Abstract
Recent development in deep learning techniques have had a massive impact in the field of agricultural disease detection. The negative impact of pest and bacterial diseases to rice plants are well known, and for regions where rice is staple, this is issue carries a lot of weight. This work proposes a high accuracy, transfer learned model that can provide a mobile solution for farmers and agricultural organizations to detect rice leaf diseases at hand. This study also utilizes a generative adversarial network to balance the number of disease samples. We compare our model to other transfer learning architectures as well. The presented model tested on a GAN augmented dataset, achieves an average cross validation accuracy of 98.79% outperforming paradigm classification architectures. The model is also compared on 3 different datasets, without the GAN augmentation, establishing benchmark performance of 98.38% average accuracy.
Export citation and abstract BibTeX RIS
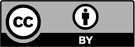
Content from this work may be used under the terms of the Creative Commons Attribution 3.0 licence. Any further distribution of this work must maintain attribution to the author(s) and the title of the work, journal citation and DOI.