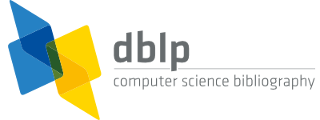


default search action
Michael Kearns
Person information
- affiliation: Department of Computer and Information Science, University of Pennsylvania
Refine list

refinements active!
zoomed in on ?? of ?? records
view refined list in
export refined list as
2020 – today
- 2024
- [c152]Ira Globus-Harris
, Declan Harrison
, Michael Kearns
, Pietro Perona
, Aaron Roth
:
Diversified Ensembling: An Experiment in Crowdsourced Machine Learning. FAccT 2024: 529-545 - [c151]Siqi Deng, Emily Diana, Michael Kearns, Aaron Roth:
Balanced Filtering via Disclosure-Controlled Proxies. FORC 2024: 4:1-4:23 - [c150]Shuai Tang, Steven Wu, Sergül Aydöre, Michael Kearns, Aaron Roth:
Membership Inference Attacks on Diffusion Models via Quantile Regression. ICML 2024 - [c149]Shuai Tang, Sergül Aydöre, Michael Kearns, Saeyoung Rho, Aaron Roth, Yichen Wang, Yu-Xiang Wang, Zhiwei Steven Wu:
Improved Differentially Private Regression via Gradient Boosting. SaTML 2024: 33-56 - [i60]Ira Globus-Harris, Declan Harrison, Michael Kearns, Pietro Perona, Aaron Roth:
Diversified Ensembling: An Experiment in Crowdsourced Machine Learning. CoRR abs/2402.10795 (2024) - [i59]Marcel Hussing, Michael Kearns, Aaron Roth, Sikata Bela Sengupta, Jessica Sorrell:
Oracle-Efficient Reinforcement Learning for Max Value Ensembles. CoRR abs/2405.16739 (2024) - [i58]Ira Globus-Harris, Varun Gupta, Michael Kearns, Aaron Roth:
Model Ensembling for Constrained Optimization. CoRR abs/2405.16752 (2024) - [i57]Martín Bertrán, Shuai Tang, Michael Kearns, Jamie Morgenstern, Aaron Roth, Zhiwei Steven Wu:
Reconstruction Attacks on Machine Unlearning: Simple Models are Vulnerable. CoRR abs/2405.20272 (2024) - 2023
- [c148]Ira Globus-Harris
, Varun Gupta
, Christopher Jung
, Michael Kearns
, Jamie Morgenstern
, Aaron Roth
:
Multicalibrated Regression for Downstream Fairness. AIES 2023: 259-286 - [c147]Natalie Collina, Eshwar Ram Arunachaleswaran, Michael Kearns:
Efficient Stackelberg Strategies for Finitely Repeated Games. AAMAS 2023: 643-651 - [c146]Ira Globus-Harris, Declan Harrison, Michael Kearns, Aaron Roth, Jessica Sorrell:
Multicalibration as Boosting for Regression. ICML 2023: 11459-11492 - [c145]Martín Bertrán, Shuai Tang, Aaron Roth, Michael Kearns, Jamie Morgenstern, Steven Wu:
Scalable Membership Inference Attacks via Quantile Regression. NeurIPS 2023 - [c144]Eric Eaton, Marcel Hussing, Michael Kearns, Jessica Sorrell:
Replicable Reinforcement Learning. NeurIPS 2023 - [i56]Ira Globus-Harris, Declan Harrison, Michael Kearns, Aaron Roth
, Jessica Sorrell:
Multicalibration as Boosting for Regression. CoRR abs/2301.13767 (2023) - [i55]Shuai Tang, Sergül Aydöre, Michael Kearns, Saeyoung Rho, Aaron Roth, Yichen Wang, Yu-Xiang Wang, Zhiwei Steven Wu:
Improved Differentially Private Regression via Gradient Boosting. CoRR abs/2303.03451 (2023) - [i54]Alessandro Achille, Michael Kearns, Carson Klingenberg, Stefano Soatto:
AI Model Disgorgement: Methods and Choices. CoRR abs/2304.03545 (2023) - [i53]Eric Eaton, Marcel Hussing, Michael Kearns, Jessica Sorrell:
