Introduction
Regardless of whether one subscribes to the view that ageing is a collection of diseases or whether one sees the ageing process as distinct from the diseases arising in later life, there can be little argument that it is the diseases associated with growing old that present the greatest challenge for 21st-century medicine and are a growing burden on the economy.
To date, multiple theories of ageing have enhanced our understanding. But none have managed to provide complete explanations for the cause of age-related diseases that are backed up by specific disease examples. Consequently, distinctive features of ageing (as typified by the SENS or “Hallmarks of Ageing” typology) are relied upon as linchpins to guide study of the ageing process. And these are viewed as producing chaotic and intractable changes which are often, but not always, classified as “age-related diseases”. This disjunction is often captured by labelling age-related disease aetiologies as “unknown” or illustrating the relationship between ageing and age-related disease with the familiar “black box”. This is a vital gap, which this paper aims to fill.
New theory. In this paper, we propose a radically new explanation of ageing and related disease, bridging multiple scientific fields, including evolutionary biology and medicine. We also take account of specific biological mechanisms (genetics, molecular, cellular, physiological) involved. Supported by multiple specific disease examples, we demonstrate that the primary driver of ageing is the futile triggering of certain molecular pathways later in life that we term
patho-pathways (pathological pathways; for a glossary see
Table 1). Patho-pathways cause pathology via inducing cell, tissue and system
hyperactivity (
Figure 1a). Patho-pathways arise from constraints associated with the interconnected nature of multiple interacting molecular pathways within organisms that guide overall signalling networks, what we term
network constraint. More specifically, network constraint arises where optimisation of function (at the molecular, cell, tissue and system level) in all contexts is not possible, risking off-target side effects in the form of pathology when a “trigger” is present. Triggers can include extrinsic (e.g. infection, mechanical injury and molecular damage) and intrinsic (somatic mutations) factors, which converge to give rise to multifactorial aetiology. Some triggers act directly, while others act as primers to increase susceptibility to other triggers. Notably, triggers may have an evolutionary origin, and as such induce pathology at only a later time point later in the life history. Notably, in a domino style effect, patho-pathways can trigger the futile activation of other patho-pathways, resulting in complex cascades of patho-pathways (
Figure 1b). Significantly, these cascades are tractable. In this paper we classify and map these patho-pathway cascades to create
Blueprint Maps that explain the aetiology of multiple diseases, which in turn has utility in improving disease prediction means, and prediction and prevention therapeutics.
New Theory: Triggered Patho-Pathways
For ease in introducing our new theory, we have tied it to a specific example. In the prostate gland, long-term exposure to normal levels of dihydrotestosterone (DHT) promotes benign prostatic hyperplasia (Nacusi and Tindall, 2011; Waters et al., 2000) and increases the risk of prostate cancer (Untergasser et al., 2005). But what is the exact aetiology of the disease? Clearly here there is some link between pathology (hyperplasia) and long-term stimulus of DHT but what is the nexus of cause and effect? Is it merely the very slow cumulative action of DHT throughout life that drives pathology either directly or indirectly via random damage accumulation? If our hypothesis is correct, and the key driver of pathology is the induction of patho-pathways, i.e. molecular pathways initiated in the wrong place and/or at the wrong time, we need to be able to identify the “trigger”. In other words, something needs to change in the ageing prostate, leading to a novel pathogenic response to DHT. Indeed a trigger does exist: increased local, late-life inflammation disrupts the balance between cell proliferation and cell death, such that patho-pathways of proliferation predominate to induce hyperplasia (Tong and Zhou, 2020). Notably, here the DHT-driven patho-pathways are triggered during adulthood rather than simply being something present all along.
In principle, multiple causes could trigger patho-pathways, both intrinsic and extrinsic factors (e.g. infection, somatic mutation) (
Figure 1a). Patho-pathway triggers can also originate within the affected tissue, as in the case of prostrate hyperplasia, or beyond. An example of the latter is endometrial hyperplasia. This condition is a precursor to endometrial carcinoma, one of the most common gynaecological malignancies (Hannemann et al., 2007). The hyperplasia is caused by chronic oestrogen stimulation of endometrial cell growth, unopposed by progesterone that would otherwise cause cell shedding. Hyperplasia is triggered by factors that increase circulating oestrogen relative to progesterone, including high BMI, late age at menopause and nulliparity (Epplein et al., 2008; Hannemann et al., 2007) (
Figure 1c).
Patho-Pathways Induce Pathology through Cell, Tissue and System Hyperactivity
In the two examples of patho-pathways above, pathology has resulted from normal pathways having been initiated to a higher than normal level in a futile manner. In other words, biological function has been increased above the level of what is optimal to maintain healthy physiology. [Note here the definition of the word “function”, which is an activity that is natural to or the purpose of a thing]. But this is not the only way in which age-related disease presents, nor is it the only means through which patho-pathways can induce pathology. Patho-pathways frequently lead to an alteration in biological activity, which includes, not just increased function, but also cells taking on new unwanted activities. This can be as extreme as a change in cellular identity. As such, triggered patho-pathways can explain diseases involving hyperactivity commonly seen during ageing, including pathological cellular trans-differentiation. One example of this is vascular calcification, where active extraskeletal ossification (bone formation) takes place with age as vascular smooth muscle transdifferentiate into pseudo-osteoblasts (Virchow, 1863), and this is triggered by high blood calcium, phosphate and glucose levels and proinflammatory factors that initiate osteogenic patho-pathways (Abedin et al., 2004; Chen and Moe, 2012; Demer, 2002). As another example, mature medial smooth muscle cells undergoing clonal expansion can pathologically transdifferentiate into macrophage-like cells causing inflammation and atherosclerotic lesions, and this is triggered by patho-pathways induced by changes regulated at the mRNA level (Feil et al., 2014; Rong et al., 2003).
Existing Theories of Ageing in the Context of Our New Theory: The Role of Nutrient Signalling in Ageing
Existing theories. Many existing theories of ageing emphasise the nutrient signalling network, including the mTOR, IIS, PI3K-AKT and the Ras-MEK-ERK pathways, as a key proximate driver of the ageing process. One view is that ageing is driven by the futile continuation (or “run-on”) of growth and developmental programmes associated with nutrient signalling into late adulthood (like a tap that has been opened and not switched off), i.e. the Developmental/Hyperfunction Theory (Blagosklonny, 2006; de Magalhães, 2005; Gems, 2022; Maklakov and Chapman, 2019). When seeking specific disease examples, proponents of this theory tend to lean heavily on presbyopia (long-sightedness with age). Presbyopia results in part from the very gradual, continued growth of the eye lens during adulthood, which increases lens thickness thereby impairing ocular function (Blagosklonny, 2012; de Magalhães and Church, 2005; Gems, 2021; Strenk et al., 2005). Presbyopia is presumably alluded to so frequently because it is easy-to-grasp. But to date we have found no other clear examples cited concerning run-on in humans. An alternative view is that nutrient signalling is an “antagonistic hallmark”, whereby damage accumulation occurs throughout life, and increase in nutrient signalling is a response to this damage that initially mitigates the damage, but eventually, if chronic or exacerbated, becomes deleterious and contributes to ageing (López-Otín et al., 2023). Both these views stem from the observation that across laboratory models, inhibition of nutrient signalling via genetic mutations or interventions such caloric/dietary restriction results in robust lifespan extension. We recently provided evidence, however, that the mechanisms of ageing limiting lifespan need not be the same across species. And mechanisms associated with a corresponding very large magnitude increases in lifespan following suppression of nutrient signalling, such as the 10-fold lifespan extension seen in the common ageing model Caenorhabditis elegans, are unlikely to be influencing human ageing (Kern and Gems, 2022; Kern et al., 2023; Kern et al., 2021).
Our new theory. Our new theory predicts that nutrient signalling is not an immediate driver of ageing, and instead is only a secondary mediator of triggered patho-pathways. Let us look at mTOR function in specific disease examples to illustrate this. In all the examples of age-related disease described above and in
Table 2, mTOR is not the key driver of the disease. As an example, in endometrial hyperplasia, the immediate cause is an increase in the ratio of oestrogen to progesterone. Yes, mTOR
excess function is a critical factor for such hyperplasia, but one that is downstream in the chain of events leading to pathology. To emphasise just how “downstream” the role of mTOR is, one can look at what happens when conversely there is too much progesterone vs oestrogen, as with the progesterone only birth control pill: atrophy of the endometrium. As other examples, in prostrate hyperplasia immediate drivers of pathology are DHT and inflammation. In the progression of fatty liver (see
Table 2), immediate drivers are insulin resistance and lipotoxicity. In osteoporosis, immediate drivers are disrupted signalling cues to osteoclasts, like disrupted oestrogen levels. In diabetic cataracts (see
Table 2) the immediate driver is too much glucose along with constraints on enzymatic conversion rates of its by-product sorbitol. In these examples, mTOR is a player in the development of patho-pathways. Yet mTOR has little to do with the initial cause of the patho-pathways – mTOR is merely one mediator of upstream triggers. Thus, nutrient signalling activity in late life, such as mTOR activity, is a secondary cause of age-related disease. In terms of testable predictions associated with this, in contrast to previous theories, our new framework predicts that nutrient signalling hyperactivity in ageing will not occur gradually throughout life but instead will occur in mid to late life. And nutrient signalling hyperactivity will typically originate from triggers outside the cells being affected, rather than from triggers originating within the affected cells (i.e. hyperactivity will typically originate cell non-autonomously, rather than cell autonomously).
