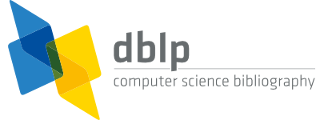


default search action
Gang Niu 0001
Person information
- affiliation: RIKEN, Japan
- affiliation (PhD 2013): Tokyo Institute of Technology, Department of Computer Science, Japan
- affiliation (former): Nanjing University, State Key Laboratory for Novel Software Technology, Nanjing, China
Other persons with the same name
- Gang Niu 0002 — City University of Hong Kong, Center for Prognostics and System Health Management, Hong Kong (and 1 more)
- Gang Niu 0003 — First Affiliated Hospital of Xi'an Jiaotong University, Department of Radiology, Xi'an, China
- Gang Niu 0004
— Tongji University, Institute of Rail Transit, Shanghai, China
- Gang Niu 0005 — Zhengzhou Huali Information Technology Co., Ltd., China
- Gang Niu 0006
— Xi'an Jiaotong University, International Center for Dielectric Research, China (and 1 more)
- Gang Niu 0007 — Sun Yat-sen University, First Affiliated Hospital, Department of Obstetrics and Gynecology, Guangzhou, China
- Gang Niu 0008 — Phil Rivers Technology Ltd, Beijing, China
Refine list

refinements active!
zoomed in on ?? of ?? records
view refined list in
export refined list as
2020 – today
- 2024
- [j28]Tingting Zhao, Guixi Li, Tuo Zhao, Yarui Chen
, Ning Xie, Gang Niu, Masashi Sugiyama
:
Learning explainable task-relevant state representation for model-free deep reinforcement learning. Neural Networks 180: 106741 (2024) - [j27]Jiaqi Lv
, Biao Liu
, Lei Feng
, Ning Xu
, Miao Xu
, Bo An
, Gang Niu
, Xin Geng
, Masashi Sugiyama
:
On the Robustness of Average Losses for Partial-Label Learning. IEEE Trans. Pattern Anal. Mach. Intell. 46(5): 2569-2583 (2024) - [j26]Haobo Wang
, Ruixuan Xiao
, Yixuan Li
, Lei Feng
, Gang Niu
, Gang Chen
, Junbo Zhao
:
PiCO+: Contrastive Label Disambiguation for Robust Partial Label Learning. IEEE Trans. Pattern Anal. Mach. Intell. 46(5): 3183-3198 (2024) - [j25]Yinghua Gao
, Dongxian Wu, Jingfeng Zhang, Guanhao Gan, Shu-Tao Xia
, Gang Niu
, Masashi Sugiyama
:
On the Effectiveness of Adversarial Training Against Backdoor Attacks. IEEE Trans. Neural Networks Learn. Syst. 35(10): 14878-14888 (2024) - [c102]Jie Xu, Yazhou Ren, Xiaolong Wang, Lei Feng, Zheng Zhang, Gang Niu, Xiaofeng Zhu:
Investigating and Mitigating the Side Effects of Noisy Views for Self-Supervised Clustering Algorithms in Practical Multi-View Scenarios. CVPR 2024: 22957-22966 - [c101]Jialiang Tang, Shuo Chen, Gang Niu, Hongyuan Zhu, Joey Tianyi Zhou, Chen Gong, Masashi Sugiyama:
Direct Distillation Between Different Domains. ECCV (80) 2024: 154-172 - [c100]Shuo Chen, Gang Niu, Chen Gong, Okan Koc, Jian Yang, Masashi Sugiyama:
Robust Similarity Learning with Difference Alignment Regularization. ICLR 2024 - [c99]Abudukelimu Wuerkaixi, Sen Cui, Jingfeng Zhang, Kunda Yan, Bo Han, Gang Niu, Lei Fang, Changshui Zhang, Masashi Sugiyama:
Accurate Forgetting for Heterogeneous Federated Continual Learning. ICLR 2024 - [c98]Ziqing Fan, Shengchao Hu, Jiangchao Yao, Gang Niu, Ya Zhang, Masashi Sugiyama, Yanfeng Wang:
Locally Estimated Global Perturbations are Better than Local Perturbations for Federated Sharpness-aware Minimization. ICML 2024 - [c97]Wei Wang, Takashi Ishida, Yu-Jie Zhang, Gang Niu, Masashi Sugiyama:
Learning with Complementary Labels Revisited: The Selected-Completely-at-Random Setting Is More Practical. ICML 2024 - [c96]Ming-Kun Xie, Jiahao Xiao, Pei Peng, Gang Niu, Masashi Sugiyama, Sheng-Jun Huang:
Counterfactual Reasoning for Multi-Label Image Classification via Patching-Based Training. ICML 2024 - [c95]Kunda Yan, Sen Cui, Abudukelimu Wuerkaixi, Jingfeng Zhang, Bo Han, Gang Niu, Masashi Sugiyama, Changshui Zhang:
Balancing Similarity and Complementarity for Federated Learning. ICML 2024 - [c94]Zhen-Yu Zhang, Siwei Han, Huaxiu Yao, Gang Niu, Masashi Sugiyama:
Generating Chain-of-Thoughts with a Pairwise-Comparison Approach to Searching for the Most Promising Intermediate Thought. ICML 2024 - [i108]Jialiang Tang, Shuo Chen, Gang Niu, Hongyuan Zhu, Joey Tianyi Zhou, Chen Gong, Masashi Sugiyama:
Direct Distillation between Different Domains. CoRR abs/2401.06826 (2024) - [i107]Zhen-Yu Zhang, Siwei Han, Huaxiu Yao, Gang Niu, Masashi Sugiyama:
Generating Chain-of-Thoughts with a Direct Pairwise-Comparison Approach to Searching for the Most Promising Intermediate Thought. CoRR abs/2402.06918 (2024) - [i106]Ming-Kun Xie, Jiahao Xiao, Pei Peng, Gang Niu, Masashi Sugiyama, Sheng-Jun Huang:
Counterfactual Reasoning for Multi-Label Image Classification via Patching-Based Training. CoRR abs/2404.06287 (2024) - [i105]Kunda Yan, Sen Cui, Abudukelimu Wuerkaixi, Jingfeng Zhang, Bo Han, Gang Niu, Masashi Sugiyama, Changshui Zhang:
Balancing Similarity and Complementarity for Federated Learning. CoRR abs/2405.09892 (2024) - [i104]Ziqing Fan, Shengchao Hu, Jiangchao Yao, Gang Niu, Ya Zhang, Masashi Sugiyama, Yanfeng Wang:
Locally Estimated Global Perturbations are Better than Local Perturbations for Federated Sharpness-aware Minimization. CoRR abs/2405.18890 (2024) - [i103]Jianing Zhu, Bo Han, Jiangchao Yao, Jianliang Xu, Gang Niu, Masashi Sugiyama:
Decoupling the Class Label and the Target Concept in Machine Unlearning. CoRR abs/2406.08288 (2024) - [i102]Jiahao Xiao, Ming-Kun Xie, Heng-Bo Fan, Gang Niu, Masashi Sugiyama, Sheng-Jun Huang:
Dual-Decoupling Learning and Metric-Adaptive Thresholding for Semi-Supervised Multi-Label Learning. CoRR abs/2407.18624 (2024) - [i101]Zhen-Yu Zhang, Jiandong Zhang, Huaxiu Yao, Gang Niu, Masashi Sugiyama:
On Unsupervised Prompt Learning for Classification with Black-box Language Models. CoRR abs/2410.03124 (2024) - 2023
- [j24]Shuo Chen
, Chen Gong, Xiang Li, Jian Yang, Gang Niu, Masashi Sugiyama:
Boundary-restricted metric learning. Mach. Learn. 112(12): 4723-4762 (2023) - [j23]Tingting Zhao, S. Wu, G. Li, Y. Chen, Gang Niu, Masashi Sugiyama:
Learning Intention-Aware Policies in Deep Reinforcement Learning. Neural Comput. 35(10): 1657-1677 (2023) - [j22]Tingting Zhao, Ying Wang, Wei Sun, Yarui Chen, Gang Niu, Masashi Sugiyama:
