Topic Menu
► Topic MenuTopic Editors
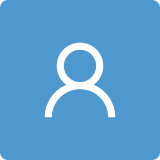
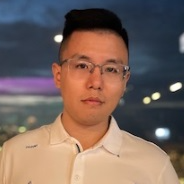
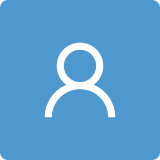
Geocomputation and Artificial Intelligence for Mapping
Topic Information
Dear Colleagues,
In the era of big data, the emergence of massive data creates opportunities and challenges to mapping. With the rapid development of Geocomputation and AI, RS mapping theory, deep learning models, and programming frameworks contribute to the intellectual development of research in geospatial-related mapping. In particular, the introduction of deep learning models and frameworks has greatly improved the accuracy and efficiency of geospatial-related mapping. However, new technology creates new opportunities as well as new challenges. As such, why are Geocomputation and artificial intelligence needed for mapping? Can specific problems be better solved using artificial intelligence techniques than traditional methods? Why does cartography need artificial intelligence, and how can artificial intelligence technology be used to improve the speed and accuracy of RS mapping? What new directions can we expect AI techniques to introduce to the broader fields of mapping and cartographic generalization?
The aim of this Topic is to provide the opportunity to explore the mentioned challenges in remote sensing mapping using computer vision, deep learning, and artificial intelligence. Topics may cover but are not limited to the following: object detection, change detection, map styles transferring, automated workflow of map generalization and mapping, etc.
- Mapping object information extraction from remote sensing and street view imagery;
- Automatic extraction of map symbols and text annotations on maps and imagery;
- Change detection and mapping based on artificial intelligence;
- Artificial intelligence for RS Mapping;
- Object recognition through artificial intelligence techniques;
- Cartographic relief shading with neural networks;
- Map style transferring using generative adversarial networks;
- Integration of artificial intelligence and map design;
- Automated workflow of cartographic generalization;
- Spatial explicit neural networks for GeoAI applications;
- AI mapping of urban socioeconomic patterns;
- Intelligent spatial analytics for earth process modeling and RS mapping.
Dr. Lili Jiang
Dr. Di Zhu
Dr. An Zhang
Topic Editors
Keywords
- artificial intelligence
- deep learning
- AI for mapping
- map styles transferring
- spatial patterns
- GeoAI
- map design
- remote sensing mapping
- object detection
Participating Journals
Journal Name | Impact Factor | CiteScore | Launched Year | First Decision (median) | APC |
---|---|---|---|---|---|
![]()
Geomatics
|
- | - | 2021 | 22.1 Days | CHF 1000 |
![]()
ISPRS International Journal of Geo-Information
|
2.8 | 6.9 | 2012 | 35.8 Days | CHF 1900 |
![]()
Remote Sensing
|
4.2 | 8.3 | 2009 | 23.9 Days | CHF 2700 |
MDPI Topics is cooperating with Preprints.org and has built a direct connection between MDPI journals and Preprints.org. Authors are encouraged to enjoy the benefits by posting a preprint at Preprints.org prior to publication:
- Immediately share your ideas ahead of publication and establish your research priority;
- Protect your idea from being stolen with this time-stamped preprint article;
- Enhance the exposure and impact of your research;
- Receive feedback from your peers in advance;
- Have it indexed in Web of Science (Preprint Citation Index), Google Scholar, Crossref, SHARE, PrePubMed, Scilit and Europe PMC.