Evolutionary Theory in the Context of Our New Theory
A biomedical lens is not the only one through which age-related disease aetiology can be perceived. Theories that explain why ageing exists at the evolutionary level can also provide insights. The theory of Antagonistic Pleiotropy (AP) argues ageing is the consequence of a subset of wild-type genes that are pleiotropic, i.e. they influence two or more traits. These traits may have opposing (i.e. antagonistic) effects, such that their presence is associated with a trade-off. The combined costs of these trade-offs is “ageing” (Williams, 1957). AP genes associated with trade-offs where the cost appears later in life are more likely to be selected for during the course of evolution. This is because evolution works on the basis of survival of the fittest, and an earlier acting trait is more likely to have a larger impact on fitness (i.e. the ability of one organism to outcompete the other via reproductive success). Hence ageing (from trade-off costs) appears later in life. Data consistent with AP has been produced through a variety of studies such as laboratory artificial selection experiments, where animals (e.g. fruit fly Drosophila) when selected for enhanced lifespan, presented with reduced early life reproduction and vice versa (Stearns et al., 2001).
The concept of triggered patho-pathways is consistent with the theory of AP. Normal functioning of molecular pathways early in life is the benefit – the trade-off cost is the patho-pathway variants.
Why are patho-pathways so common such that they give rise to much of deleterious age-related disease, as in the examples above? What is the nature of the trade-off between beneficial molecular pathways vs their patho-pathway variants, such that benefit could not be uncoupled from cost over the course of evolution? The likely answer is that patho-pathways arise from an inability to optimise the function of all molecular pathway networks (at the molecular, cell, tissue and system level) across an organism in all contexts (cell and tissue types, genotypes, phenotypes and environmental conditions) – we term this
network constraint. Network constraint translates to a risk of off-target side effects in the form of pathology when an additional “trigger” is present (
Figure 4).
While in line with AP, the new concept of triggered patho-pathways requires an adjustment to the evolutionary theory. It is not simply a subset of genes that are AP genes, rather any and all genes in the human body (and their corresponding proteins and associated molecules) have the potential to be triggered (/activated) in a futile manner, and so incur cost in the form of patho-pathways. In other words, AP is feature of
all wild-type genes and corresponding molecules. As an example, consider transformed breast epithelial tissues in metastatic breast cancer: here all the genes and molecules supporting cellular function have become pathogenic - all exhibit AP (
Figure 4).
Whilst AP is feature of
all wild-type genes and molecules, all do not pose equal risk of generating age-related pathology. There are two key determinants of risk. First, presence of an appropriate trigger, such that hyperactivity arises. Second, the cost to physiology following the gene/molecule being triggered. As such, we suggest a simple risk equation as follows
Equation 1. Calculating risk of pathology from futile triggering of wild-type genes and molecules as part of patho-pathways. Risk of pathology (Rp) is a function of the cost incurred when the gene/molecule is triggered in error (Ct), and the likelihood of the gene/molecule being triggered in error, i.e. the probability of the cost (pCt). Genes/molecules associated with regulatory and signalling proteins like mTOR and IIS are at high risk of contributing to pathology. If triggered in a futile manner, such genes/molecules will have multiple knock on effects, making Ct high. And, as such genes/molecules typically have multiple upstream mediators, pCt is also high.
where Rp is risk of pathology, Ct is cost incurred when the gene is triggered in error and pCt the probability of the cost, which in turn is dependent on the probability of the trigger. Ct is predicted to be higher when a given gene/molecule plays a role in many different contexts (responding to environment, regulating reproductive status etc). An example of this is regulatory and signalling proteins, like the oestrogen receptor, that may serve multiple functions which, similar to building the circuitry of a machine or software coding, are at higher risk of signal cross-talk. Given triggered patho-pathways arise from trade-offs, trade-off functions are another useful means of mathematical representation. And to put the above another way, if one were to plot level of cost on the y axis and benefit on the x axis, network constraint would determine the shape of the trade-off function. Ecology, by determining fitness strategy, will determine trade-off intercept (and the presence of the trigger(s) determines when cost is incurred).
Blueprint Maps of Triggered Patho-Pathways in Wider Aetiological Webs
Complex though they are, triggered patho-pathway cascades represent small parts of much wider webs of disease aetiology. The highly complex and interconnected nature of late-life disease can present ageing as a hopelessly intractable condition. Yet, in principle, it ought to be possible to map out the interconnected webs of causation, thereby creating a
Blueprint Map. For an example of a Blueprint Map see case study 1 and
Figure 1b.
Case Study 1: A Blueprint Map of T2D and periodontitis |
As an illustration of a triggered patho-pathway aetiological web, consider the relationship between type II diabetes (T2D) and periodontitis, where working backwards we find both can stem from menopause-related endocrine changes in women >50 yrs.
T2D can suppress the immune system via several different discrete stage patho-pathway cascades. This suppression of the immune system by T2D goes on to risk infection such as periodontitis (severe gum infection that can destroy teeth and the bone that supports them). As an example, hyperglycaemia due to T2D leads to formation of advanced glycation end-products (AGEs). And this in turn induces patho-pathways in macrophages, causing a shift of class frequency. Numbers of M1 macrophages that encourage inflammation are reduced, and numbers of M2 macrophages that favour tissue repair are increased (He et al., 2020). This leads to a reduction in microbicidal capacity (Berbudi et al., 2020; Liu et al., 2012; Pavlou et al., 2018) which, along with reduced blood flow, increases risk of infection and impairs wound healing. Reduction in blood flow results from several causes, including increased blood viscosity from the hyperglycaemia and change in arterial wall tension from AGEs and triggered patho-pathway inflammation that causes vascular endothelial cell dysfunction (Cinar et al., 2001; Hadi and Suwaidi, 2007).
To illustrate how complex the picture is, infection risk from T2D-associated immune suppression in the mouth is further compounded by hyposalivation (possibly due to T2D associated impaired blood flow to the salivary glands) and an increase in salivary glucose levels (resulting from elevated plasma glucose) which promotes bacterial growth (Al-Maskari et al., 2011; Pérez-Ros et al., 2021). Hyposalivation contributes to infection risk as saliva contains antimicrobial proteins and peptides, and acts as a mechanical barrier preventing adhesion of microbes to the surface of the oral mucosa. Moreover, a lack of salvia production causes stagnation in flow; any stagnation in flow increases risk of infection via bacterial build-up from a lack of mechanical washing (Iwabuchi et al., 2012). Bacterial build-up both directly destroys tissue, and has indirect effects such as lodging in between the gum and enamel thereby reducing adherence of gum to the enamel (leading to gum recession).
When tracing out the cascades, what becomes apparent is that risk of periodontitis must be especially high in post-menopausal women. This is because the menopause increases risk of T2D as well as can directly contribute to periodontitis.
Regarding menopause and its relation to T2D, we find that endocrine changes during menopause initiate patho-pathways of insulin resistance that closely mimics the cascades of insulin resistance seen in more general inflammaging (Table 2, Figure 3b). Particularly oestrogen is known to play a role in regulating insulin sensitivity and glucose metabolism, and so decline in oestrogen causes elevated blood sugar levels that give rise to T2D (Ou et al., 2023). Specifically, oestrogen decline causes patho-pathways associated with a decline in pancreatic insulin production (Godsland, 2005), pulling glucose out of stores like muscle and the adipose tissue. Simultaneously, low oestrogen triggers patho-pathways of liver gluconeogenesis while decreasing glucose catabolism (Shen and Shi, 2015).
Regarding the menopause directly contributing to periodontitis, we find oestrogen deficiency induces triggered patho-pathways of bone resorption contributing to loss of bone that supports teeth, and oestrogen decline affects gingiva (gum) integrity (Bhardwaj and Bhardwaj, 2012; Kovacs, 2016). The latter arises from yet another triggered patho-pathway change: oestrogen controls proliferation and differentiation of keratinocytes and fibroblasts in the gingiva, with low oestrogen favouring cell shedding (Bhardwaj and Bhardwaj, 2012).
This complex example illustrates how diverging pathological outcomes from one condition in an aetiological web can reconverge to generate a further disease (here periodontitis). In other words, many diseases can converge to form one disease and one disease can proliferate to generate many more in a domino-style effect. |
Such Blueprint Maps are a means to go beyond studying
distinctive features, i.e. Hallmarks, of ageing, as they allow for hallmarks to be placed into the contexted of age-related disease. Hallmarks may act as causal drivers of a disease (acting as triggers of patho-pathways, being part of patho-pathways or being part of hyperactivity associated with pathopathways (see steps 1-4 in
Figure 5)). Hallmarks may also play more of a contributer role or play no causal role and merely be symptomatic outcomes that may or may not worsen disease outcome. Notably, different Hallmarks likely play different roles across differen age-related diseases. As specific examples, genomic instability (from replication errors or environmental insults) is a trigger in tumour and cancer formation, inducing pathopathways and hyperactivity associated with unregulated cell division and metastasis. Dysbiosis, via contributing to systemic inflammation, plays more of a trigger role in neurodegerative disease associated with neuroinflammation that contributes to Alzhmier’s and Parkinson’s disease. For instance, neuroinflamation via patho-pathways inducing microglia hyperactivation contribute to Alzhmier’s (
Table 2). Deregulated nutrient-signalling is often a mediator of patho-pathways as detailed earlier. Loss of proteostasis is an outcome of lipotoxicity, following patho-pathways of insulin resistance and associated hyperactivity in NAFLD (Baiceanu et al., 2016) (
Table 2).