Representation learning for continuous action spaces is beneficial for efficient policy learning. Neural Networks 159: 137-152 (2023) - [j21]Chen Gong
, Yongliang Ding, Bo Han
, Gang Niu
, Jian Yang
, Jane You
, Dacheng Tao
, Masashi Sugiyama
:
Class-Wise Denoising for Robust Learning Under Label Noise. IEEE Trans. Pattern Anal. Mach. Intell. 45(3): 2835-2848 (2023) - [j20]Shuo Yang
, Songhua Wu
, Erkun Yang
, Bo Han
, Yang Liu
, Min Xu
, Gang Niu
, Tongliang Liu
:
A Parametrical Model for Instance-Dependent Label Noise. IEEE Trans. Pattern Anal. Mach. Intell. 45(12): 14055-14068 (2023) - [j19]Lei Feng
, Senlin Shu, Yuzhou Cao, Lue Tao, Hongxin Wei
, Tao Xiang
, Bo An
, Gang Niu
:
Multiple-Instance Learning From Unlabeled Bags With Pairwise Similarity. IEEE Trans. Knowl. Data Eng. 35(11): 11599-11609 (2023) - [c93]Shiyu Xia, Jiaqi Lv, Ning Xu, Gang Niu, Xin Geng:
Towards Effective Visual Representations for Partial-Label Learning. CVPR 2023: 15589-15598 - [c92]Penghui Yang, Ming-Kun Xie, Chen-Chen Zong, Lei Feng, Gang Niu, Masashi Sugiyama, Sheng-Jun Huang:
Multi-Label Knowledge Distillation. ICCV 2023: 17225-17234 - [c91]Jialiang Tang, Shuo Chen, Gang Niu, Masashi Sugiyama, Chen Gong:
Distribution Shift Matters for Knowledge Distillation with Webly Collected Images. ICCV 2023: 17424-17434 - [c90]Takashi Ishida, Ikko Yamane, Nontawat Charoenphakdee, Gang Niu, Masashi Sugiyama:
Is the Performance of My Deep Network Too Good to Be True? A Direct Approach to Estimating the Bayes Error in Binary Classification. ICLR 2023 - [c89]Ruijiang Dong, Feng Liu, Haoang Chi, Tongliang Liu, Mingming Gong, Gang Niu, Masashi Sugiyama, Bo Han:
Diversity-enhancing Generative Network for Few-shot Hypothesis Adaptation. ICML 2023: 8260-8275 - [c88]Zixi Wei, Lei Feng, Bo Han, Tongliang Liu, Gang Niu, Xiaofeng Zhu, Heng Tao Shen:
A Universal Unbiased Method for Classification from Aggregate Observations. ICML 2023: 36804-36820 - [c87]Hongxin Wei, Huiping Zhuang, Renchunzi Xie, Lei Feng, Gang Niu, Bo An, Yixuan Li:
Mitigating Memorization of Noisy Labels by Clipping the Model Prediction. ICML 2023: 36868-36886 - [c86]Tongtong Fang, Nan Lu, Gang Niu, Masashi Sugiyama:
Generalizing Importance Weighting to A Universal Solver for Distribution Shift Problems. NeurIPS 2023 - [c85]Wei Wang, Lei Feng, Yuchen Jiang, Gang Niu, Min-Ling Zhang, Masashi Sugiyama:
Binary Classification with Confidence Difference. NeurIPS 2023 - [c84]Ming-Kun Xie, Jiahao Xiao, Hao-Zhe Liu, Gang Niu, Masashi Sugiyama, Sheng-Jun Huang:
Class-Distribution-Aware Pseudo-Labeling for Semi-Supervised Multi-Label Learning. NeurIPS 2023 - [c83]Jie Xu, Shuo Chen, Yazhou Ren, Xiaoshuang Shi, Hengtao Shen, Gang Niu, Xiaofeng Zhu:
Self-Weighted Contrastive Learning among Multiple Views for Mitigating Representation Degeneration. NeurIPS 2023 - [c82]Jianing Zhu, Yu Geng, Jiangchao Yao, Tongliang Liu, Gang Niu, Masashi Sugiyama, Bo Han:
Diversified Outlier Exposure for Out-of-Distribution Detection via Informative Extrapolation. NeurIPS 2023 - [i100]Jiaheng Wei, Zhaowei Zhu, Gang Niu, Tongliang Liu, Sijia Liu, Masashi Sugiyama, Yang Liu:
Fairness Improves Learning from Noisily Labeled Long-Tailed Data. CoRR abs/2303.12291 (2023) - [i99]Jie Xu, Gang Niu, Xiaolong Wang, Yazhou Ren, Lei Feng, Xiaoshuang Shi, Heng Tao Shen, Xiaofeng Zhu:
Investigating and Mitigating the Side Effects of Noisy Views in Multi-view Clustering in Practical Scenarios. CoRR abs/2303.17245 (2023) - [i98]Jingfeng Zhang, Bo Song, Bo Han, Lei Liu, Gang Niu, Masashi Sugiyama:
Assessing Vulnerabilities of Adversarial Learning Algorithm through Poisoning Attacks. CoRR abs/2305.00399 (2023) - [i97]Ming-Kun Xie, Jiahao Xiao, Hao-Zhe Liu, Gang Niu, Masashi Sugiyama, Sheng-Jun Huang:
Class-Distribution-Aware Pseudo Labeling for Semi-Supervised Multi-Label Learning. CoRR abs/2305.02795 (2023) - [i96]Shiyu Xia, Jiaqi Lv, Ning Xu, Gang Niu, Xin Geng:
Towards Effective Visual Representations for Partial-Label Learning. CoRR abs/2305.06080 (2023) - [i95]Wei-I Lin, Gang Niu, Hsuan-Tien Lin, Masashi Sugiyama:
Enhancing Label Sharing Efficiency in Complementary-Label Learning with Label Augmentation. CoRR abs/2305.08344 (2023) - [i94]Tongtong Fang
, Nan Lu, Gang Niu, Masashi Sugiyama:
Generalizing Importance Weighting to A Universal Solver for Distribution Shift Problems. CoRR abs/2305.14690 (2023) - [i93]Yuhao Wu, Xiaobo Xia, Jun Yu, Bo Han, Gang Niu, Masashi Sugiyama, Tongliang Liu:
Making Binary Classification from Multiple Unlabeled Datasets Almost Free of Supervision. CoRR abs/2306.07036 (2023) - [i92]Zixi Wei, Lei Feng, Bo Han, Tongliang Liu, Gang Niu, Xiaofeng Zhu, Heng Tao Shen:
A Universal Unbiased Method for Classification from Aggregate Observations. CoRR abs/2306.11343 (2023) - [i91]Ruijiang Dong, Feng Liu, Haoang Chi, Tongliang Liu, Mingming Gong, Gang Niu, Masashi Sugiyama, Bo Han:
Diversity-enhancing Generative Network for Few-shot Hypothesis Adaptation. CoRR abs/2307.05948 (2023) - [i90]Jialiang Tang, Shuo Chen, Gang Niu, Masashi Sugiyama, Chen Gong:
Distribution Shift Matters for Knowledge Distillation with Webly Collected Images. CoRR abs/2307.11469 (2023) - [i89]Penghui Yang
, Ming-Kun Xie, Chen-Chen Zong, Lei Feng
, Gang Niu, Masashi Sugiyama, Sheng-Jun Huang:
Multi-Label Knowledge Distillation. CoRR abs/2308.06453 (2023) - [i88]Wei Wang, Lei Feng, Yuchen Jiang, Gang Niu, Min-Ling Zhang, Masashi Sugiyama:
Binary Classification with Confidence Difference. CoRR abs/2310.05632 (2023) - [i87]Wentao Yu, Shuo Chen, Chen Gong, Gang Niu, Masashi Sugiyama:
Atom-Motif Contrastive Transformer for Molecular Property Prediction. CoRR abs/2310.07351 (2023) - [i86]Jianing Zhu, Geng Yu, Jiangchao Yao, Tongliang Liu, Gang Niu, Masashi Sugiyama, Bo Han:
Diversified Outlier Exposure for Out-of-Distribution Detection via Informative Extrapolation. CoRR abs/2310.13923 (2023) - [i85]Wei Wang, Takashi Ishida, Yu-Jie Zhang, Gang Niu, Masashi Sugiyama:
