Open Access
ARTICLE
Block-Wise Neural Network for Brain Tumor Identification in Magnetic Resonance Images
1 Radiological Sciences Department, College of Applied Medical Sciences, Najran University, Najran 61441, Saudi Arabia
2 Department of Computer Science, COMSATS University Islamabad, Sahiwal Campus, Sahiwal, 57000, Pakistan
3 Department of Computer Science, Bahauddin Zakariya University, Multan 66000, Pakistan
4 Electrical Engineering Department, College of Engineering, Najran University, Najran, 61441, Saudi Arabia
* Corresponding Author: Ahmad Shaf. Email:
Computers, Materials & Continua 2022, 73(3), 5735-5753. https://doi.org/10.32604/cmc.2022.031747
Received 26 April 2022; Accepted 09 June 2022; Issue published 28 July 2022
Abstract
The precise brain tumor diagnosis is critical and shows a vital role in the medical support for treating tumor patients. Manual brain tumor segmentation for cancer analysis from many Magnetic Resonance Images (MRIs) created in medical practice is a problematic and timewasting task for experts. As a result, there is a critical necessity for more accurate computer-aided methods for early tumor detection. To remove this gap, we enhanced the computational power of a computer-aided system by proposing a fine-tuned Block-Wise Visual Geometry Group19 (BW-VGG19) architecture. In this method, a pre-trained VGG19 is fine-tuned with CNN architecture in the block-wise mechanism to enhance the system`s accuracy. The publicly accessible Contrast-Enhanced Magnetic Resonance Imaging (CE-MRI) dataset collected from 2005 to 2020 from different hospitals in China has been used in this research. Our proposed method is simple and achieved an accuracy of 0.98%. We compare our technique results with the existing Convolutional Neural network (CNN), VGG16, and VGG19 approaches. The results indicate that our proposed technique outperforms the best results associated with the existing methods.Keywords
Cite This Article
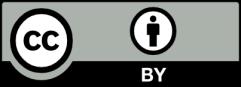
This work is licensed under a Creative Commons Attribution 4.0 International License , which permits unrestricted use, distribution, and reproduction in any medium, provided the original work is properly cited.