Changing Clinical Practice and Scientific Approach: Triggered Patho-Pathway Cascades Are Amenable to Medical Intervention
The newly identified pathological principle of “triggered patho-pathways” serves as a tool to create Blueprint Maps in intricate detail. By understanding the aetiological webs that drive ageing, opportunities for interventions to improve health come into view. For example, using case study 1, we predict that hormone replacement therapy in post-menopausal women will be an effective treatment against periodontitis. And when searching the literature, this is supported by correlation data (Man et al.) As such, Blueprint Maps of triggered patho-pathways are a potential means for rational design of long-distance intervention approaches.
More broadly, there are three types of approaches one can take to utilise Blueprint Maps. (i) Identify and target the initiating triggers of patho-pathways, i.e. preventing a cause far upstream in the cascade of the immediate cause of a disease. This may be particularly effective where trigger amplification has occurred, as in the triggering of metabolic syndrome by inflammageing (
Table 2). (ii) Eliminate as many secondary systemic triggered patho-pathways as possible - given our new theory reveals much of age-related disease is a secondary consequence of initial triggers due to patho-pathway cascades that arise in a cell non-autonomous manner. One way to do this is via readjusting cell signalling cues in blood; this is likely why parabiosis (linking of the blood supplies) of young and old mice works to alleviate some age-related pathology - at least as far as clonal animals, like clonal laboratory mice, are concerned. Another, likely more practical, approach here is to use drugs like rapamycin (sirolimus) that target key mediators of signal transduction (i.e. target genes/molecules where the likelihood of pathology is high, i.e.
Ct is high in Equation 1). Such an approach can be likened to chemotherapy. Chemotherapy damages genes associated with cellular proliferation. The consequence is that highly proliferative cells, e.g. skin and hair, are most affected, while there is a smaller effect on more vital and slow dividing cells. General suppression of IIS/mTOR with a drug like rapamycin to treat ageing is likely to be beneficial in the same way - a balance will be struck between preventing gross pathological change by dampening all cellular function. But this will come with side effects like reduced immunosuppression and wound healing (from mTOR hypofunction). As a specific example, rapamycin dampens bone remodelling and so delays fracture healing (Holstein et al., 2008), but multiple papers also suggest it is beneficial against osteoporosis. In other words, beneficial effects will be highly disease-specific, and there is risk of off-target side effects. (iii) Find key downstream endpoints of multiple triggers and cascades to devise interventions that are effective across whole hosts of multifactorial diseases. Notably, for all of these, given Blueprint Maps enable bigger picture understanding of disease links, off-target side effects of therapeutics can be pre-empted and so avoided to a larger degree.
Predictions of the New Theory
An operative theory of ageing must be able to explain age-relate disease aetiology, as the new blueprint-theory does for many diseases. A further means to validate our new theory in this article is to see if it makes testable predictions, some of which may involve explaining past observations.
Table 3 contains a number of such predictions.
Conclusions
In this paper what is described is a new blueprint-style theory of ageing, spanning multiple scientific disciplines. Our theory is supported by specific disease examples and testable predictions. The theory demonstrates that the primary driver of much of age-related diseases is the futile triggering of specific molecular pathways in a pathological manner, which we term patho-pathways. Patho-pathways cause pathology via inducing cell, tissue and system hyperactivity (i.e. increase or change in normal biological activity). All wild-type (normal) genes and corresponding molecules have the potential to contribute to age-related diseases via patho-pathways. Specific risk of a wild-type gene or molecule giving rise to age-related disease can be formularised. In a domino-style effect, one patho-pathway typically triggers others, resulting in complex, but tractable, cascades. Significantly, mapping of these cascades is achievable, and the resulting Blueprint Maps enables identification of biomarkers and therapeutic targets far upstream in the causal chain of events. Thus enabling better predictions of disease occurrence far in advance, providing explanations for unexplained correlations between seemingly disparate diseases, and enabling development of more effective prevention and treatment measures, where off-target effects side effects are minimised.
References
- Abedin, M., Tintut, Y. and Demer, L.L., 2004. Vascular calcification: mechanisms and clinical ramifications. Arterioscler Thromb Vasc Biol. 24, 1161-70.
- Acerenza, L., 2016. Constraints, Trade-offs and the Currency of Fitness. J Mol Evol. 82, 117-27. [CrossRef]
- Adams, L.A., Angulo, P. and Lindor, K.D., 2005. Nonalcoholic fatty liver disease. Canad. Med. Assoc. J. 172, 899-905.
- Adinolfi, L.E., Rinaldi, L., Guerrera, B., Restivo, L., Marrone, A., Giordano, M. and Zampino, R., 2016. NAFLD and NASH in HCV Infection: Prevalence and Significance in Hepatic and Extrahepatic Manifestations. Int J Mol Sci. 17. [CrossRef]
- Admasu, T.D., Rae, M. and Stolzing, A., 2021. Dissecting primary and secondary senescence to enable new senotherapeutic strategies. Ageing Res Rev. 70, 101412. [CrossRef]
- Al-Maskari, A.Y., Al-Maskari, M.Y. and Al-Sudairy, S., 2011. Oral Manifestations and Complications of Diabetes Mellitus: A review. Sult. Qab. Uni. med. J. 11, 179-186.
- Baiceanu, A., Mesdom, P., Lagouge, M. and Foufelle, F., 2016. Endoplasmic reticulum proteostasis in hepatic steatosis. Nat Rev Endocrinol. 12, 710-722. [CrossRef]
- Berbudi, A., Rahmadika, N., Tjahjadi, A.I. and Ruslami, R., 2020. Type 2 diabetes and its impact on the immune system. Curr. Diabetes Rev. 16, 442-449. [CrossRef]
- Bhardwaj, A. and Bhardwaj, S.V., 2012. Effect of menopause on women's periodontium. J. Midliife Health. 3, 5-9. [CrossRef]
- Blagosklonny, M.V., 2006. Aging and immortality: quasi-programmed senescence and its pharmacologic inhibition. Cell Cycle. 5, 2087-102. [CrossRef]
- Blagosklonny, M.V., 2012. Answering the ultimate question "what is the proximal cause of aging?". Aging (Albany NY). 4, 861-877.
- Borash, D.J., Rose, M.R. and Mueller, L.D., 2007. Mutation accumulation affects male virility in Drosophila selected for later reproduction. Physiological and Biochemical Zoology. 80, 461-472. [CrossRef]
- Brown, R.J., Araujo-Vilar, D., Cheung, P.T., Dunger, D., Garg, A., Jack, M., Mungai, L., Oral, E.A., Patni, N., Rother, K.I., von Schnurbein, J., Sorkina, E., Stanley, T., Vigouroux, C., Wabitsch, M., Williams, R. and Yorifuji, T., 2016. The Diagnosis and Management of Lipodystrophy Syndromes: A Multi-Society Practice Guideline. The Journal of Clinical Endocrinology & Metabolism. 101, 4500-4511. [CrossRef]
- Carbone, M.A., Jordan, K.W., Lyman, R.F., Harbison, S.T., Leips, J., Morgan, T.J., DeLuca, M., Awadalla, P. and Mackay, T.F., 2006. Phenotypic variation and natural selection at Catsup, a pleiotropic quantitative trait gene in Drosophila. Current Biology. 16, 912-919. [CrossRef]
- Chang, L. and Heitkemper, M.M., 2002. Gender differences in irritable bowel syndrome. Gastroenterology. 123, 1686-1701. [CrossRef]
- Charlesworth, B., 2001. Patterns of age-specific means and genetic variances of mortality rates predicted by the mutation-accumulation theory of ageing. Journal of Theoretical Biology. 210, 47-65. [CrossRef]
- Chen, N.X. and Moe, S.M., 2012. Vascular calcification: pathophysiology and risk factors. Curr. Hypertens. Rep. 14, 228-237. [CrossRef]
- Chen, Z., Yu, R., Xiong, Y., Du, F. and Zhu, S., 2017. A vicious circle between insulin resistance and inflammation in nonalcoholic fatty liver disease. Lipids in Health and Disease. 16, 203. [CrossRef]
- Cinar, Y., Senyol, A.M. and Duman, K., 2001. Blood viscosity and blood pressure: role of temperature and hyperglycemia. Am J Hypertens. 14, 433-8. [CrossRef]
- Cooper, G.S., Bynum, M.L. and Somers, E.C., 2009. Recent insights in the epidemiology of autoimmune diseases: improved prevalence estimates and understanding of clustering of diseases. J Autoimmun. 33, 197-207. [CrossRef]
- Curtsinger, J.W. and Khazaeli, A.A., 2002. Lifespan, QTLs, age-specificity, and pleiotropy in Drosophila. Mechanisms of ageing and development. 123, 81-93.
- De Luca, M., Roshina, N.V., Geiger-Thornsberry, G.L., Lyman, R.F., Pasyukova, E.G. and Mackay, T.F., 2003. Dopa decarboxylase (Ddc) affects variation in Drosophila longevity. Nature genetics. 34, 429-433. [CrossRef]
- de Magalhães, J.P., 2005. Open-minded scepticism: inferring the causal mechanisms of human ageing from genetic perturbations. Ageing Res. Rev. 4, 1–22.