Learning with Complementary Labels Revisited: A Consistent Approach via Negative-Unlabeled Learning. CoRR abs/2311.15502 (2023) - 2022
- [j18]Yuangang Pan, Ivor W. Tsang
, Weijie Chen, Gang Niu, Masashi Sugiyama:
Fast and Robust Rank Aggregation against Model Misspecification. J. Mach. Learn. Res. 23: 23:1-23:35 (2022) - [j17]Songhua Wu, Tongliang Liu, Bo Han, Jun Yu, Gang Niu, Masashi Sugiyama:
Learning from Noisy Pairwise Similarity and Unlabeled Data. J. Mach. Learn. Res. 23: 307:1-307:34 (2022) - [j16]Zhuowei Wang, Jing Jiang, Bo Han, Lei Feng, Bo An, Gang Niu, Guodong Long:
SemiNLL: A Framework of Noisy-Label Learning by Semi-Supervised Learning. Trans. Mach. Learn. Res. 2022 (2022) - [j15]Jingfeng Zhang
, Xilie Xu, Bo Han, Tongliang Liu, Lizhen Cui, Gang Niu, Masashi Sugiyama:
NoiLin: Improving adversarial training and correcting stereotype of noisy labels. Trans. Mach. Learn. Res. 2022 (2022) - [c81]Masashi Sugiyama, Tongliang Liu
, Bo Han, Yang Liu, Gang Niu:
Learning and Mining with Noisy Labels. CIKM 2022: 5152-5155 - [c80]De Cheng, Tongliang Liu
, Yixiong Ning, Nannan Wang, Bo Han, Gang Niu, Xinbo Gao, Masashi Sugiyama:
Instance-Dependent Label-Noise Learning with Manifold-Regularized Transition Matrix Estimation. CVPR 2022: 16609-16618 - [c79]Haoang Chi, Feng Liu, Wenjing Yang, Long Lan, Tongliang Liu, Bo Han, Gang Niu, Mingyuan Zhou
, Masashi Sugiyama:
Meta Discovery: Learning to Discover Novel Classes given Very Limited Data. ICLR 2022 - [c78]Nan Lu, Zhao Wang, Xiaoxiao Li, Gang Niu, Qi Dou, Masashi Sugiyama:
Federated Learning from Only Unlabeled Data with Class-conditional-sharing Clients. ICLR 2022 - [c77]Haobo Wang, Ruixuan Xiao, Yixuan Li, Lei Feng, Gang Niu, Gang Chen, Junbo Zhao:
PiCO: Contrastive Label Disambiguation for Partial Label Learning. ICLR 2022 - [c76]Jiaheng Wei, Zhaowei Zhu, Hao Cheng, Tongliang Liu, Gang Niu, Yang Liu:
Learning with Noisy Labels Revisited: A Study Using Real-World Human Annotations. ICLR 2022 - [c75]Xiaobo Xia, Tongliang Liu, Bo Han, Mingming Gong, Jun Yu, Gang Niu, Masashi Sugiyama:
Sample Selection with Uncertainty of Losses for Learning with Noisy Labels. ICLR 2022 - [c74]Yu Yao, Tongliang Liu, Bo Han, Mingming Gong, Gang Niu, Masashi Sugiyama, Dacheng Tao:
Rethinking Class-Prior Estimation for Positive-Unlabeled Learning. ICLR 2022 - [c73]Fei Zhang, Lei Feng, Bo Han, Tongliang Liu, Gang Niu, Tao Qin, Masashi Sugiyama:
Exploiting Class Activation Value for Partial-Label Learning. ICLR 2022 - [c72]Yonggang Zhang, Mingming Gong, Tongliang Liu, Gang Niu, Xinmei Tian, Bo Han, Bernhard Schölkopf, Kun Zhang:
Adversarial Robustness Through the Lens of Causality. ICLR 2022 - [c71]Jianing Zhu, Jiangchao Yao, Bo Han, Jingfeng Zhang
, Tongliang Liu, Gang Niu, Jingren Zhou, Jianliang Xu, Hongxia Yang:
Reliable Adversarial Distillation with Unreliable Teachers. ICLR 2022 - [c70]Ruize Gao, Jiongxiao Wang, Kaiwen Zhou, Feng Liu, Binghui Xie, Gang Niu, Bo Han, James Cheng:
Fast and Reliable Evaluation of Adversarial Robustness with Minimum-Margin Attack. ICML 2022: 7144-7163 - [c69]Jiaheng Wei, Hangyu Liu, Tongliang Liu, Gang Niu, Masashi Sugiyama, Yang Liu:
To Smooth or Not? When Label Smoothing Meets Noisy Labels. ICML 2022: 23589-23614 - [c68]Shuo Yang, Erkun Yang, Bo Han, Yang Liu, Min Xu, Gang Niu, Tongliang Liu:
Estimating Instance-dependent Bayes-label Transition Matrix using a Deep Neural Network. ICML 2022: 25302-25312 - [c67]Jianan Zhou, Jianing Zhu, Jingfeng Zhang, Tongliang Liu, Gang Niu, Bo Han, Masashi Sugiyama:
Adversarial Training with Complementary Labels: On the Benefit of Gradually Informative Attacks. NeurIPS 2022 - [c66]Shuo Chen, Chen Gong, Jun Li, Jian Yang, Gang Niu, Masashi Sugiyama:
Learning Contrastive Embedding in Low-Dimensional Space. NeurIPS 2022 - [c65]Yuzhou Cao, Tianchi Cai, Lei Feng, Lihong Gu, Jinjie Gu, Bo An, Gang Niu, Masashi Sugiyama:
Generalizing Consistent Multi-Class Classification with Rejection to be Compatible with Arbitrary Losses. NeurIPS 2022 - [e1]Kamalika Chaudhuri, Stefanie Jegelka, Le Song, Csaba Szepesvári, Gang Niu, Sivan Sabato:
International Conference on Machine Learning, ICML 2022, 17-23 July 2022, Baltimore, Maryland, USA. Proceedings of Machine Learning Research 162, PMLR 2022 [contents] - [i84]Haobo Wang, Ruixuan Xiao, Yixuan Li, Lei Feng, Gang Niu, Gang Chen, Junbo Zhao:
PiCO: Contrastive Label Disambiguation for Partial Label Learning. CoRR abs/2201.08984 (2022) - [i83]Takashi Ishida, Ikko Yamane, Nontawat Charoenphakdee, Gang Niu, Masashi Sugiyama:
Is the Performance of My Deep Network Too Good to Be True? A Direct Approach to Estimating the Bayes Error in Binary Classification. CoRR abs/2202.00395 (2022) - [i82]Yinghua Gao, Dongxian Wu, Jingfeng Zhang, Guanhao Gan, Shu-Tao Xia, Gang Niu, Masashi Sugiyama:
On the Effectiveness of Adversarial Training against Backdoor Attacks. CoRR abs/2202.10627 (2022) - [i81]Nan Lu, Zhao Wang, Xiaoxiao Li, Gang Niu, Qi Dou, Masashi Sugiyama:
Federated Learning from Only Unlabeled Data with Class-Conditional-Sharing Clients. CoRR abs/2204.03304 (2022) - [i80]De Cheng, Tongliang Liu
, Yixiong Ning, Nannan Wang, Bo Han, Gang Niu, Xinbo Gao, Masashi Sugiyama:
Instance-Dependent Label-Noise Learning with Manifold-Regularized Transition Matrix Estimation. CoRR abs/2206.02791 (2022) - [i79]Ruize Gao, Jiongxiao Wang, Kaiwen Zhou, Feng Liu, Binghui Xie, Gang Niu, Bo Han, James Cheng:
Fast and Reliable Evaluation of Adversarial Robustness with Minimum-Margin Attack. CoRR abs/2206.07314 (2022) - [i78]Qiong Zhang, Aline Talhouk, Gang Niu, Xiaoxiao Li:
FedMT: Federated Learning with Mixed-type Labels. CoRR abs/2210.02042 (2022) - [i77]Jianan Zhou, Jianing Zhu, Jingfeng Zhang, Tongliang Liu
, Gang Niu, Bo Han, Masashi Sugiyama:
Adversarial Training with Complementary Labels: On the Benefit of Gradually Informative Attacks. CoRR abs/2211.00269 (2022) - [i76]Tingting Zhao, Ying Wang, Wei Sun, Yarui Chen, Gang Niu, Masashi Sugiyama:
