Dudík, Robert E. Schapire, Aleksandrs Slivkins:
Robust Multi-objective Learning with Mentor Feedback. COLT 2014: 726-741 - [c26]Alekh Agarwal, Sham M. Kakade, Nikos Karampatziakis, Le Song, Gregory Valiant:
Least Squares Revisited: Scalable Approaches for Multi-class Prediction. ICML 2014: 541-549 - [c25]Alekh Agarwal, Daniel J. Hsu, Satyen Kale, John Langford, Lihong Li, Robert E. Schapire:
Taming the Monster: A Fast and Simple Algorithm for Contextual Bandits. ICML 2014: 1638-1646 - [c24]Alekh Agarwal, Alina Beygelzimer, Daniel J. Hsu, John Langford, Matus Telgarsky:
Scalable Non-linear Learning with Adaptive Polynomial Expansions. NIPS 2014: 2051-2059 - [i17]Alekh Agarwal, Daniel J. Hsu, Satyen Kale, John Langford, Lihong Li, Robert E. Schapire:
Taming the Monster: A Fast and Simple Algorithm for Contextual Bandits. CoRR abs/1402.0555 (2014) - [i16]Alekh Agarwal, Alina Beygelzimer, Daniel J. Hsu, John Langford, Matus Telgarsky:
Scalable Nonlinear Learning with Adaptive Polynomial Expansions. CoRR abs/1410.0440 (2014) - 2013
- [j6]Alekh Agarwal, Dean P. Foster, Daniel J. Hsu, Sham M. Kakade, Alexander Rakhlin:
Stochastic Convex Optimization with Bandit Feedback. SIAM J. Optim. 23(1): 213-240 (2013) - [j5]Alekh Agarwal, John C. Duchi:
The Generalization Ability of Online Algorithms for Dependent Data. IEEE Trans. Inf. Theory 59(1): 573-587 (2013) - [c23]Alekh Agarwal:
Selective sampling algorithms for cost-sensitive multiclass prediction. ICML (3) 2013: 1220-1228 - [i15]Alekh Agarwal, Animashree Anandkumar, Praneeth Netrapalli:
Exact Recovery of Sparsely Used Overcomplete Dictionaries. CoRR abs/1309.1952 (2013) - [i14]Alekh Agarwal, Sham M. Kakade, Nikos Karampatziakis, Le Song, Gregory Valiant:
Least Squares Revisited: Scalable Approaches for Multi-class Prediction. CoRR abs/1310.1949 (2013) - [i13]Alekh Agarwal, Animashree Anandkumar, Prateek Jain, Praneeth Netrapalli, Rashish Tandon:
Learning Sparsely Used Overcomplete Dictionaries via Alternating Minimization. CoRR abs/1310.7991 (2013) - [i12]Alekh Agarwal, Léon Bottou, Miroslav Dudík, John Langford:
Para-active learning. CoRR abs/1310.8243 (2013) - 2012
- [b1]Alekh Agarwal:
Computational Trade-offs in Statistical Learning. University of California, Berkeley, USA, 2012 - [j4]John C. Duchi, Alekh Agarwal, Mikael Johansson, Michael I. Jordan
:
Ergodic Mirror Descent. SIAM J. Optim. 22(4): 1549-1578 (2012) - [j3]John C. Duchi, Alekh Agarwal, Martin J. Wainwright
:
Dual Averaging for Distributed Optimization: Convergence Analysis and Network Scaling. IEEE Trans. Autom. Control. 57(3): 592-606 (2012) - [j2]Alekh Agarwal, Peter L. Bartlett
, Pradeep Ravikumar, Martin J. Wainwright
:
Information-Theoretic Lower Bounds on the Oracle Complexity of Stochastic Convex Optimization. IEEE Trans. Inf. Theory 58(5): 3235-3249 (2012) - [c22]John C. Duchi, Alekh Agarwal, Martin J. Wainwright
:
Dual averaging for distributed optimization. Allerton Conference 2012: 1564-1565 - [c21]Alekh Agarwal, John C. Duchi:
Distributed delayed stochastic optimization. CDC 2012: 5451-5452 - [c20]Alekh Agarwal, Sahand N. Negahban, Martin J. Wainwright:
Stochastic optimization and sparse statistical recovery: Optimal algorithms for high dimensions. NIPS 2012: 1547-1555 - [c19]Alekh Agarwal, Sahand N. Negahban, Martin J. Wainwright
:
FASt global convergence of gradient methods for solving regularized M-estimation. SSP 2012: 409-412 - [c18]Alekh Agarwal, Miroslav Dudík, Satyen Kale, John Langford, Robert E. Schapire:
Contextual Bandit Learning with Predictable Rewards. AISTATS 2012: 19-26 - [i11]Alekh Agarwal, Miroslav Dudík, Satyen Kale, John Langford, Robert E. Schapire:
Contextual Bandit Learning with Predictable Rewards. CoRR abs/1202.1334 (2012) - [i10]Alekh Agarwal, Sahand N. Negahban, Martin J. Wainwright:
Stochastic optimization and sparse statistical recovery: An optimal algorithm for high dimensions. CoRR abs/1207.4421 (2012) - [i9]Alekh Agarwal, Peter L. Bartlett, John C. Duchi:
Oracle inequalities for computationally adaptive model selection. CoRR abs/1208.0129 (2012) - 2011
- [c17]John C. Duchi, Alekh Agarwal, Mikael Johansson, Michael I. Jordan
:
Ergodic mirror descent. Allerton 2011: 701-706 - [c16]Alekh Agarwal, Sahand N. Negahban, Martin J. Wainwright:
Noisy matrix decomposition via convex relaxation: Optimal rates in high dimensions. ICML 2011: 1129-1136 - [c15]Alekh Agarwal, John C. Duchi:
Distributed Delayed Stochastic Optimization. NIPS 2011: 873-881 - [c14]Alekh Agarwal, Dean P. Foster, Daniel J. Hsu, Sham M. Kakade, Alexander Rakhlin:
Stochastic convex optimization with bandit feedback. NIPS 2011: 1035-1043 - [c13]Afshin Rostamizadeh, Alekh Agarwal, Peter L. Bartlett:
Learning with Missing Features. UAI 2011: 635-642 - [c12]Alekh Agarwal, John C. Duchi, Peter L. Bartlett, Clément Levrard:
Oracle inequalities for computationally budgeted model selection. COLT 2011: 69-86 - [i8]Alekh Agarwal, Sahand N. Negahban, Martin J. Wainwright:
Noisy matrix decomposition via convex relaxation: Optimal rates in high dimensions. CoRR abs/1102.4807 (2011) - [i7]Afshin Rostamizadeh, Alekh Agarwal, Peter L. Bartlett:
Online and Batch Learning Algorithms for Data with Missing Features. CoRR abs/1104.0729 (2011) - [i6]Alekh Agarwal, Sahand N. Negahban, Martin J. Wainwright:
Fast global convergence of gradient methods for high-dimensional statistical recovery. CoRR abs/1104.4824 (2011) - [i5]Alekh Agarwal, Dean P. Foster, Daniel J. Hsu, Sham M. Kakade, Alexander Rakhlin:
Stochastic convex optimization with bandit feedback. CoRR abs/1107.1744 (2011) - [i4]Alekh Agarwal, John C. Duchi:
The Generalization Ability of Online Algorithms for Dependent Data. CoRR abs/1110.2529 (2011) - [i3]Alekh Agarwal, Olivier Chapelle, Miroslav Dudík, John Langford:
A Reliable Effective Terascale Linear Learning System. CoRR abs/1110.4198 (2011) - 2010
- [j1]Pradeep Ravikumar, Alekh Agarwal, Martin J. Wainwright:
Message-passing for Graph-structured Linear Programs: Proximal Methods and Rounding Schemes. J. Mach. Learn. Res. 11: 1043-1080 (2010) - [c11]Alekh Agarwal, Ofer Dekel, Lin Xiao:
Optimal Algorithms for Online Convex Optimization with Multi-Point Bandit Feedback. COLT 2010: 28-40 - [c10]Alekh Agarwal, Sahand N. Negahban, Martin J. Wainwright:
Fast global convergence rates of gradient methods for high-dimensional statistical recovery. NIPS 2010: 37-45 - [c9]John C. Duchi, Alekh Agarwal, Martin J. Wainwright:
Distributed Dual Averaging In Networks. NIPS 2010: 550-558 - [c8]Alekh Agarwal, Peter L. Bartlett, Max Dama:
Optimal Allocation Strategies for the Dark Pool Problem. AISTATS 2010: 9-16 - [i2]Alekh Agarwal, Peter L. Bartlett, Pradeep Ravikumar, Martin J. Wainwright:
Information-theoretic lower bounds on the oracle complexity of stochastic convex optimization. CoRR abs/1009.0571 (2010)
2000 – 2009
- 2009
- [c7]Jacob D. Abernethy, Alekh Agarwal, Peter L. Bartlett, Alexander Rakhlin:
A Stochastic View of Optimal Regret through Minimax Duality. COLT 2009 - [c6]Alekh Agarwal, Peter L. Bartlett, Pradeep Ravikumar, Martin J. Wainwright:
Information-theoretic lower bounds on the oracle complexity of convex optimization. NIPS 2009: 1-9 - [i1]Jacob D. Abernethy, Alekh Agarwal, Peter L. Bartlett, Alexander Rakhlin:
A Stochastic View of Optimal Regret through Minimax Duality. CoRR abs/0903.5328 (2009) - 2008
- [c5]Pradeep Ravikumar, Alekh Agarwal, Martin J. Wainwright:
Message-passing for graph-structured linear programs: proximal projections, convergence and rounding schemes. ICML 2008: 800-807 - 2007
- [c4]Alekh Agarwal, Soumen Chakrabarti:
Learning random walks to rank nodes in graphs. ICML 2007: 9-16 - [c3]Fabian H. Sinz, Olivier Chapelle, Alekh Agarwal, Bernhard Schölkopf:
An Analysis of Inference with the Universum. NIPS 2007: 1369-1376 - 2006
- [c2]Alekh Agarwal, Soumen Chakrabarti, Sunny Aggarwal:
Learning to rank networked entities. KDD 2006: 14-23 - [c1]Soumen Chakrabarti, Alekh Agarwal:
Learning Parameters in Entity Relationship Graphs from Ranking Preferences. PKDD 2006: 91-102
Coauthor Index

manage site settings
To protect your privacy, all features that rely on external API calls from your browser are turned off by default. You need to opt-in for them to become active. All settings here will be stored as cookies with your web browser. For more information see our F.A.Q.
Unpaywalled article links
Add open access links from to the list of external document links (if available).
Privacy notice: By enabling the option above, your browser will contact the API of unpaywall.org to load hyperlinks to open access articles. Although we do not have any reason to believe that your call will be tracked, we do not have any control over how the remote server uses your data. So please proceed with care and consider checking the Unpaywall privacy policy.
Archived links via Wayback Machine
For web page which are no longer available, try to retrieve content from the of the Internet Archive (if available).
Privacy notice: By enabling the option above, your browser will contact the API of archive.org to check for archived content of web pages that are no longer available. Although we do not have any reason to believe that your call will be tracked, we do not have any control over how the remote server uses your data. So please proceed with care and consider checking the Internet Archive privacy policy.
Reference lists
Add a list of references from ,
, and
to record detail pages.
load references from crossref.org and opencitations.net
Privacy notice: By enabling the option above, your browser will contact the APIs of crossref.org, opencitations.net, and semanticscholar.org to load article reference information. Although we do not have any reason to believe that your call will be tracked, we do not have any control over how the remote server uses your data. So please proceed with care and consider checking the Crossref privacy policy and the OpenCitations privacy policy, as well as the AI2 Privacy Policy covering Semantic Scholar.
Citation data
Add a list of citing articles from and
to record detail pages.
load citations from opencitations.net
Privacy notice: By enabling the option above, your browser will contact the API of opencitations.net and semanticscholar.org to load citation information. Although we do not have any reason to believe that your call will be tracked, we do not have any control over how the remote server uses your data. So please proceed with care and consider checking the OpenCitations privacy policy as well as the AI2 Privacy Policy covering Semantic Scholar.
OpenAlex data
Load additional information about publications from .
Privacy notice: By enabling the option above, your browser will contact the API of openalex.org to load additional information. Although we do not have any reason to believe that your call will be tracked, we do not have any control over how the remote server uses your data. So please proceed with care and consider checking the information given by OpenAlex.
last updated on 2025-03-13 20:22 CET by the dblp team
all metadata released as open data under CC0 1.0 license
see also: Terms of Use | Privacy Policy | Imprint