- de Magalhães, J.P. and Church, G.M., 2005. Genomes optimize reproduction: aging as a consequence of the developmental program. Physiology. 20, 252–259.
- Demaria, M., Ohtani, N., Youssef, S., Rodier, F., Toussaint, W., Mitchell, J., Laberge, R., Vijg, J., Van Steeg, H., Dollé, M., Hoeijmakers, J., de Bruin, A., Hara, E. and Campisi, J., 2014. An essential role for senescent cells in optimal wound healing through secretion of PDGF-AA. Dev Cell. 31, 722-733. [CrossRef]
- Demer, L.L., 2002. Vascular calcification and osteoporosis: inflammatory responses to oxidized lipids. International Journal of Epidemiology. 31, 737-741. [CrossRef]
- Distler, J.H.W., Wenger, R.H., Gassmann, M., Kurowska, M., Hirth, A., Gay, S. and Distler, O., 2004. Physiologic responses to hypoxia and implications for hypoxia-inducible factors in the pathogenesis of rheumatoid arthritis. Arthritis & Rheumatism. 50, 10-23. [CrossRef]
- Dørum, A., Tonstad, S., Liavaag, A.H., Michelsen, T.M., Hildrum, B. and Dahl, A.A., 2008. Bilateral oophorectomy before 50 years of age is significantly associated with the metabolic syndrome and Framingham risk score: a controlled, population-based study (HUNT-2). Gynecol Oncol. 109, 377-83. [CrossRef]
- Epplein, M., Reed, S.D., Voigt, L.F., Newton, K.M., Holt, V.L. and Weiss, N.S., 2008. Risk of Complex and Atypical Endometrial Hyperplasia in Relation to Anthropometric Measures and Reproductive History. Am. J Epidem. 168, 563-570. [CrossRef]
- Escobar, J.S., Jarne, P., Charmantier, A. and David, P., 2008. Outbreeding alleviates senescence in hermaphroditic snails as expected from the mutation-accumulation theory. Current Biology. 18, 906-910. [CrossRef]
- Everman, E.R. and Morgan, T.J., 2018. Antagonistic pleiotropy and mutation accumulation contribute to age-related decline in stress response. Evolution. 72, 303-317. [CrossRef]
- Fang, Y.P., Zhao, Y., Huang, J.Y., Yang, X., Liu, Y. and Zhang, X.L., 2024. The functional role of cellular senescence during vascular calcification in chronic kidney disease. Front Endocrinol (Lausanne). 15, 1330942. [CrossRef]
- Feil, S., Fehrenbacher, B., Lukowski, R., Essmann, F., Schulze-Osthoff, K., Schaller, M. and Feil, R., 2014. Transdifferentiation of Vascular Smooth Muscle Cells to Macrophage-Like Cells During Atherogenesis. Circulation Research. 115, 662-667. [CrossRef]
- Gems, D., 2021. Understanding hyperfunction: an emerging paradigm for the biology of aging. Preprints. [CrossRef]
- Gems, D., 2022. The hyperfunction theory: an emerging paradigm for the biology of aging. Ageing. Res. Rev. 74, 101557. [CrossRef]
- Giles, J.T., Szklo, M., Post, W., Petri, M., Blumenthal, R.S., Lam, G., Gelber, A.C., Detrano, R., Scott, W.W., Kronmal, R.A. and Bathon, J.M., 2009. Coronary arterial calcification in rheumatoid arthritis: comparison with the Multi-Ethnic Study of Atherosclerosis. Arthritis Research & Therapy. 11, R36. [CrossRef]
- Glyn-Jones, S., Palmer, A.J., Agricola, R., Price, A.J., Vincent, T.L., Weinans, H. and Carr, A.J., 2015. Osteoarthritis. Lancet. 386, 376-387.
- Godsland, I.F., 2005. Oestrogens and insulin secretion. Diabetologia. 48, 2213-2220. [CrossRef]
- Gong, Y., Thompson Jr, J.N. and Woodruff, R., 2006. Effect of deleterious mutations on life span in Drosophila melanogaster. The Journals of Gerontology Series A: Biological Sciences and Medical Sciences. 61, 1246-1252.
- Hadi, H.A.R. and Suwaidi, J.A., 2007. Endothelial dysfunction in diabetes mellitus. Vasc. Health Risk Manag. 3, 853-876.
- Hamilton, W.D., 1966. The moulding of senescence by natural selection. J. Theor. Biol. 12, 12-45. [CrossRef]
- Hannemann, M.M., Alexander, H.M., Cope, N.J. and Acheson, N., 2007. Endometrial hyperplasia: a clinician's review. Obst. Gyn. & Reproduct. Med. 17, 169-172.
- He, S., Hu, Q., Xu, X., Niu, Y., Chen, Y., Lu, Y., Su, Q. and Qin, L., 2020. Advanced glycation end products enhance M1 macrophage polarization by activating the MAPK pathway. Biochem Biophys Res Commun. 525, 334-340. [CrossRef]
- Heppner, F.L., Ransohoff, R.M. and Becher, B., 2015. Immune attack: the role of inflammation in Alzheimer disease. Nature Reviews Neuroscience. 16, 358-372. [CrossRef]
- Hibler, E.A., Kauderer, J., Greene, M.H., Rodriguez, G.C. and Alberts, D.S., 2016. Bone loss after oophorectomy among high-risk women: an NRG oncology/gynecologic oncology group study. Menopause (New York, N.Y.). 23, 1228-1232. [CrossRef]
- Holstein, J.H., Klein, M., Garcia, P., Histing, T., Culemann, U., Pizanis, A., Laschke, M.W., Scheuer, C., Meier, C., Schorr, H., Pohlemann, T. and Menger, M.D., 2008. Rapamycin affects early fracture healing in mice. British journal of pharmacology. 154, 1055-1062. [CrossRef]
- Hughes, K.A., Alipaz, J.A., Drnevich, J.M. and Reynolds, R.M., 2002. A test of evolutionary theories of aging. Proceedings of the National Academy of Sciences. 99, 14286-14291.
- Ising, C., Venegas, C., Zhang, S., Scheiblich, H., Schmidt, S.V., Vieira-Saecker, A., Schwartz, S., Albasset, S., McManus, R.M. and Tejera, D., 2019. NLRP3 inflammasome activation drives tau pathology. Nature. 575, 669-673. [CrossRef]
- Iwabuchi, H., Fujibayashi, T., Yamane, G., Imai, H. and Nakao, H., 2012. Relationship between Hyposalivation and Acute Respiratory Infection in Dental Outpatients. Gerontology. 58, 205-211. [CrossRef]
- Jemal, A., Bray, F., Center, M.M., Ferlay, J., Ward, E. and Forman, D., 2011. Global cancer statistics. CA: a cancer journal for clinicians. 61, 69-90.
- Karavassilis, M.E. and Faragher, R., 2013. A relationship exists between replicative senescence and cardiovascular health. Longev Healthspan. 2, 3. [CrossRef]
- Karmakar, S., Kay, J. and Gravallese, E.M., 2010. Bone damage in rheumatoid arthritis: mechanistic insights and approaches to prevention. Rheum. Dis. Clin. North Am. 36, 385-404. [CrossRef]
- Keller, L., Reid, J. and Arcese, P., 2008. Testing evolutionary models of senescence in a natural population: age and inbreeding effects on fitness components in song sparrows. Proceedings of the Royal Society B: Biological Sciences. 275, 597-604. [CrossRef]
- Kelly, J.K., 1999. An experimental method for evaluating the contribution of deleterious mutations to quantitative trait variation. Genetics Research. 73, 263-273. [CrossRef]
- Kelly, J.K., 2003. Deleterious mutations and the genetic variance of male fitness components in Mimulus guttatus. Genetics. 164, 1071-1085. [CrossRef]
- Kelly, J.K., 2008. Testing the rare-alleles model of quantitative variation by artificial selection. Genetica. 132, 187-198. [CrossRef]
- Kern, C.C. and Gems, D., 2022. Semelparous Death as one Element of Iteroparous Aging Gone Large. Front Genet. 13, 880343. [CrossRef]
- Kern, C.C., Srivastava, S., Ezcurra, M., Hsiung, K.C., Hui, N., Townsend, S., Maczik, D., Zhang, B., Tse, V., Konstantellos, V., Bähler, J. and Gems, D., 2023. C. elegans ageing is accelerated by a self-destructive reproductive programme. Nature Communications. 14, 4381. [CrossRef]
- Kern, C.C., Townsend, S., Salzmann, A., Rendell, N., Taylor, G., Comisel, R.M., Foukas, L., Bähler, J. and Gems, D., 2021. C. elegans feed yolk to their young in a form of primitive lactation. Nature Communications. 12, 5801. [CrossRef]
- Kim, S.-E., Paik, H.Y., Yoon, H., Lee, J.E., Kim, N. and Sung, M.-K., 2015. Sex-and gender-specific disparities in colorectal cancer risk. WJG. 21, 5167.