Representation Learning for Continuous Action Spaces is Beneficial for Efficient Policy Learning. CoRR abs/2211.13257 (2022) - [i75]Hongxin Wei, Huiping Zhuang, Renchunzi Xie, Lei Feng, Gang Niu, Bo An, Yixuan Li:
Logit Clipping for Robust Learning against Label Noise. CoRR abs/2212.04055 (2022) - 2021
- [j14]Tomoya Sakai, Gang Niu, Masashi Sugiyama:
Information-Theoretic Representation Learning for Positive-Unlabeled Classification. Neural Comput. 33(1): 244-268 (2021) - [j13]Wenkai Xu, Gang Niu, Aapo Hyvärinen
, Masashi Sugiyama:
Direction Matters: On Influence-Preserving Graph Summarization and Max-Cut Principle for Directed Graphs. Neural Comput. 33(8): 2128-2162 (2021) - [c64]Qizhou Wang, Bo Han, Tongliang Liu, Gang Niu, Jian Yang, Chen Gong:
Tackling Instance-Dependent Label Noise via a Universal Probabilistic Model. AAAI 2021: 10183-10191 - [c63]Alon Jacovi, Gang Niu, Yoav Goldberg, Masashi Sugiyama:
Scalable Evaluation and Improvement of Document Set Expansion via Neural Positive-Unlabeled Learning. EACL 2021: 581-592 - [c62]Jingfeng Zhang
, Jianing Zhu, Gang Niu, Bo Han, Masashi Sugiyama, Mohan S. Kankanhalli:
Geometry-aware Instance-reweighted Adversarial Training. ICLR 2021 - [c61]Antonin Berthon, Bo Han, Gang Niu, Tongliang Liu, Masashi Sugiyama:
Confidence Scores Make Instance-dependent Label-noise Learning Possible. ICML 2021: 825-836 - [c60]Yuzhou Cao, Lei Feng, Yitian Xu, Bo An, Gang Niu, Masashi Sugiyama:
Learning from Similarity-Confidence Data. ICML 2021: 1272-1282 - [c59]Shuo Chen, Gang Niu, Chen Gong, Jun Li, Jian Yang, Masashi Sugiyama:
Large-Margin Contrastive Learning with Distance Polarization Regularizer. ICML 2021: 1673-1683 - [c58]Xuefeng Du, Jingfeng Zhang, Bo Han, Tongliang Liu, Yu Rong, Gang Niu, Junzhou Huang, Masashi Sugiyama:
Learning Diverse-Structured Networks for Adversarial Robustness. ICML 2021: 2880-2891 - [c57]Lei Feng, Senlin Shu, Nan Lu, Bo Han, Miao Xu
, Gang Niu, Bo An, Masashi Sugiyama:
Pointwise Binary Classification with Pairwise Confidence Comparisons. ICML 2021: 3252-3262 - [c56]Ruize Gao, Feng Liu, Jingfeng Zhang, Bo Han, Tongliang Liu, Gang Niu, Masashi Sugiyama:
Maximum Mean Discrepancy Test is Aware of Adversarial Attacks. ICML 2021: 3564-3575 - [c55]Xuefeng Li, Tongliang Liu, Bo Han, Gang Niu, Masashi Sugiyama:
Provably End-to-end Label-noise Learning without Anchor Points. ICML 2021: 6403-6413 - [c54]Nan Lu, Shida Lei, Gang Niu, Issei Sato, Masashi Sugiyama:
Binary Classification from Multiple Unlabeled Datasets via Surrogate Set Classification. ICML 2021: 7134-7144 - [c53]Songhua Wu, Xiaobo Xia, Tongliang Liu, Bo Han, Mingming Gong, Nannan Wang, Haifeng Liu, Gang Niu:
Class2Simi: A Noise Reduction Perspective on Learning with Noisy Labels. ICML 2021: 11285-11295 - [c52]Hanshu Yan, Jingfeng Zhang, Gang Niu, Jiashi Feng, Vincent Y. F. Tan, Masashi Sugiyama:
CIFS: Improving Adversarial Robustness of CNNs via Channel-wise Importance-based Feature Selection. ICML 2021: 11693-11703 - [c51]Yivan Zhang, Gang Niu, Masashi Sugiyama:
Learning Noise Transition Matrix from Only Noisy Labels via Total Variation Regularization. ICML 2021: 12501-12512 - [c50]Lei Feng
, Senlin Shu, Yuzhou Cao, Lue Tao, Hongxin Wei, Tao Xiang
, Bo An, Gang Niu:
Multiple-Instance Learning from Similar and Dissimilar Bags. KDD 2021: 374-382 - [c49]Yu Yao, Tongliang Liu, Mingming Gong, Bo Han, Gang Niu, Kun Zhang:
Instance-dependent Label-noise Learning under a Structural Causal Model. NeurIPS 2021: 4409-4420 - [c48]Qizhou Wang, Feng Liu, Bo Han, Tongliang Liu, Chen Gong, Gang Niu, Mingyuan Zhou, Masashi Sugiyama:
Probabilistic Margins for Instance Reweighting in Adversarial Training. NeurIPS 2021: 23258-23269 - [c47]Yingbin Bai, Erkun Yang, Bo Han, Yanhua Yang, Jiatong Li, Yinian Mao, Gang Niu, Tongliang Liu:
Understanding and Improving Early Stopping for Learning with Noisy Labels. NeurIPS 2021: 24392-24403 - [i74]Qizhou Wang, Bo Han, Tongliang Liu, Gang Niu, Jian Yang, Chen Gong:
Tackling Instance-Dependent Label Noise via a Universal Probabilistic Model. CoRR abs/2101.05467 (2021) - [i73]Shida Lei, Nan Lu, Gang Niu, Issei Sato, Masashi Sugiyama:
Binary Classification from Multiple Unlabeled Datasets via Surrogate Set Classification. CoRR abs/2102.00678 (2021) - [i72]Xuefeng Du, Jingfeng Zhang, Bo Han, Tongliang Liu, Yu Rong, Gang Niu, Junzhou Huang, Masashi Sugiyama:
Learning Diverse-Structured Networks for Adversarial Robustness. CoRR abs/2102.01886 (2021) - [i71]Xuefeng Li, Tongliang Liu, Bo Han, Gang Niu, Masashi Sugiyama:
Provably End-to-end Label-Noise Learning without Anchor Points. CoRR abs/2102.02400 (2021) - [i70]Yivan Zhang, Gang Niu, Masashi Sugiyama:
Learning Noise Transition Matrix from Only Noisy Labels via Total Variation Regularization. CoRR abs/2102.02414 (2021) - [i69]Jianing Zhu, Jingfeng Zhang, Bo Han, Tongliang Liu, Gang Niu, Hongxia Yang, Mohan S. Kankanhalli, Masashi Sugiyama:
Understanding the Interaction of Adversarial Training with Noisy Labels. CoRR abs/2102.03482 (2021) - [i68]Haoang Chi, Feng Liu, Wenjing Yang, Long Lan, Tongliang Liu, Gang Niu, Bo Han:
Meta Discovery: Learning to Discover Novel Classes given Very Limited Data. CoRR abs/2102.04002 (2021) - [i67]Hanshu Yan, Jingfeng Zhang, Gang Niu, Jiashi Feng, Vincent Y. F. Tan, Masashi Sugiyama:
CIFS: Improving Adversarial Robustness of CNNs via Channel-wise Importance-based Feature Selection. CoRR abs/2102.05311 (2021) - [i66]Yuzhou Cao, Lei Feng, Yitian Xu, Bo An, Gang Niu, Masashi Sugiyama:
Learning from Similarity-Confidence Data. CoRR abs/2102.06879 (2021) - [i65]Chen Chen, Jingfeng Zhang, Xilie Xu, Tianlei Hu, Gang Niu, Gang Chen, Masashi Sugiyama:
Guided Interpolation for Adversarial Training. CoRR abs/2102.07327 (2021) - [i64]Shuo Yang, Erkun Yang, Bo Han, Yang Liu, Min Xu, Gang Niu, Tongliang Liu:
Estimating Instance-dependent Label-noise Transition Matrix using DNNs. CoRR abs/2105.13001 (2021) - [i63]Jingfeng Zhang, Xilie Xu, Bo Han, Tongliang Liu, Gang Niu, Lizhen Cui, Masashi Sugiyama:
NoiLIn: Do Noisy Labels Always Hurt Adversarial Training? CoRR abs/2105.14676 (2021) - [i62]Xiaobo Xia, Tongliang Liu, Bo Han, Mingming Gong, Jun Yu, Gang Niu, Masashi Sugiyama:
Sample Selection with Uncertainty of Losses for Learning with Noisy Labels. CoRR abs/2106.00445 (2021) - [i61]Xiaobo Xia, Tongliang Liu, Bo Han, Mingming Gong, Jun Yu, Gang Niu, Masashi Sugiyama:
Instance Correction for Learning with Open-set Noisy Labels. CoRR abs/2106.00455 (2021) - [i60]Jiaheng Wei, Hangyu Liu, Tongliang Liu, Gang Niu, Yang Liu:
Understanding (Generalized) Label Smoothing when Learning with Noisy Labels. CoRR abs/2106.04149 (2021) - [i59]Jianing Zhu, Jiangchao Yao, Bo Han, Jingfeng Zhang, Tongliang Liu, Gang Niu, Jingren Zhou, Jianliang Xu, Hongxia Yang:
Reliable Adversarial Distillation with Unreliable Teachers. CoRR abs/2106.04928 (2021) - [i58]Jiaqi Lv, Lei Feng, Miao Xu, Bo An, Gang Niu, Xin Geng, Masashi Sugiyama:
On the Robustness of Average Losses for Partial-Label Learning. CoRR abs/2106.06152 (2021) - [i57]Yonggang Zhang, Mingming Gong, Tongliang Liu, Gang Niu, Xinmei Tian, Bo Han, Bernhard Schölkopf, Kun Zhang:
Adversarial Robustness through the Lens of Causality. CoRR abs/2106.06196 (2021) - [i56]Qizhou Wang, Feng Liu, Bo Han, Tongliang Liu, Chen Gong, Gang Niu, Mingyuan Zhou, Masashi Sugiyama:
Probabilistic Margins for Instance Reweighting in Adversarial Training. CoRR abs/2106.07904 (2021) - [i55]Yuzhou Cao, Lei Feng, Senlin Shu, Yitian Xu, Bo An, Gang Niu, Masashi Sugiyama:
Multi-Class Classification from Single-Class Data with Confidences. CoRR abs/2106.08864 (2021) - [i54]Ruize Gao, Feng Liu, Kaiwen Zhou, Gang Niu, Bo Han, James Cheng:
Local Reweighting for Adversarial Training. CoRR abs/2106.15776 (2021) - [i53]Yingbin Bai, Erkun Yang, Bo Han, Yanhua Yang, Jiatong Li, Yinian Mao, Gang Niu, Tongliang Liu:
Understanding and Improving Early Stopping for Learning with Noisy Labels. CoRR abs/2106.15853 (2021) - [i52]Yu Yao, Tongliang Liu, Mingming Gong, Bo Han, Gang Niu, Kun Zhang:
Instance-dependent Label-noise Learning under a Structural Causal Model. CoRR abs/2109.02986 (2021) - [i51]Cheng-Yu Hsieh, Wei-I Lin, Miao Xu, Gang Niu, Hsuan-Tien Lin, Masashi Sugiyama:
Active Refinement for Multi-Label Learning: A Pseudo-Label Approach. CoRR abs/2109.14676 (2021) - [i50]Jiaheng Wei, Zhaowei Zhu, Hao Cheng, Tongliang Liu, Gang Niu, Yang Liu:
Learning with Noisy Labels Revisited: A Study Using Real-World Human Annotations. CoRR abs/2110.12088 (2021) - 2020
- [c46]Chao Li, Mohammad Emtiyaz Khan, Zhun Sun, Gang Niu, Bo Han, Shengli Xie, Qibin Zhao:
Beyond Unfolding: Exact Recovery of Latent Convex Tensor Decomposition Under Reshuffling. AAAI 2020: 4602-4609 - [c45]Nan Lu, Tianyi Zhang, Gang Niu, Masashi Sugiyama:
Mitigating Overfitting in Supervised Classification from Two Unlabeled Datasets: A Consistent Risk Correction Approach. AISTATS 2020: 1115-1125 - [c44]Chun Wang, Bo Han, Shirui Pan, Jing Jiang, Gang Niu, Guodong Long:
Cross-Graph: Robust and Unsupervised Embedding for Attributed Graphs with Corrupted Structure. ICDM 2020: 571-580 - [c43]Yu-Ting Chou, Gang Niu, Hsuan-Tien Lin, Masashi Sugiyama:
Unbiased Risk Estimators Can Mislead: A Case Study of Learning with Complementary Labels. ICML 2020: 1929-1938 - [c42]Lei Feng, Takuo Kaneko, Bo Han, Gang Niu, Bo An, Masashi Sugiyama:
Learning with Multiple Complementary Labels. ICML 2020: 3072-3081 - [c41]Bo Han, Gang Niu, Xingrui Yu, Quanming Yao, Miao Xu, Ivor W. Tsang
, Masashi Sugiyama:
SIGUA: Forgetting May Make Learning with Noisy Labels More Robust. ICML 2020: 4006-4016 - [c40]Takashi Ishida, Ikko Yamane, Tomoya Sakai, Gang Niu, Masashi Sugiyama:
Do We Need Zero Training Loss After Achieving Zero Training Error? ICML 2020: 4604-4614 - [c39]Jiaqi Lv, Miao Xu, Lei Feng, Gang Niu, Xin Geng, Masashi Sugiyama:
Progressive Identification of True Labels for Partial-Label Learning. ICML 2020: 6500-6510 - [c38]Quanming Yao, Hansi Yang, Bo Han, Gang Niu, James Tin-Yau Kwok:
Searching to Exploit Memorization Effect in Learning with Noisy Labels. ICML 2020: 10789-10798 - [c37]Jingfeng Zhang, Xilie Xu, Bo Han, Gang Niu, Lizhen Cui, Masashi Sugiyama, Mohan S. Kankanhalli:
Attacks Which Do Not Kill Training Make Adversarial Learning Stronger. ICML 2020: 11278-11287 - [c36]Tongtong Fang, Nan Lu, Gang Niu, Masashi Sugiyama:
Rethinking Importance Weighting for Deep Learning under Distribution Shift. NeurIPS 2020 - [c35]Lei Feng, Jiaqi Lv, Bo Han, Miao Xu, Gang Niu, Xin Geng, Bo An, Masashi Sugiyama:
Provably Consistent Partial-Label Learning. NeurIPS 2020 - [c34]Xiaobo Xia, Tongliang Liu, Bo Han, Nannan Wang, Mingming Gong, Haifeng Liu, Gang Niu, Dacheng Tao, Masashi Sugiyama:
Part-dependent Label Noise: Towards Instance-dependent Label Noise. NeurIPS 2020 - [c33]Yu Yao, Tongliang Liu, Bo Han, Mingming Gong, Jiankang Deng, Gang Niu, Masashi Sugiyama:
Dual T: Reducing Estimation Error for Transition Matrix in Label-noise Learning. NeurIPS 2020 - [i49]Antonin Berthon, Bo Han, Gang Niu, Tongliang Liu, Masashi Sugiyama:
Confidence Scores Make Instance-dependent Label-noise Learning Possible. CoRR abs/2001.03772 (2020) - [i48]Yu Yao, Tongliang Liu, Bo Han, Mingming Gong, Gang Niu, Masashi Sugiyama, Dacheng Tao:
Towards Mixture Proportion Estimation without Irreducibility. CoRR abs/2002.03673 (2020) - [i47]Songhua Wu, Xiaobo Xia, Tongliang Liu, Bo Han, Mingming Gong, Nannan Wang, Haifeng Liu, Gang Niu:
Multi-Class Classification from Noisy-Similarity-Labeled Data. CoRR abs/2002.06508 (2020) - [i46]Jiaqi Lv, Miao Xu, Lei Feng, Gang Niu, Xin Geng, Masashi Sugiyama:
Progressive Identification of True Labels for Partial-Label Learning. CoRR abs/2002.08053 (2020) - [i45]Takashi Ishida, Ikko Yamane, Tomoya Sakai, Gang Niu, Masashi Sugiyama:
Do We Need Zero Training Loss After Achieving Zero Training Error? CoRR abs/2002.08709 (2020) - [i44]Jingfeng Zhang, Xilie Xu, Bo Han, Gang Niu, Lizhen Cui, Masashi Sugiyama, Mohan S. Kankanhalli:
Attacks Which Do Not Kill Training Make Adversarial Learning Stronger. CoRR abs/2002.11242 (2020) - [i43]Tongtong Fang, Nan Lu, Gang Niu, Masashi Sugiyama:
Rethinking Importance Weighting for Deep Learning under Distribution Shift. CoRR abs/2006.04662 (2020) - [i42]Yu Yao, Tongliang Liu, Bo Han, Mingming Gong, Jiankang Deng, Gang Niu, Masashi Sugiyama:
Dual T: Reducing Estimation Error for Transition Matrix in Label-noise Learning. CoRR abs/2006.07805 (2020) - [i41]Songhua Wu, Xiaobo Xia, Tongliang Liu, Bo Han, Mingming Gong, Nannan Wang, Haifeng Liu, Gang Niu:
Class2Simi: A New Perspective on Learning with Label Noise. CoRR abs/2006.07831 (2020) - [i40]Xiaobo Xia, Tongliang Liu, Bo Han, Nannan Wang, Mingming Gong, Haifeng Liu, Gang Niu, Dacheng Tao, Masashi Sugiyama:
Parts-dependent Label Noise: Towards Instance-dependent Label Noise. CoRR abs/2006.07836 (2020) - [i39]Yu-Ting Chou, Gang Niu, Hsuan-Tien Lin, Masashi Sugiyama:
Unbiased Risk Estimators Can Mislead: A Case Study of Learning with Complementary Labels. CoRR abs/2007.02235 (2020) - [i38]Lei Feng, Jiaqi Lv, Bo Han, Miao Xu, Gang Niu, Xin Geng, Bo An, Masashi Sugiyama:
Provably Consistent Partial-Label Learning. CoRR abs/2007.08929 (2020) - [i37]Jingfeng Zhang, Jianing Zhu, Gang Niu, Bo Han, Masashi Sugiyama, Mohan S. Kankanhalli:
Geometry-aware Instance-reweighted Adversarial Training. CoRR abs/2010.01736 (2020) - [i36]Lei Feng, Senlin Shu, Nan Lu, Bo Han, Miao Xu
, Gang Niu, Bo An, Masashi Sugiyama:
Pointwise Binary Classification with Pairwise Confidence Comparisons. CoRR abs/2010.01875 (2020) - [i35]Ruize Gao, Feng Liu, Jingfeng Zhang, Bo Han, Tongliang Liu, Gang Niu, Masashi Sugiyama:
Maximum Mean Discrepancy is Aware of Adversarial Attacks. CoRR abs/2010.11415 (2020) - [i34]Bo Han, Quanming Yao, Tongliang Liu, Gang Niu, Ivor W. Tsang, James T. Kwok, Masashi Sugiyama:
A Survey of Label-noise Representation Learning: Past, Present and Future. CoRR abs/2011.04406 (2020) - [i33]Zhuowei Wang, Jing Jiang, Bo Han, Lei Feng, Bo An, Gang Niu, Guodong Long:
SemiNLL: A Framework of Noisy-Label Learning by Semi-Supervised Learning. CoRR abs/2012.00925 (2020)
2010 – 2019
- 2019
- [c32]Nan Lu, Gang Niu, Aditya Krishna Menon, Masashi Sugiyama:
On the Minimal Supervision for Training Any Binary Classifier from Only Unlabeled Data. ICLR (Poster) 2019 - [c31]Yu-Guan Hsieh, Gang Niu, Masashi Sugiyama:
Classification from Positive, Unlabeled and Biased Negative Data. ICML 2019: 2820-2829 - [c30]Takashi Ishida, Gang Niu, Aditya Krishna Menon, Masashi Sugiyama:
Complementary-Label Learning for Arbitrary Losses and Models. ICML 2019: 2971-2980 - [c29]Xingrui Yu, Bo Han, Jiangchao Yao, Gang Niu, Ivor W. Tsang
, Masashi Sugiyama:
How does Disagreement Help Generalization against Label Corruption? ICML 2019: 7164-7173 - [c28]Liyuan Xu, Junya Honda, Gang Niu, Masashi Sugiyama:
Uncoupled Regression from Pairwise Comparison Data. NeurIPS 2019: 3994-4004 - [c27]Xiaobo Xia, Tongliang Liu, Nannan Wang, Bo Han, Chen Gong, Gang Niu, Masashi Sugiyama:
Are Anchor Points Really Indispensable in Label-Noise Learning? NeurIPS 2019: 6835-6846 - [i32]Xingrui Yu, Bo Han, Jiangchao Yao, Gang Niu, Ivor W. Tsang, Masashi Sugiyama:
How does Disagreement Help Generalization against Label Corruption? CoRR abs/1901.04215 (2019) - [i31]Miao Xu
, Bingcong Li, Gang Niu, Bo Han, Masashi Sugiyama:
Revisiting Sample Selection Approach to Positive-Unlabeled Learning: Turning Unlabeled Data into Positive rather than Negative. CoRR abs/1901.10155 (2019) - [i30]Feng Liu, Jie Lu, Bo Han, Gang Niu, Guangquan Zhang, Masashi Sugiyama:
Butterfly: A Panacea for All Difficulties in Wildly Unsupervised Domain Adaptation. CoRR abs/1905.07720 (2019) - [i29]Yuangang Pan, Weijie Chen, Gang Niu, Ivor W. Tsang, Masashi Sugiyama:
Fast and Robust Rank Aggregation against Model Misspecification. CoRR abs/1905.12341 (2019) - [i28]Liyuan Xu, Junya Honda, Gang Niu, Masashi Sugiyama:
Uncoupled Regression from Pairwise Comparison Data. CoRR abs/1905.13659 (2019) - [i27]Xiaobo Xia, Tongliang Liu, Nannan Wang, Bo Han, Chen Gong, Gang Niu, Masashi Sugiyama:
Are Anchor Points Really Indispensable in Label-Noise Learning? CoRR abs/1906.00189 (2019) - [i26]Wenkai Xu, Gang Niu, Aapo Hyvärinen, Masashi Sugiyama:
Direction Matters: On Influence-Preserving Graph Summarization and Max-cut Principle for Directed Graphs. CoRR abs/1907.09588 (2019) - [i25]Nan Lu, Tianyi Zhang, Gang Niu, Masashi Sugiyama:
Mitigating Overfitting in Supervised Classification from Two Unlabeled Datasets: A Consistent Risk Correction Approach. CoRR abs/1910.08974 (2019) - [i24]Alon Jacovi, Gang Niu, Yoav Goldberg, Masashi Sugiyama:
Scalable Evaluation and Improvement of Document Set Expansion via Neural Positive-Unlabeled Learning. CoRR abs/1910.13339 (2019) - [i23]Hansi Yang, Quanming Yao, Bo Han, Gang Niu:
Searching to Exploit Memorization Effect in Learning from Corrupted Labels. CoRR abs/1911.02377 (2019) - [i22]Jingfeng Zhang, Bo Han, Gang Niu, Tongliang Liu, Masashi Sugiyama:
Where is the Bottleneck of Adversarial Learning with Unlabeled Data? CoRR abs/1911.08696 (2019) - 2018
- [j12]Tomoya Sakai, Gang Niu, Masashi Sugiyama
:
Semi-supervised AUC optimization based on positive-unlabeled learning. Mach. Learn. 107(4): 767-794 (2018) - [j11]Tomoya Sakai, Gang Niu, Masashi Sugiyama
:
Correction to: Semi-supervised AUC optimization based on positive-unlabeled learning. Mach. Learn. 107(4): 795 (2018) - [j10]Hiroaki Sasaki, Voot Tangkaratt, Gang Niu, Masashi Sugiyama
:
Sufficient Dimension Reduction via Direct Estimation of the Gradients of Logarithmic Conditional Densities. Neural Comput. 30(2) (2018) - [c26]Han Bao
, Gang Niu, Masashi Sugiyama:
Classification from Pairwise Similarity and Unlabeled Data. ICML 2018: 461-470 - [c25]Weihua Hu, Gang Niu, Issei Sato, Masashi Sugiyama:
Does Distributionally Robust Supervised Learning Give Robust Classifiers? ICML 2018: 2034-2042 - [c24]Sheng-Jun Huang, Miao Xu