- Knobe, K. and Berntorp, E., 2011. Haemophilia and joint disease: pathophysiology, evaluation, and management. J Comorb. 1, 51-59. [CrossRef]
- Kovacs, C.S., 2016. Maternal mineral and bone metabolism during pregnancy, lactation, and post-weaning recovery. Physiological reviews. [CrossRef]
- Leips, J., Gilligan, P. and Mackay, T.F., 2006. Quantitative trait loci with age-specific effects on fecundity in Drosophila melanogaster. Genetics. 172, 1595-1605. [CrossRef]
- Lesser, K.J., Paiusi, I.C. and Leips, J., 2006. Naturally occurring genetic variation in the age-specific immune response of Drosophila melanogaster. Aging cell. 5, 293-295. [CrossRef]
- Liu, H.F., Zhang, H.J., Hu, Q.X., Liu, X.Y., Wang, Z.Q., Fan, J.Y., Zhan, M. and Chen, F.L., 2012. Altered polarization, morphology, and impaired innate immunity germane to resident peritoneal macrophages in mice with long-term type 2 diabetes. J Biomed Biotechnol. 2012, 867023. [CrossRef]
- Livingston, R.S., Myles, M.H., Livingston, B.A., Criley, J.M. and Franklin, C.L., 2004. Sex influence on chronic intestinal inflammation in Helicobacter hepaticus-infected A/JCr mice. Comp Med. 54, 301-8.
- Long, E. and Zhang, J., 2019. Retesting the influences of mutation accumulation and antagonistic pleiotropy on human senescence and disease. Nat Ecol Evol. 3, 992-993. [CrossRef]
- López-Otín, C., Blasco, M.A., Partridge, L., Serrano, M. and Kroemer, G., 2023. Hallmarks of aging: An expanding universe. Cell. [CrossRef]
- Macdonald, S.J. and Long, A.D., 2007. Joint estimates of quantitative trait locus effect and frequency using synthetic recombinant populations of Drosophila melanogaster. Genetics. 176, 1261-1281. [CrossRef]
- Maklakov, A.A. and Chapman, T., 2019. Evolution of ageing as a tangle of trade-offs: energy versus function. Proc Biol Sci. 286, 20191604. [CrossRef]
- Man, Y., Zhang, C., Cheng, C., Yan, L., Zong, M. and Niu, F. Hormone replacement therapy and periodontitis progression in postmenopausal women: A prospective cohort study. Journal of Periodontal Research. n/a. [CrossRef]
- Martin, G., 1978. Genetic syndromes in man with potential relevance to the pathobiology of aging. Birth Defects Orig Artic Ser. 14, 5–39.
- McGee, J.S. and Huttenhower, C., 2021. Of Mice and Men and Women: Sexual Dimorphism of the Gut Microbiome. IJW Derma. [CrossRef]
- Medawar, P.B., 1952. An Unsolved Problem Of Biology, H.K. Lewis, London.
- Miller, R.A., Berger, S.B., Burke, D.T., Galecki, A., Garcia, G.G., Harper, J.M. and Sadighi Akha, A.A., 2005. T cells in aging mice: genetic, developmental, and biochemical analyses. Immunological reviews. 205, 94-103. [CrossRef]
- Nacusi, L.P. and Tindall, D.J., 2011. Targeting 5alpha-reductase for prostate cancer prevention and treatment. Nat Rev Urol. 8, 378-84. [CrossRef]
- NCI, 2023. Surveillance, Epidemiology, and End Results Program http://seer.cancer.gov/.
- Nuzhdin, S.V., Pasyukova, E.G., Dilda, C.L., Zeng, Z.-B. and Mackay, T.F., 1997. Sex-specific quantitative trait loci affecting longevity in Drosophila melanogaster. Proceedings of the national academy of Sciences. 94, 9734-9739. [CrossRef]
- Ou, Y.J., Lee, J.I., Huang, S.P., Chen, S.C., Geng, J.H. and Su, C.H., 2023. Association between Menopause, Postmenopausal Hormone Therapy and Metabolic Syndrome. J Clin Med. 12. [CrossRef]
- Pavlou, S., Lindsay, J., Ingram, R., Xu, H. and Chen, M., 2018. Sustained high glucose exposure sensitizes macrophage responses to cytokine stimuli but reduces their phagocytic activity. BMC Immunol. 19, 24. [CrossRef]
- Pérez-Ros, P., Navarro-Flores, E., Julián-Rochina, I., Martínez-Arnau, F.M. and Cauli, O., 2021. Changes in Salivary Amylase and Glucose in Diabetes: A Scoping Review. Diagnostics (Basel). 11. [CrossRef]
- Pollreisz, A. and Schmidt-Erfurth, U., 2010. Diabetic cataract-pathogenesis, epidemiology and treatment. J Ophthalmol. 2010, 608751.
- Pollycove, R., Naftolin, F. and Simon, J.A., 2011. The evolutionary origin and significance of menopause. Menopause. 18. [CrossRef]
- Prata, L.G.P.L., Ovsyannikova, I.G., Tchkonia, T. and Kirkland, J.L., 2018. Senescent cell clearance by the immune system: Emerging therapeutic opportunities. Seminars in immunology. 40, 101275-101275. [CrossRef]
- Regan, J., Khericha, M., Dobson, A., Bolukbasi, E., Rattanavirotkul, N. and Partridge, L., 2016. Sex difference in pathology of the ageing gut mediates the greater response of female lifespan to dietary restriction. Elife. 5, e10956.
- Reynolds, R.M., Temiyasathit, S., Reedy, M.M., Ruedi, E.A., Drnevich, J.M., Leips, J. and Hughes, K.A., 2007. Age specificity of inbreeding load in Drosophila melanogaster and implications for the evolution of late-life mortality plateaus. Genetics. 177, 587-595.
- Rong, J.X., Shapiro, M., Trogan, E. and Fisher, E.A., 2003. Transdifferentiation of mouse aortic smooth muscle cells to a macrophage-like state after cholesterol loading. Proc Natl Acad Sci U S A. 100, 13531-6. [CrossRef]
- Sala Frigerio, C., Wolfs, L., Fattorelli, N., Thrupp, N., Voytyuk, I., Schmidt, I., Mancuso, R., Chen, W., Woodbury, M. and Srivastava, G., 2019. The major risk factors for Alzheimer’s disease: age, sex, and genes modulate the microglia response to Aβ plaques. Cell Rep 27: 1293–1306. e6.
- Sapey, E., Greenwood, H., Walton, G., Mann, E., Love, A., Aaronson, N., Insall, R.H., Stockley, R.A. and Lord, J.M., 2014. Phosphoinositide 3-kinase inhibition restores neutrophil accuracy in the elderly: toward targeted treatments for immunosenescence. Blood. 123, 239-48. [CrossRef]
- Sears, B. and Perry, M., 2015. The role of fatty acids in insulin resistance. Lipids Health Dis. 14, 121-121. [CrossRef]
- Shen, M. and Shi, H., 2015. Sex Hormones and Their Receptors Regulate Liver Energy Homeostasis. International Journal of Endocrinology. 2015, 294278. [CrossRef]
- Stearns, S., Partridge, L., Masoro, E. and Austad, S., 2001. Handbook of the Biology of Aging.
- Strenk, S.A., Strenk, L.M. and Koretz, J.F., 2005. The mechanism of presbyopia. Prog. Retin. Eye Res. 24, 379-393. [CrossRef]
- Swindell, W.R. and Bouzat, J.L., 2006. Inbreeding depression and male survivorship in Drosophila: implications for senescence theory. Genetics. 172, 317-327. [CrossRef]
- Tong, Y. and Zhou, R.Y., 2020. Review of the Roles and Interaction of Androgen and Inflammation in Benign Prostatic Hyperplasia. Mediators Inflamm. 2020, 7958316. [CrossRef]
- Unger, R.H., 2003. Minireview: weapons of lean body mass destruction: the role of ectopic lipids in the metabolic syndrome. Endocrinology. 144, 5159-65. [CrossRef]
- Untergasser, G., Madersbacher, S. and Berger, P., 2005. Benign prostatic hyperplasia: age-related tissue-remodeling. Exp Gerontol. 40, 121-128. [CrossRef]
- van Deursen, J.M., 2014. The role of senescent cells in ageing. Nature. 509, 439-446.
- Virchow, R., 1863. Cellular Pathology, as Based upon Physiological and Pathological Histology, Dover Publications, Inc, New York, NY. [CrossRef]
- Voynow, J.A. and Shinbashi, M., 2021. Neutrophil Elastase and Chronic Lung Disease. Biomolecules. 11. [CrossRef]
- Wang, Q., Liu, Y. and Zhou, J., 2015. Neuroinflammation in Parkinson's disease and its potential as therapeutic target. Transl Neurodegener. 4, 19. [CrossRef]
- Waters, D.J., Shen, S. and Glickman, L.T., 2000. Life expectancy, antagonistic pleiotropy, and the testis of dogs and men. The Prostate. 43, 272-277. [CrossRef]
- Whiteside, S.K., Snook, J.P., Williams, M.A. and Weis, J.J., 2018. Bystander T Cells: A Balancing Act of Friends and Foes. Trends Immunol. 39, 1021-1035. [CrossRef]
- Williams, G.C., 1957. Pleiotropy, natural selection and the evolution of senescence. Evolution. 11, 398-411.
- Xie, J., Van Hoecke, L. and Vandenbroucke, R.E., 2021. The Impact of Systemic Inflammation on Alzheimer's Disease Pathology. Front Immunol. 12, 796867.
Figure 1.
Patho-pathways are triggered. a, Patho-pathways induce pathology via hyperactivity. Triggers of patho-pathways include environmental factors as well as genetic, accumulative and evolutionary. Patho-pathways are the futile activation of molecular pathways at the wrong time and/or wrong place. Hyperactivity is unwanted activity by cells, tissues and/or whole systems. b, Once triggered, one patho-pathway can trigger others, resulting in cascades of patho-pathways. Patho-pathways may directly induce pathology or converge to induce pathology. c, An example of a triggered patho-pathway. Listed triggers: elevate oestrogen to progesterone ratio causing endometrial hyperplasia, increasing risk of endometrial carcinoma.
Figure 1.
Patho-pathways are triggered. a, Patho-pathways induce pathology via hyperactivity. Triggers of patho-pathways include environmental factors as well as genetic, accumulative and evolutionary. Patho-pathways are the futile activation of molecular pathways at the wrong time and/or wrong place. Hyperactivity is unwanted activity by cells, tissues and/or whole systems. b, Once triggered, one patho-pathway can trigger others, resulting in cascades of patho-pathways. Patho-pathways may directly induce pathology or converge to induce pathology. c, An example of a triggered patho-pathway. Listed triggers: elevate oestrogen to progesterone ratio causing endometrial hyperplasia, increasing risk of endometrial carcinoma.
Figure 2.
Disease onset may be dependent on the convergence of multiple patho-pathway triggers. a, Some triggers may not directly induce pathology, and rather only prime cells, tissue and systems. Other triggers then act to kick-start destruction once the tissue is primed. As an analogy, for a fire you need both the spark, i.e. kick-starting trigger, and fuel, i.e. priming. b, Cascades of primary and secondary patho-pathways promote organismal ageing (hypothetical scheme). More severe patho-pathways are seen in later life due to the occurrence of triggers with an evolutionary origin at that time.
Figure 2.
Disease onset may be dependent on the convergence of multiple patho-pathway triggers. a, Some triggers may not directly induce pathology, and rather only prime cells, tissue and systems. Other triggers then act to kick-start destruction once the tissue is primed. As an analogy, for a fire you need both the spark, i.e. kick-starting trigger, and fuel, i.e. priming. b, Cascades of primary and secondary patho-pathways promote organismal ageing (hypothetical scheme). More severe patho-pathways are seen in later life due to the occurrence of triggers with an evolutionary origin at that time.
Figure 3.
Multi-stage and discrete stage patho-pathway cascades. a, b, Multi-stage patho-pathway cascades. a, Rheumatoid arthritis driven by patho-pathways of inflammation. b, Non-alcoholic fatty liver disease (NAFLD) driven by patho-pathways of insulin resistance. c, d, Discrete stage patho-pathway cascades. c, Calcium and phosphate movement into blood triggers patho-pathways of vascular transdifferentiation and ossification, which cause vascular calcification. d, Lipotoxicity in NAFLD increases susceptibility of the liver to injury and causes inflammation, which in turn triggers fibrosis patho-pathways and an exaggerated wound healing response. Specifically, proinflammatory cytokines induce patho-pathways of hyperactivity in innate immune cells which directly damage tissue. Concomitantly these cytokines trigger patho-pathways of hyperactivity in fibroblasts which causes the fibroblasts to secrete too much extracellular matrix including collagen. The resulting fibrosis contributes to liver failure via disruption of the liver architecture and function.
Figure 3.
Multi-stage and discrete stage patho-pathway cascades. a, b, Multi-stage patho-pathway cascades. a, Rheumatoid arthritis driven by patho-pathways of inflammation. b, Non-alcoholic fatty liver disease (NAFLD) driven by patho-pathways of insulin resistance. c, d, Discrete stage patho-pathway cascades. c, Calcium and phosphate movement into blood triggers patho-pathways of vascular transdifferentiation and ossification, which cause vascular calcification. d, Lipotoxicity in NAFLD increases susceptibility of the liver to injury and causes inflammation, which in turn triggers fibrosis patho-pathways and an exaggerated wound healing response. Specifically, proinflammatory cytokines induce patho-pathways of hyperactivity in innate immune cells which directly damage tissue. Concomitantly these cytokines trigger patho-pathways of hyperactivity in fibroblasts which causes the fibroblasts to secrete too much extracellular matrix including collagen. The resulting fibrosis contributes to liver failure via disruption of the liver architecture and function.

Figure 4.
A blueprint model for the causes of ageing and late-life disease (hypothetical scheme). a, Iustration of network constraint demonstrating how the presence of a trigger indues patho-pathways due to unavoidable interlinkages within and between molecular pathways. Blue: normal function. Red: pathological function. b, Overview of blueprint model working across scientific disciplines, from evolutionary biology through to medicine and including biological mechanisms (genetics, molecular, cellular, physiological). Patho-pathways are the futile activity (hyperactivity) of molecular pathways in the wrong time and/or place. The beneficial action of the same molecular pathways in their adapted and intended form (i.e. function in the right time and at the right place) cannot be uncoupled from their patho-pathway variants due to network constraint, underlying the basis of an AP trade-off. Patho-pathway hyperactivity causes hyperactivity at higher orders of biological organisations, e.g. cell, tissue, organ and system. At these higher levels of biological organisations, hyperactivity translates to pathology phenotypes in the form of hypertrophy, hypersecretion, dystrophy, dysplasia, transdifferentiation etc. And in turn this disrupts organ and system structure and function to cause disease.
Figure 4.
A blueprint model for the causes of ageing and late-life disease (hypothetical scheme). a, Iustration of network constraint demonstrating how the presence of a trigger indues patho-pathways due to unavoidable interlinkages within and between molecular pathways. Blue: normal function. Red: pathological function. b, Overview of blueprint model working across scientific disciplines, from evolutionary biology through to medicine and including biological mechanisms (genetics, molecular, cellular, physiological). Patho-pathways are the futile activity (hyperactivity) of molecular pathways in the wrong time and/or place. The beneficial action of the same molecular pathways in their adapted and intended form (i.e. function in the right time and at the right place) cannot be uncoupled from their patho-pathway variants due to network constraint, underlying the basis of an AP trade-off. Patho-pathway hyperactivity causes hyperactivity at higher orders of biological organisations, e.g. cell, tissue, organ and system. At these higher levels of biological organisations, hyperactivity translates to pathology phenotypes in the form of hypertrophy, hypersecretion, dystrophy, dysplasia, transdifferentiation etc. And in turn this disrupts organ and system structure and function to cause disease.
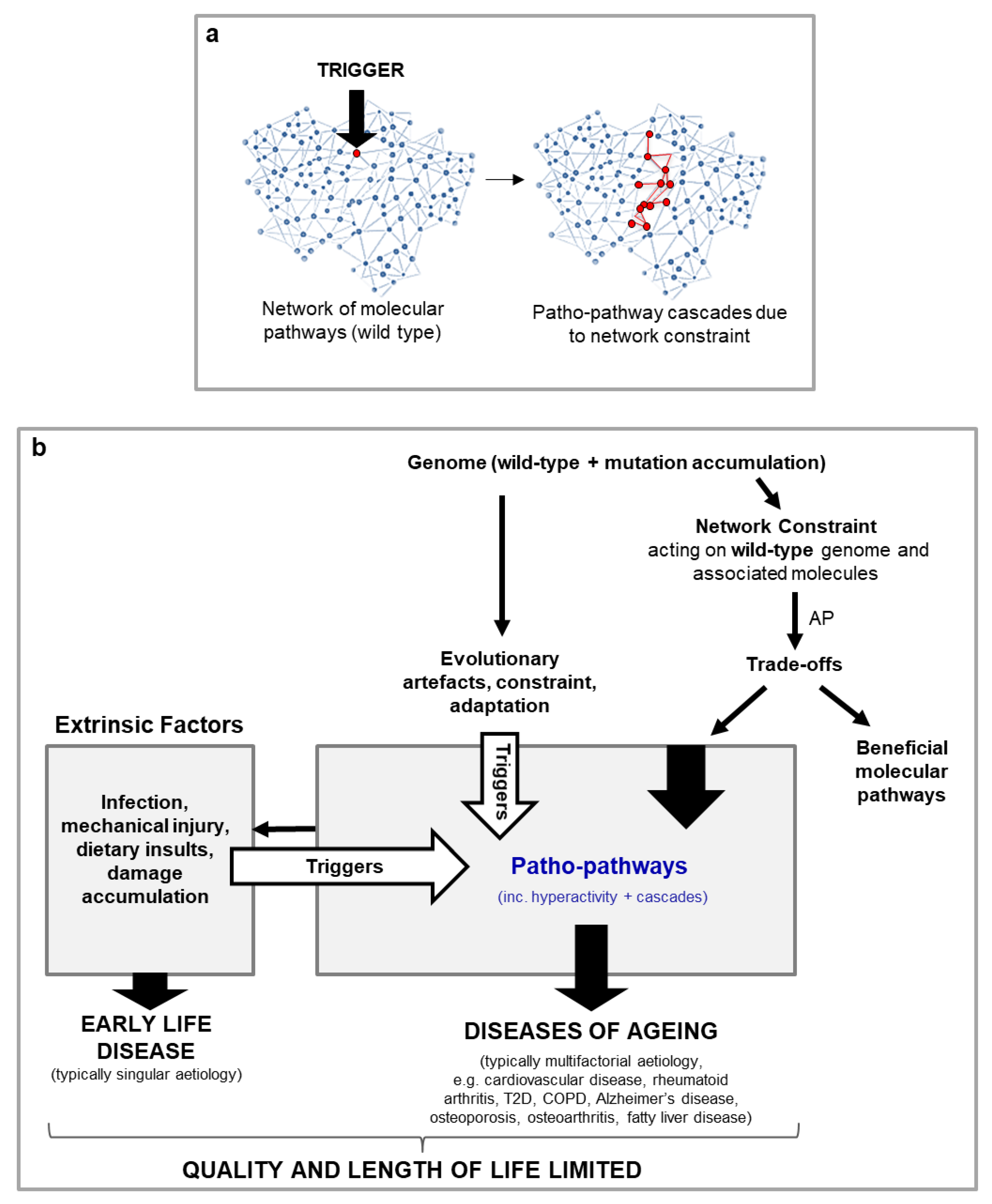
Figure 5.