, Ming-Kun Xie, Masashi Sugiyama
, Gang Niu, Songcan Chen:
Active Feature Acquisition with Supervised Matrix Completion. KDD 2018: 1571-1579 - [c23]Bo Han, Jiangchao Yao, Gang Niu, Mingyuan Zhou, Ivor W. Tsang
, Ya Zhang, Masashi Sugiyama:
Masking: A New Perspective of Noisy Supervision. NeurIPS 2018: 5841-5851 - [c22]Takashi Ishida, Gang Niu, Masashi Sugiyama:
Binary Classification from Positive-Confidence Data. NeurIPS 2018: 5921-5932 - [c21]Bo Han, Quanming Yao, Xingrui Yu, Gang Niu, Miao Xu, Weihua Hu, Ivor W. Tsang
, Masashi Sugiyama:
Co-teaching: Robust training of deep neural networks with extremely noisy labels. NeurIPS 2018: 8536-8546 - [i21]Han Bao, Gang Niu, Masashi Sugiyama:
Classification from Pairwise Similarity and Unlabeled Data. CoRR abs/1802.04381 (2018) - [i20]Sheng-Jun Huang, Miao Xu, Ming-Kun Xie, Masashi Sugiyama, Gang Niu, Songcan Chen:
Active Feature Acquisition with Supervised Matrix Completion. CoRR abs/1802.05380 (2018) - [i19]Bo Han, Quanming Yao, Xingrui Yu, Gang Niu, Miao Xu, Weihua Hu, Ivor W. Tsang, Masashi Sugiyama:
Co-sampling: Training Robust Networks for Extremely Noisy Supervision. CoRR abs/1804.06872 (2018) - [i18]Bo Han, Jiangchao Yao, Gang Niu, Mingyuan Zhou, Ivor W. Tsang, Ya Zhang, Masashi Sugiyama:
Masking: A New Perspective of Noisy Supervision. CoRR abs/1805.08193 (2018) - [i17]Miao Xu
, Gang Niu, Bo Han, Ivor W. Tsang, Zhi-Hua Zhou, Masashi Sugiyama:
Matrix Co-completion for Multi-label Classification with Missing Features and Labels. CoRR abs/1805.09156 (2018) - [i16]Nan Lu, Gang Niu, Aditya Krishna Menon, Masashi Sugiyama:
On the Minimal Supervision for Training Any Binary Classifier from Only Unlabeled Data. CoRR abs/1808.10585 (2018) - [i15]Masahiro Kato, Liyuan Xu, Gang Niu, Masashi Sugiyama:
Alternate Estimation of a Classifier and the Class-Prior from Positive and Unlabeled Data. CoRR abs/1809.05710 (2018) - [i14]Bo Han, Gang Niu, Jiangchao Yao, Xingrui Yu, Miao Xu, Ivor W. Tsang, Masashi Sugiyama:
Pumpout: A Meta Approach for Robustly Training Deep Neural Networks with Noisy Labels. CoRR abs/1809.11008 (2018) - [i13]Yu-Guan Hsieh, Gang Niu, Masashi Sugiyama:
Classification from Positive, Unlabeled and Biased Negative Data. CoRR abs/1810.00846 (2018) - [i12]Takashi Ishida, Gang Niu, Aditya Krishna Menon, Masashi Sugiyama:
Complementary-Label Learning for Arbitrary Losses and Models. CoRR abs/1810.04327 (2018) - 2017
- [j9]Hiroaki Sasaki, Takafumi Kanamori, Aapo Hyvärinen, Gang Niu, Masashi Sugiyama:
Mode-Seeking Clustering and Density Ridge Estimation via Direct Estimation of Density-Derivative-Ratios. J. Mach. Learn. Res. 18: 180:1-180:47 (2017) - [j8]Marthinus Christoffel du Plessis, Gang Niu, Masashi Sugiyama
:
Class-prior estimation for learning from positive and unlabeled data. Mach. Learn. 106(4): 463-492 (2017) - [c20]Hiroaki Shiino, Hiroaki Sasaki, Gang Niu, Masashi Sugiyama:
Whitening-Free Least-Squares Non-Gaussian Component Analysis. ACML 2017: 375-390 - [c19]Tomoya Sakai, Marthinus Christoffel du Plessis, Gang Niu, Masashi Sugiyama:
Semi-Supervised Classification Based on Classification from Positive and Unlabeled Data. ICML 2017: 2998-3006 - [c18]Ryuichi Kiryo, Gang Niu, Marthinus Christoffel du Plessis, Masashi Sugiyama:
Positive-Unlabeled Learning with Non-Negative Risk Estimator. NIPS 2017: 1675-1685 - [c17]Takashi Ishida, Gang Niu, Weihua Hu, Masashi Sugiyama:
Learning from Complementary Labels. NIPS 2017: 5639-5649 - [i11]Ryuichi Kiryo, Gang Niu, Marthinus Christoffel du Plessis, Masashi Sugiyama:
Positive-Unlabeled Learning with Non-Negative Risk Estimator. CoRR abs/1703.00593 (2017) - [i10]Tomoya Sakai, Gang Niu, Masashi Sugiyama:
Semi-Supervised AUC Optimization based on Positive-Unlabeled Learning. CoRR abs/1705.01708 (2017) - [i9]Takashi Ishida, Gang Niu, Masashi Sugiyama:
Learning from Complementary Labels. CoRR abs/1705.07541 (2017) - [i8]Tomoya Sakai, Gang Niu, Masashi Sugiyama:
Estimation of Squared-Loss Mutual Information from Positive and Unlabeled Data. CoRR abs/1710.05359 (2017) - [i7]Takashi Ishida, Gang Niu, Masashi Sugiyama:
Binary Classification from Positive-Confidence Data. CoRR abs/1710.07138 (2017) - 2016
- [j7]Hiroaki Sasaki, Yung-Kyun Noh, Gang Niu, Masashi Sugiyama
:
Direct Density Derivative Estimation. Neural Comput. 28(6): 1101-1140 (2016) - [c16]Hiroaki Sasaki, Gang Niu, Masashi Sugiyama:
Non-Gaussian Component Analysis with Log-Density Gradient Estimation. AISTATS 2016: 1177-1185 - [c15]Gang Niu, Marthinus Christoffel du Plessis, Tomoya Sakai, Yao Ma, Masashi Sugiyama:
Theoretical Comparisons of Positive-Unlabeled Learning against Positive-Negative Learning. NIPS 2016: 1199-1207 - [i6]Gang Niu, Marthinus Christoffel du Plessis, Tomoya Sakai, Masashi Sugiyama:
Theoretical Comparisons of Learning from Positive-Negative, Positive-Unlabeled, and Negative-Unlabeled Data. CoRR abs/1603.03130 (2016) - [i5]Tomoya Sakai, Marthinus Christoffel du Plessis, Gang Niu, Masashi Sugiyama:
Beyond the Low-density Separation Principle: A Novel Approach to Semi-supervised Learning. CoRR abs/1605.06955 (2016) - [i4]Marthinus Christoffel du Plessis, Gang Niu, Masashi Sugiyama:
Class-prior Estimation for Learning from Positive and Unlabeled Data. CoRR abs/1611.01586 (2016) - 2015
- [c14]Marthinus Christoffel du Plessis, Gang Niu, Masashi Sugiyama:
Class-prior Estimation for Learning from Positive and Unlabeled Data. ACML 2015: 221-236 - [c13]Tingting Zhao, Gang Niu, Ning Xie, Jucheng Yang, Masashi Sugiyama:
Regularized Policy Gradients: Direct Variance Reduction in Policy Gradient Estimation. ACML 2015: 333-348 - [c12]Marthinus Christoffel du Plessis, Gang Niu, Masashi Sugiyama:
Convex Formulation for Learning from Positive and Unlabeled Data. ICML 2015: 1386-1394 - 2014
- [j6]Masashi Sugiyama
, Gang Niu, Makoto Yamada
, Manabu Kimura, Hirotaka Hachiya:
Information-Maximization Clustering Based on Squared-Loss Mutual Information. Neural Comput. 26(1): 84-131 (2014) - [j5]Gang Niu, Bo Dai, Makoto Yamada
, Masashi Sugiyama
:
Information-Theoretic Semi-Supervised Metric Learning via Entropy Regularization. Neural Comput. 26(8): 1717-1762 (2014) - [j4]Daniele Calandriello, Gang Niu, Masashi Sugiyama
:
Semi-supervised information-maximization clustering. Neural Networks 57: 103-111 (2014) - [c11]Gang Niu, Bo Dai, Marthinus Christoffel du Plessis, Masashi Sugiyama:
Transductive Learning with Multi-class Volume Approximation. ICML 2014: 1377-1385 - [c10]Marthinus Christoffel du Plessis, Gang Niu, Masashi Sugiyama:
Analysis of Learning from Positive and Unlabeled Data. NIPS 2014: 703-711 - [i3]Gang Niu, Bo Dai, Marthinus Christoffel du Plessis, Masashi Sugiyama:
Transductive Learning with Multi-class Volume Approximation. CoRR abs/1402.0288 (2014) - 2013
- [j3]Gang Niu, Bo Dai, Lin Shang, Masashi Sugiyama:
Maximum volume clustering: a new discriminative clustering approach. J. Mach. Learn. Res. 14(1): 2641-2687 (2013) - [c9]Gang Niu, Wittawat Jitkrittum, Bo Dai, Hirotaka Hachiya, Masashi Sugiyama:
Squared-loss Mutual Information Regularization: A Novel Information-theoretic Approach to Semi-supervised Learning. ICML (3) 2013: 10-18 - [c8]Marthinus Christoffel du Plessis, Gang Niu, Masashi Sugiyama
:
Clustering Unclustered Data: Unsupervised Binary Labeling of Two Datasets Having Different Class Balances. TAAI 2013: 1-6 - [i2]Daniele Calandriello, Gang Niu, Masashi Sugiyama:
Semi-Supervised Information-Maximization Clustering. CoRR abs/1304.8020 (2013) - 2012
- [j2]Tingting Zhao, Hirotaka Hachiya, Gang Niu, Masashi Sugiyama
:
Analysis and improvement of policy gradient estimation. Neural Networks 26: 118-129 (2012) - [c7]Gang Niu, Bo Dai, Makoto Yamada, Masashi Sugiyama:
Information-theoretic Semi-supervised Metric Learning via Entropy Regularization. ICML 2012 - [i1]Gang Niu, Bo Dai, Makoto Yamada, Masashi Sugiyama:
Information-theoretic Semi-supervised Metric Learning via Entropy Regularization. CoRR abs/1206.4614 (2012) - 2011
- [j1]Yangsheng Ji, Jiajun Chen, Gang Niu, Lin Shang, Xinyu Dai:
Transfer Learning via Multi-View Principal Component Analysis. J. Comput. Sci. Technol. 26(1): 81-98 (2011) - [c6]Tingting Zhao, Hirotaka Hachiya, Gang Niu, Masashi Sugiyama:
Analysis and Improvement of Policy Gradient Estimation. NIPS 2011: 262-270 - [c5]Makoto Yamada, Gang Niu, Jun Takagi, Masashi Sugiyama:
Suffcient Component Analysis. ACML 2011: 247-262 - [c4]Gang Niu, Bo Dai, Lin Shang, Masashi Sugiyama:
Maximum Volume Clustering. AISTATS 2011: 561-569 - 2010
- [c3]Bo Dai, Bao-Gang Hu
, Gang Niu:
Bayesian Maximum Margin Clustering. ICDM 2010: 108-117 - [c2]Gang Niu, Bo Dai, Lin Shang, Yangsheng Ji:
Rough Margin Based Core Vector Machine. PAKDD (1) 2010: 134-141 - [c1]Bo Dai, Gang Niu:
Compact Margin Machine. PAKDD (2) 2010: 507-514
Coauthor Index

manage site settings
To protect your privacy, all features that rely on external API calls from your browser are turned off by default. You need to opt-in for them to become active. All settings here will be stored as cookies with your web browser. For more information see our F.A.Q.
Unpaywalled article links
Add open access links from to the list of external document links (if available).
Privacy notice: By enabling the option above, your browser will contact the API of unpaywall.org to load hyperlinks to open access articles. Although we do not have any reason to believe that your call will be tracked, we do not have any control over how the remote server uses your data. So please proceed with care and consider checking the Unpaywall privacy policy.
Archived links via Wayback Machine
For web page which are no longer available, try to retrieve content from the of the Internet Archive (if available).
Privacy notice: By enabling the option above, your browser will contact the API of archive.org to check for archived content of web pages that are no longer available. Although we do not have any reason to believe that your call will be tracked, we do not have any control over how the remote server uses your data. So please proceed with care and consider checking the Internet Archive privacy policy.
Reference lists
Add a list of references from ,
, and
to record detail pages.
load references from crossref.org and opencitations.net
Privacy notice: By enabling the option above, your browser will contact the APIs of crossref.org, opencitations.net, and semanticscholar.org to load article reference information. Although we do not have any reason to believe that your call will be tracked, we do not have any control over how the remote server uses your data. So please proceed with care and consider checking the Crossref privacy policy and the OpenCitations privacy policy, as well as the AI2 Privacy Policy covering Semantic Scholar.
Citation data
Add a list of citing articles from and
to record detail pages.
load citations from opencitations.net
Privacy notice: By enabling the option above, your browser will contact the API of opencitations.net and semanticscholar.org to load citation information. Although we do not have any reason to believe that your call will be tracked, we do not have any control over how the remote server uses your data. So please proceed with care and consider checking the OpenCitations privacy policy as well as the AI2 Privacy Policy covering Semantic Scholar.
OpenAlex data
Load additional information about publications from .
Privacy notice: By enabling the option above, your browser will contact the API of openalex.org to load additional information. Although we do not have any reason to believe that your call will be tracked, we do not have any control over how the remote server uses your data. So please proceed with care and consider checking the information given by OpenAlex.
last updated on 2024-11-15 20:35 CET by the dblp team
all metadata released as open data under CC0 1.0 license
see also: Terms of Use | Privacy Policy | Imprint