Blueprint Maps allow for Hallmarks of ageing to be placed into the context of disease aetiology. Hallmarks may fall into any 1-4 steps dhown in blue, or overlap across steps. 12 recent Hallmarks of ageing shown from (López-Otín et al., 2023). Hallmarks may act as causal drivers of a disease, acting as triggers of patho-pathways, being part of patho-pathways or being part of hyperactivity associated with pathopathways. Hallmarks may also play more of a contributer role or play no causal role and merely be symptomatic outcomes that may or may not worsen disease outcome. Hallmarks may also play no role altogether. Different Hallmarks likely play different roles across differen age-related diseases.
Figure 5.
Blueprint Maps allow for Hallmarks of ageing to be placed into the context of disease aetiology. Hallmarks may fall into any 1-4 steps dhown in blue, or overlap across steps. 12 recent Hallmarks of ageing shown from (López-Otín et al., 2023). Hallmarks may act as causal drivers of a disease, acting as triggers of patho-pathways, being part of patho-pathways or being part of hyperactivity associated with pathopathways. Hallmarks may also play more of a contributer role or play no causal role and merely be symptomatic outcomes that may or may not worsen disease outcome. Hallmarks may also play no role altogether. Different Hallmarks likely play different roles across differen age-related diseases.
Table 1.
Glossary of key terms.
Table 1.
Glossary of key terms.
Antagonistic pleiotropy (AP) |
Where action of a given gene is both beneficial and detrimental to fitness. If the latter occurs later in life and is therefore subject to weaker selection, such a gene may be favoured by natural selection, and promote ageing (Williams, 1957). |
Biological constraint |
A property of organisms and/or their ecology that prevents the evolution of traits that would increase fitness. |
Discrete stage patho-pathway cascade (New term) |
A series of patho-pathways triggered in a causal chain by different, but linked, triggers (c.f. multi-stage patho-pathway cascade). |
Hyperactivity (New term) |
Where an excess of normal cell, tissue or system activity leads to age-related pathology. This includes, not only an increase in normal function, such that it is above optimal for health, but also cells and tissues taking on new, unwanted and pathological activities. |
Multi-stage patho-pathway cascade (New term) |
Where a single underlying trigger drives multiple patho-pathways in succession, such that multiple stages can be distinguished (c.f. discrete stage patho-pathway cascade). |
Network constraint (new term) |
Where a given molecule acts in diverse contexts (cell and tissue types, genotypes, phenotypes, and environmental conditions) across signalling networks within an organism, such that optimisation of function in all contexts is not possible. (This is a type of biological constraint, c.f. biological constraint). |
Patho-pathway cascade (New term) |
A causal chain of patho-pathways, in which each triggers the next in the chain (c.f. multi-stage patho-pathway cascade and discrete patho-pathway cascade). |
Wild-type |
Genes maintained in the population, i.e. non atypical mutant. |
Table 2.
Multi-stage vs discrete stage patho-pathway cascade examples.
Table 2.
Multi-stage vs discrete stage patho-pathway cascade examples.
Multi-Stage Patho-Pathway Cascade Example |
Discrete Stage Patho-Pathway Cascade Example |
Non-alcoholic fatty liver disease (NAFLD) Key trigger: insulin resistance
The progression of NAFLD entails the sequential action of insulin resistance-triggered patho-pathways (Figure 3b). A major initiator of these is inflammation in adipose tissue, typically a consequence of obesity and/or inflammaging. [Obesity, with fat accumulation causes adipocytes reach their limit of expansion. This induces hypoxia which triggers proinflammatory patho-pathways (Sears and Perry, 2015) that mimic effects of inflammaging]. Steps are as follows:
(i) Inflammation triggers patho-pathways of adipocyte insulin resistance. This causes increased export of free fatty acids (FFA) from the adipocytes and into the circulation (stage 1). (ii) FFA are then taken up by other organs that are not adapted to store large amounts of fat, such as the liver. This in turn leads to lipotoxicity (lipid-induced dysfunction) in those organs, which triggers localised patho-pathways of insulin resistance at those sites (stage 2) (Adams et al., 2005; Sears and Perry, 2015; Unger, 2003). It is here that the systemic consequences of insulin resistance really start to take effect, as hepatic insulin resistance and lipid accumulation trigger a patho-pathway positive feedback loop, driving the development of the metabolic syndrome (Figure 3b).
To compound these changes, insulin resistance impairs control of blood glucose levels, further triggering type II diabetes (T2D) (Adams et al., 2005; Chen et al., 2017; Unger, 2003). Specifically, in the liver insulin resistance associated patho-pathways causes excess glucose secretion into the blood, and insulin resistance patho-pathways in muscle reduces glucose uptake, which together pathologically increase blood glucose levels, to induce T2D.
In conclusion, Although NAFLD involves different organs (particularly adipose tissue and liver), the tight causal relationship between patho-pathway changes define a multi-stage patho-pathway cascade. |
Non-alcoholic steatohepatitis (NASH)
Key trigger: Lipotoxicity associatedinjury
Returning to NAFLD (Figure 3b), lipotoxicity increases the susceptibility of the liver to injury (i.e. primes the liver c.f. Figure 2a). As a discrete stage cascade, upon injury, severe inflammation and fibrosis patho-pathways are triggered as an exaggerated wound healing response. (Specifically, proinflammatory cytokines induce patho-pathways of hyperactivity in innate immune cells which directly damage tissue. Concomitantly these cytokines trigger patho-pathways of hyperactivity in fibroblasts which causes the fibroblasts to secrete too much extracellular matrix including collagen - the resulting fibrosis contributes to liver failure via disruption of the liver architecture and function) (Figure 3d).
Overall, this can result in disease progression to NASH, an aggressive form of fatty liver disease marked by liver inflammation, and advanced scarring and fibrosis (i.e. cirrhosis) (Adams et al., 2005). |
Neurodegeneration
Key trigger: inflammation
General systemic inflammation, from causes such as those that give rise to NAFLD (Figure 3b), can then go on to further trigger neurodegeneration via patho-pathways associated with microglia hyperactivity.
As a specific example, increasing evidence indicates that systemic inflammation might drive the initiation and progression of Alzheimer's disease as immune cues can pass into the brain through various routes (e.g. via the circumventricular organs, across the brain barriers, through activating vascular cells at the brain barriers and even neural routes like cytokines from the thoracic-abdominal cavity (e.g. Kupffer cells in the liver) directly activating the vagal nerve which signals to the brain (Xie et al., 2021)). Neuroinflammation in turn contributes to Alzheimer's disease (Heppner et al., 2015; Sala Frigerio et al., 2019) via microglia hyperactivation as it exacerbates amyloid beta and tau pathologies that in turn cause cognitive decline (Ising et al., 2019). Likewise Parkinson’s disease is associated with neuroinflammation that systemic inflammation contributes to (Wang et al., 2015). |
Diabetic cataracts
Key trigger: excessive glucose
Insulin resistance leading to T2D (Unger, 2003), can in turn cause diabetic cataracts as a part of a discrete stage patho-pathway cascade. Diabetic cataracts develop due to patho-pathways associated with excessive conversion of glucose into sorbitol, i.e. at a rate higher than sorbitol can be converted into fructose by the enzyme sorbitol dehydrogenase. The resulting build-up of sorbitol then generates increased osmotic stress in the lens fibres, causing them to swell and rupture (Pollreisz and Schmidt-Erfurth, 2010). |
Cellular Senescence accumulation
Key trigger: inflammation
Another example of a discrete-stage patho-pathway cascade involves immunosenescence and accumulation of cells that have undergone cellular senescence (cell cycle arrest, and entry into a hypertrophic, hypersecretory state) (Prata et al., 2018) due to insulin resistance.
Throughout life, fibroblasts undergo these type of differentiative changes to support wound healing (Demaria et al., 2014). Such “senescent” fibroblasts are subsequently cleared by the immune system, triggered by signals within the fibroblast senescence-associated secretory phenotype (SASP). Any factor that compromises efficiency of immune clearance of senescent fibroblasts, such as the insulin resistance that contributes to and aggravates systemic inflammation (see Figure 3b), results in path-pathway variants of this - and the accumulation of senescent fibroblasts that go on to disrupt cellular microenvironments which, in mice at least, promotes diverse diseases of ageing (van Deursen, 2014).
Secondary cellular senescence
Key trigger: autocrine and paracrine signals
In an additional discrete stage cascade, accumulating senescent cells can trigger patho-pathways in neighbouring cells that cause them to also undergo senescence (secondary senescence via autocrine and paracrine signals) (Admasu et al., 2021). |
Table 3.
Predictions of the new theory.
Table 3.
Predictions of the new theory.
Predictions of the New Theory |
Prediction |
Supporting or Falsifying Evidence |
Nutrient signalling pathway hyperactivity in-vivo will occur in mid to late life, rather than increasing gradually throughout life |
To be tested |
Nutrient signalling pathway hyperactivity will typically originate cell non-autonomously, rather than cell autonomously |
To be tested |
Ageing and age-related disease is not restricted to old ages – ageing can be “triggered” in the young
If age-related disease can indeed be “triggered”, age-related disease alongside their associated patho-pathway cascades, should be observable in the young |
Supporting evidence:
Numerous disorders exhibit features that resemble acceleration of some aspects of ageing, so-called progeroid conditions (Martin, 1978). And in many cases, this reflects earlier occurrence of triggered patho-pathway cascades. For example, oophorectomy (removal of the ovaries) prior to menopause leads to a sharp decline in circulating oestrogen which causes osteoporosis and metabolic syndrome (Dørum et al., 2008; Hibler et al., 2016).
Multiple progeroid congenital syndromes also entail earlier occurrence of triggered patho-pathway cascades. One example is lipodystrophy syndromes, such as Beradinelli-Seip syndrome, Ferreira-Marques lipoatrophia, Werner syndrome and Hutchinson-Gilford syndrome (HGS), which induce pathology via similar path-pathway cascades to those seen in inflammaging and obesity ( Figure 3b). This is because the adipose tissue’s capacity to store FFA is reduced in lipodystrophy syndromes, resulting in FFA movement into the circulation and subsequent chain of events that culminate in metabolic syndrome (Brown et al., 2016).
Bystander activation associated with infectious disease can induce similar patho-pathways as those seen with inflammaging. One example here is in Lyme disease, which results from infection with the tick-borne bacterium Borrelia burgdorferi. Here bystander T cell activation can induce patho-pathways in synoviocytes and osteoclasts mirroring the pathogenesis of rheumatoid arthritis (Whiteside et al., 2018) ( Figure 3a). Another example is Hepatitis C. Bystander activation after infection with the virus hepatitis C causes liver damage via triggered patho-pathways closely resembling that of NAFLD and NASH (Adinolfi et al., 2016)
|
Observation of age-related increases in genetic variance for fitness components and in inbreeding load
Genetic variance. Genetic variance is the difference in DNA sequences between individuals within a population. The more genes involved in generating ageing and related disease, the greater the likelihood of genetic variance being observed for fitness components.
Previous proximate mechanism models of AP assume ageing stems from a subset of AP genes. In contrast, triggered patho-pathways and associated cascades do not assume that a small number of AP genes control fitness changes at both early and late stages of life. Cascades arising in later life entail the futile, unregulated triggering of whole hosts of genes and activation of molecules in later life (and any and every gene has the potential to be a pathology inducing AP gene).
Inbreeding load. If only a subset of AP genes are driving ageing, an age-related increases in inbreeding load (the negative consequences of recessive allele build-up) is not expected. In our new theory, however, recessive alleles can act as any other extrinsic or extrinsic factor that triggers patho-pathways due to network constraint. This means that an age related increase in inbreeding load is expected. Additionally, we should be able to find specific medical examples of mutated alleles inducing disease via triggering patho-pathways. |
Supporting evidence: Observations of age-related change in genetic variance for fitness components and in inbreeding load has mostly been done in invertebrates such as Drosophila. The results show that genetic variation and inbreeding effects increase dramatically with age (Borash et al., 2007; Charlesworth, 2001; Escobar et al., 2008; Everman and Morgan, 2018; Gong et al., 2006; Hughes et al., 2002; Keller et al., 2008; Lesser et al., 2006; Reynolds et al., 2007; Swindell and Bouzat, 2006), in support of our new theory.
And there are specific medical examples of mutated alleles inducing disease via triggering patho-pathways. As one such example, the frequency of the mutated allele in haemophilia and so the incidence of the disorder, was greater among the royal families of Europe due to the high levels of royal inbreeding. Haemophilia causes recurring joint bleeding that triggers haemophilic arthropathy due to consequential joint inflammation. This joint inflammation initiates patho-pathways of synovial hypertrophy and other associated cascades as in Figure 3a, with haemophilic arthropathy closely resembling the pathological mechanisms seen in rheumatoid arthritis (Knobe and Berntorp, 2011).
|
Common versus rare alleles as drivers of ageing
Previous proximate mechanism models of AP assume ageing stems from a subset of AP genes. If true, genetic variation in ageing should be mainly due to alleles that segregate at intermediate frequencies.
This contrasts our new theory. Not just common alleles, but also rare alleles could be triggered late on as part of patho-pathway cascades and so contribute to ageing. Expression of rare alleles may also act as patho-pathway triggers directly or indirectly via their knock-on effects. If true, there should be medical examples of this.
|
Testing the prediction that genetic variation in ageing should be mainly due to alleles that segregate at intermediate frequencies has proved to be challenging, so there is no consensus (Carbone et al., 2006; De Luca et al., 2003; Kelly, 1999; Kelly, 2003; Kelly, 2008; Macdonald and Long, 2007).
But in terms of medical examples of rare alleles accelerating age-related disease, there are examples as in the case of Haemophilia above. |
Do similar or different genomic regions control variance at early and late ages?
Previous proximate mechanism models of AP assume that similar genomic regions will control variance in the phenotype at early and late ages, due to a small number of AP genes.
In contrast, late life action of triggered patho-pathways and cascades would mean that different genomic regions would, in fact, be predicted to contribute to variation at early and late ages. Presence of triggered patho-pathways means genes exerting effects at only very specific times during youth for fitness benefits may be more frequently activated in a futile manner late in life. For example, genes and molecules associated with an immune response will only be triggered when an infection is sensed in youth, but may be constantly expressed later in life as part of an autoimmune disease such as rheumatoid arthritis. |
Supporting evidence:
Results of quantitative trait locus (QTL) mapping studies in Drosophila and mice were consistent with our new theory (Curtsinger and Khazaeli, 2002; Leips et al., 2006; Miller et al., 2005; Nuzhdin et al., 1997).
There is also more recent work that is looking at using human genome wide association studies (GWAS) to test these predictions, with particular focus on long-term longitudinal studies, but sufficiently large data sets are required to attain reliable results here (Long and Zhang, 2019), which we currently lack. |
If network constraint is the reason behind why evolution is unable to uncouple trade-offs (i.e. separate cost from benefit) associated with patho-pathways, we should see evolutionary conservation of network constraint (i.e. all similar species should be subject to network constraint).
While this is difficult to test for directly, we should see indirect evidence in the form of conservation of triggered patho-pathways and cascades across species |
Supporting evidence:
This prediction is consistent with the presence of similar diseases of ageing in many mammalian species. As a specific example, canine rheumatoid arthritis shows patho-pathway cascades and stages that are very similar to those seen in human rheumatoid arthritis (Figure 3a) (Bennett, 1987; Innes & Clegg, 2010). |
If patho-pathways stem from network constraint, a prediction that follows is more broadly we should see a pattern where elements of biology associated with greater plasticity of function are expected to be at greater risk of inducing pathology (because they are subject to greater levels of network constraint). This prediction should hold true at all levels, from the molecular level up to cells and whole tissues and organs
An easy means to test this is at the tissue and organ level – are tissue types and organs that show greater plasticity of strategy (such as those associated with active tissue remodelling during adulthood, e.g. to respond to infection, or for reproduction) more likely to be associated with disease? |
Supporting evidence:
In line with this prediction, structurally more static tissues e.g. myocardial and neurological, are less prone to pathology (Cooper et al., 2009; NCI, 2023).
Sexual dimorphism in pathology presentation is also in line with this prediction. Typically in the more “plastic” sex, i.e. females that undergo greater change in tissue status for reproduction, show greater susceptibility to some diseases of ageing both in laboratory model animals and humans. One example of this is intestinal pathology, where the female intestine undergoes remodelling for reproduction and lactation and as a consequence tends to be more prone to patho-pathways and pathology. For example, in the fruit fly Drosophila melanogaster intestinal degeneration and tumours are seen females but not males due to stem cell hyperproliferation in the female intestine (Regan et al., 2016). Female mice are also susceptible to more severe intestinal inflammation and autoimmune disorders compared to males (Livingston et al., 2004; McGee and Huttenhower, 2021). Similar trends are seen in humans, where women are more susceptible to certain gastrointestinal autoimmune diseases (e.g. irritable bowel syndrome) and gastrointestinal cancer (Chang and Heitkemper, 2002; Jemal et al., 2011; Kim et al., 2015; McGee and Huttenhower, 2021).
|
|
Disclaimer/Publisher’s Note: The statements, opinions and data contained in all publications are solely those of the individual author(s) and contributor(s) and not of MDPI and/or the editor(s). MDPI and/or the editor(s) disclaim responsibility for any injury to people or property resulting from any ideas, methods, instructions or products referred to in the content. |
© 2024 by the authors. Licensee MDPI, Basel, Switzerland. This article is an open access article distributed under the terms and conditions of the Creative Commons Attribution (CC BY) license (http://creativecommons.org/licenses/by/4.0/).