Replicable Reinforcement Learning. CoRR abs/2305.15284 (2023) - [i52]Siqi Deng, Emily Diana, Michael Kearns, Aaron Roth:
Balanced Filtering via Non-Disclosive Proxies. CoRR abs/2306.15083 (2023) - [i51]Martín Bertrán, Shuai Tang, Michael Kearns, Jamie Morgenstern, Aaron Roth, Zhiwei Steven Wu:
Scalable Membership Inference Attacks via Quantile Regression. CoRR abs/2307.03694 (2023) - [i50]Shuai Tang, Zhiwei Steven Wu, Sergül Aydöre, Michael Kearns, Aaron Roth:
Membership Inference Attacks on Diffusion Models via Quantile Regression. CoRR abs/2312.05140 (2023) - 2022
- [c143]Aditya Golatkar, Alessandro Achille, Yu-Xiang Wang, Aaron Roth
, Michael Kearns, Stefano Soatto:
Mixed Differential Privacy in Computer Vision. CVPR 2022: 8366-8376 - [c142]Ira Globus-Harris, Michael Kearns, Aaron Roth
:
An Algorithmic Framework for Bias Bounties. FAccT 2022: 1106-1124 - [c141]Emily Diana, Wesley Gill, Michael Kearns, Krishnaram Kenthapadi, Aaron Roth
, Saeed Sharifi-Malvajerdi:
Multiaccurate Proxies for Downstream Fairness. FAccT 2022: 1207-1239 - [c140]Giuseppe Vietri, Cédric Archambeau, Sergül Aydöre, William Brown, Michael Kearns, Aaron Roth, Amaresh Ankit Siva, Shuai Tang, Zhiwei Steven Wu:
Private Synthetic Data for Multitask Learning and Marginal Queries. NeurIPS 2022 - [i49]Ira Globus-Harris, Michael Kearns, Aaron Roth:
Beyond the Frontier: Fairness Without Accuracy Loss. CoRR abs/2201.10408 (2022) - [i48]Aditya Golatkar, Alessandro Achille, Yu-Xiang Wang, Aaron Roth, Michael Kearns, Stefano Soatto:
Mixed Differential Privacy in Computer Vision. CoRR abs/2203.11481 (2022) - [i47]Eshwar Ram Arunachaleswaran, Natalie Collina, Michael Kearns:
Efficient Stackelberg Strategies for Finitely Repeated Games. CoRR abs/2207.04192 (2022) - [i46]Ira Globus-Harris, Varun Gupta, Christopher Jung, Michael Kearns, Jamie Morgenstern, Aaron Roth
:
Multicalibrated Regression for Downstream Fairness. CoRR abs/2209.07312 (2022) - [i45]Giuseppe Vietri, Cédric Archambeau, Sergül Aydöre, William Brown, Michael Kearns, Aaron Roth
, Amaresh Ankit Siva, Shuai Tang, Zhiwei Steven Wu:
Private Synthetic Data for Multitask Learning and Marginal Queries. CoRR abs/2209.07400 (2022) - [i44]Travis Dick, Cynthia Dwork, Michael Kearns, Terrance Liu, Aaron Roth
, Giuseppe Vietri, Zhiwei Steven Wu:
Confidence-Ranked Reconstruction of Census Microdata from Published Statistics. CoRR abs/2211.03128 (2022) - 2021
- [c139]Emily Diana, Wesley Gill, Michael Kearns, Krishnaram Kenthapadi, Aaron Roth
:
Minimax Group Fairness: Algorithms and Experiments. AIES 2021: 66-76 - [c138]Christopher Jung, Michael Kearns, Seth Neel, Aaron Roth
, Logan Stapleton, Zhiwei Steven Wu
:
An Algorithmic Framework for Fairness Elicitation. FORC 2021: 2:1-2:19 - [c137]Emily Diana, Wesley Gill, Ira Globus-Harris, Michael Kearns, Aaron Roth
, Saeed Sharifi-Malvajerdi:
Lexicographically Fair Learning: Algorithms and Generalization. FORC 2021: 6:1-6:23 - [c136]Sergül Aydöre, William Brown, Michael Kearns, Krishnaram Kenthapadi, Luca Melis, Aaron Roth, Amaresh Ankit Siva:
Differentially Private Query Release Through Adaptive Projection. ICML 2021: 457-467 - [c135]Emily Diana, Travis Dick, Hadi Elzayn, Michael Kearns, Aaron Roth
, Zachary Schutzman, Saeed Sharifi-Malvajerdi, Juba Ziani:
Algorithms and Learning for Fair Portfolio Design. EC 2021: 371-389 - [i43]Emily Diana, Wesley Gill, Ira Globus-Harris, Michael Kearns, Aaron Roth, Saeed Sharifi-Malvajerdi:
Lexicographically Fair Learning: Algorithms and Generalization. CoRR abs/2102.08454 (2021) - [i42]Sergül Aydöre, William Brown, Michael Kearns, Krishnaram Kenthapadi, Luca Melis, Aaron Roth, Amaresh Ankit Siva
:
Differentially Private Query Release Through Adaptive Projection. CoRR abs/2103.06641 (2021) - [i41]Emily Diana, Wesley Gill, Michael Kearns, Krishnaram Kenthapadi, Aaron Roth, Saeed Sharifi-Malvajerdi:
Multiaccurate Proxies for Downstream Fairness. CoRR abs/2107.04423 (2021) - 2020
- [j38]Michael Kearns, Aaron Roth:
Ethical algorithm design. SIGecom Exch. 18(1): 31-36 (2020) - [c134]Emily Diana, Michael Kearns, Seth Neel, Aaron Roth
:
Optimal, truthful, and private securities lending. ICAIF 2020: 48:1-48:8 - [c133]Emily Diana, Hadi Elzayn, Michael Kearns, Aaron Roth
, Saeed Sharifi-Malvajerdi, Juba Ziani:
Differentially Private Call Auctions and Market Impact. EC 2020: 541-583 - [i40]Emily Diana, Hadi Elzayn, Michael J. Kearns, Aaron Roth, Saeed Sharifi-Malvajerdi, Juba Ziani:
Differentially Private Call Auctions and Market Impact. CoRR abs/2002.05699 (2020) - [i39]Emily Diana, Travis Dick, Hadi Elzayn, Michael J. Kearns, Aaron Roth, Zachary Schutzman, Saeed Sharifi-Malvajerdi, Juba Ziani:
Algorithms and Learning for Fair Portfolio Design. CoRR abs/2006.07281 (2020) - [i38]Yiling Chen, Arpita Ghosh, Michael Kearns, Tim Roughgarden, Jennifer Wortman Vaughan:
Mathematical Foundations for Social Computing. CoRR abs/2007.03661 (2020) - [i37]Emily Diana, Wesley Gill, Michael Kearns, Krishnaram Kenthapadi, Aaron Roth:
Convergent Algorithms for (Relaxed) Minimax Fairness. CoRR abs/2011.03108 (2020)
2010 – 2019
- 2019
- [j37]Sanjeev Goyal, Hoda Heidari, Michael J. Kearns:
Competitive contagion in networks. Games Econ. Behav. 113: 58-79 (2019) - [c132]Michael J. Kearns, Seth Neel, Aaron Roth
, Zhiwei Steven Wu
:
An Empirical Study of Rich Subgroup Fairness for Machine Learning. FAT 2019: 100-109 - [c131]Hadi Elzayn, Shahin Jabbari, Christopher Jung, Michael J. Kearns, Seth Neel, Aaron Roth
, Zachary Schutzman
:
Fair Algorithms for Learning in Allocation Problems. FAT 2019: 170-179 - [c130]Matthew Jagielski, Michael J. Kearns, Jieming Mao, Alina Oprea, Aaron Roth, Saeed Sharifi-Malvajerdi, Jonathan R. Ullman:
Differentially Private Fair Learning. ICML 2019: 3000-3008 - [c129]Yu Chen, Shahin Jabbari, Michael J. Kearns, Sanjeev Khanna, Jamie Morgenstern:
Network Formation under Random Attack and Probabilistic Spread. IJCAI 2019: 180-186 - [c128]Jinshuo Dong, Hadi Elzayn, Shahin Jabbari, Michael J. Kearns, Zachary Schutzman
:
Equilibrium Characterization for Data Acquisition Games. IJCAI 2019: 252-258 - [c127]Saeed Sharifi-Malvajerdi, Michael J. Kearns, Aaron Roth:
Average Individual Fairness: Algorithms, Generalization and Experiments. NeurIPS 2019: 8240-8249 - [i36]Jinshuo Dong, Hadi Elzayn, Shahin Jabbari, Michael J. Kearns, Zachary Schutzman
:
Equilibrium Characterization for Data Acquisition Games. CoRR abs/1905.08909 (2019) - [i35]Michael J. Kearns, Aaron Roth, Saeed Sharifi-Malvajerdi:
Average Individual Fairness: Algorithms, Generalization and Experiments. CoRR abs/1905.10607 (2019) - [i34]Christopher Jung, Michael J. Kearns, Seth Neel, Aaron Roth, Logan Stapleton, Zhiwei Steven Wu:
Eliciting and Enforcing Subjective Individual Fairness. CoRR abs/1905.10660 (2019) - [i33]Yu Chen, Shahin Jabbari, Michael J. Kearns, Sanjeev Khanna, Jamie Morgenstern:
Network Formation under Random Attack and Probabilistic Spread. CoRR abs/1906.00241 (2019) - [i32]Emily Diana, Michael J. Kearns, Seth Neel, Aaron Roth:
Optimal, Truthful, and Private Securities Lending. CoRR abs/1912.06202 (2019) - 2018
- [c126]Matthew Joseph, Michael J. Kearns, Jamie Morgenstern, Seth Neel, Aaron Roth
:
Meritocratic Fairness for Infinite and Contextual Bandits. AIES 2018: 158-163 - [c125]Michael J. Kearns, Seth Neel, Aaron Roth, Zhiwei Steven Wu:
Preventing Fairness Gerrymandering: Auditing and Learning for Subgroup Fairness. ICML 2018: 2569-2577 - [c124]Stephen Gillen, Christopher Jung, Michael J. Kearns, Aaron Roth:
Online Learning with an Unknown Fairness Metric. NeurIPS 2018: 2605-2614 - [i31]Stephen Gillen, Christopher Jung, Michael J. Kearns, Aaron Roth:
Online Learning with an Unknown Fairness Metric. CoRR abs/1802.06936 (2018) - [i30]Michael J. Kearns, Seth Neel, Aaron Roth, Zhiwei Steven Wu:
An Empirical Study of Rich Subgroup Fairness for Machine Learning. CoRR abs/1808.08166 (2018) - [i29]Hadi Elzayn, Shahin Jabbari, Christopher Jung, Michael J. Kearns, Seth Neel, Aaron Roth, Zachary Schutzman
:
Fair Algorithms for Learning in Allocation Problems. CoRR abs/1808.10549 (2018) - [i28]Matthew Jagielski, Michael J. Kearns, Jieming Mao, Alina Oprea, Aaron Roth, Saeed Sharifi-Malvajerdi, Jonathan R. Ullman:
Differentially Private Fair Learning. CoRR abs/1812.02696 (2018) - 2017
- [c123]Michael J. Kearns, Zhiwei Steven Wu:
Predicting with Distributions. COLT 2017: 1214-1241 - [c122]Shahin Jabbari, Matthew Joseph, Michael J. Kearns, Jamie Morgenstern, Aaron Roth:
Fairness in Reinforcement Learning. ICML 2017: 1617-1626 - [c121]Michael J. Kearns, Aaron Roth, Zhiwei Steven Wu:
Meritocratic Fairness for Cross-Population Selection. ICML 2017: 1828-1836 - [c120]Michael J. Kearns:
Fair Algorithms for Machine Learning. EC 2017: 1 - [c119]Sampath Kannan, Michael J. Kearns, Jamie Morgenstern, Mallesh M. Pai, Aaron Roth
, Rakesh V. Vohra, Zhiwei Steven Wu:
Fairness Incentives for Myopic Agents. EC 2017: 369-386 - [i27]Sampath Kannan, Michael J. Kearns, Jamie Morgenstern, Mallesh M. Pai, Aaron Roth, Rakesh V. Vohra, Zhiwei Steven Wu:
Fairness Incentives for Myopic Agents. CoRR abs/1705.02321 (2017) - [i26]Richard Berk, Hoda Heidari, Shahin Jabbari, Matthew Joseph, Michael J. Kearns, Jamie Morgenstern, Seth Neel, Aaron Roth:
A Convex Framework for Fair Regression. CoRR abs/1706.02409 (2017) - [i25]Michael J. Kearns, Seth Neel, Aaron Roth, Zhiwei Steven Wu:
Preventing Fairness Gerrymandering: Auditing and Learning for Subgroup Fairness. CoRR abs/1711.05144 (2017) - 2016
- [j36]Yiling Chen, Arpita Ghosh, Michael J. Kearns, Tim Roughgarden, Jennifer Wortman Vaughan:
Mathematical foundations for social computing. Commun. ACM 59(12): 102-108 (2016) - [j35]Michael J. Kearns, Aaron Roth
, Zhiwei Steven Wu, Grigory Yaroslavtsev:
Private algorithms for the protected in social network search. Proc. Natl. Acad. Sci. USA 113(4): 913-918 (2016) - [c118]Hoda Heidari, Michael J. Kearns, Aaron Roth:
Tight Policy Regret Bounds for Improving and Decaying Bandits. IJCAI 2016: 1562-1570 - [c117]Matthew Joseph, Michael J. Kearns, Jamie Morgenstern, Aaron Roth:
Fairness in Learning: Classic and Contextual Bandits. NIPS 2016: 325-333 - [c116]Sanjeev Goyal, Shahin Jabbari, Michael J. Kearns, Sanjeev Khanna, Jamie Morgenstern:
Strategic Network Formation with Attack and Immunization. WINE 2016: 429-443 - [i24]Matthew Joseph, Michael J. Kearns, Jamie Morgenstern, Aaron Roth:
Fairness in Learning: Classic and Contextual Bandits. CoRR abs/1605.07139 (2016) - [i23]Michael J. Kearns, Zhiwei Steven Wu:
Predicting with Distributions. CoRR abs/1606.01275 (2016) - [i22]Matthew Joseph, Michael J. Kearns, Jamie Morgenstern, Seth Neel, Aaron Roth:
Rawlsian Fairness for Machine Learning. CoRR abs/1610.09559 (2016) - [i21]Shahin Jabbari, Matthew Joseph, Michael J. Kearns, Jamie Morgenstern, Aaron Roth:
Fair Learning in Markovian Environments. CoRR abs/1611.03071 (2016) - 2015
- [c115]Kareem Amin, Rachel Cummings, Lili Dworkin, Michael J. Kearns, Aaron Roth:
Online Learning and Profit Maximization from Revealed Preferences. AAAI 2015: 770-776 - [c114]Lili Dworkin, Michael J. Kearns:
From "In" to "Over": Behavioral Experiments on Whole-Network Computation. HCOMP 2015: 52-61 - [c113]Rachel Cummings, Michael J. Kearns, Aaron Roth
, Zhiwei Steven Wu:
Privacy and Truthful Equilibrium Selection for Aggregative Games. WINE 2015: 286-299 - [i20]Michael J. Kearns, Aaron Roth, Zhiwei Steven Wu, Grigory Yaroslavtsev:
Privacy for the Protected (Only). CoRR abs/1506.00242 (2015) - [i19]Sanjeev Goyal, Shahin Jabbari, Michael J. Kearns, Sanjeev Khanna, Jamie Morgenstern:
Strategic Network Formation with Attack and Immunization. CoRR abs/1511.05196 (2015) - [i18]Michael J. Kearns, Mallesh M. Pai, Ryan M. Rogers, Aaron Roth, Jonathan R. Ullman:
Robust Mediators in Large Games. CoRR abs/1512.02698 (2015) - 2014
- [c112]Moez Draief, Hoda Heidari, Michael J. Kearns:
New Models for Competitive Contagion. AAAI 2014: 637-644 - [c111]Lili Dworkin, Michael J. Kearns, Lirong Xia:
Efficient Inference for Complex Queries on Complex Distributions. AISTATS 2014: 211-219 - [c110]Lili Dworkin, Michael J. Kearns, Yuriy Nevmyvaka:
Pursuit-Evasion Without Regret, with an Application to Trading. ICML 2014: 1521-1529 - [c109]Kareem Amin, Hoda Heidari, Michael J. Kearns:
Learning from Contagion (Without Timestamps). ICML 2014: 1845-1853 - [c108]Michael J. Kearns, Mallesh M. Pai, Aaron Roth
, Jonathan R. Ullman:
Mechanism design in large games: incentives and privacy. ITCS 2014: 403-410 - [i17]Michael J. Kearns, Lili Dworkin:
A Computational Study of Feasible Repackings in the FCC Incentive Auctions. CoRR abs/1406.4837 (2014) - [i16]Kareem Amin, Rachel Cummings, Lili Dworkin, Michael J. Kearns, Aaron Roth:
Online Learning and Profit Maximization from Revealed Preferences. CoRR abs/1407.7294 (2014) - [i15]Rachel Cummings, Michael J. Kearns, Aaron Roth, Zhiwei Steven Wu:
Privacy and Truthful Equilibrium Selection for Aggregative Games. CoRR abs/1407.7740 (2014) - 2013
- [c107]Hoda Heidari, Michael J. Kearns:
Depth-Workload Tradeoffs for Workforce Organization. HCOMP 2013: 60-68 - [c106]Jacob D. Abernethy, Kareem Amin, Michael J. Kearns, Moez Draief:
Large-Scale Bandit Problems and KWIK Learning. ICML (1) 2013: 588-596 - [c105]Tim Roughgarden, Michael J. Kearns:
Marginals-to-Models Reducibility. NIPS 2013: 1043-1051 - [e6]Michael J. Kearns, R. Preston McAfee, Éva Tardos:
Proceedings of the fourteenth ACM Conference on Electronic Commerce, EC 2013, Philadelphia, PA, USA, June 16-20, 2013. ACM 2013, ISBN 978-1-4503-1962-1 [contents] - [i14]Michael J. Kearns, Yishay Mansour:
Efficient Nash Computation in Large Population Games with Bounded Influence. CoRR abs/1301.0577 (2013) - [i13]Michael J. Kearns, Michael L. Littman, Satinder Singh:
Graphical Models for Game Theory. CoRR abs/1301.2281 (2013) - [i12]Michael J. Kearns, Yishay Mansour, Satinder Singh:
Fast Planning in Stochastic Games. CoRR abs/1301.3867 (2013) - [i11]Satinder Singh, Michael J. Kearns, Yishay Mansour:
Nash Convergence of Gradient Dynamics in Iterated General-Sum Games. CoRR abs/1301.3892 (2013) - [i10]Michael J. Kearns, Yishay Mansour:
Exact Inference of Hidden Structure from Sample Data in Noisy-OR Networks. CoRR abs/1301.7391 (2013) - [i9]Michael J. Kearns, Lawrence K. Saul:
Large Deviation Methods for Approximate Probabilistic Inference. CoRR abs/1301.7392 (2013) - [i8]Michael J. Kearns, Yishay Mansour, Andrew Y. Ng:
An Information-Theoretic Analysis of Hard and Soft Assignment Methods for Clustering. CoRR abs/1302.1552 (2013) - 2012
- [j34]Michael J. Kearns:
Experiments in social computation. Commun. ACM 55(10): 56-67 (2012) - [c104]Quang Duong, Michael P. Wellman, Satinder Singh, Michael J. Kearns:
Learning and predicting dynamic networked behavior with graphical multiagent models. AAMAS 2012: 441-448 - [c103]Michael J. Kearns:
Experiments in social computation: (and the data they generate). KDD 2012: 5 - [c102]Michael J. Kearns, J. Stephen Judd, Yevgeniy Vorobeychik
:
Behavioral experiments on a network formation game. EC 2012: 690-704 - [c101]Sanjeev Goyal, Michael J. Kearns:
Competitive contagion in networks. STOC 2012: 759-774 - [c100]Kareem Amin, Michael J. Kearns, Peter B. Key, Anton Schwaighofer:
Budget Optimization for Sponsored Search: Censored Learning in MDPs. UAI 2012: 54-63 - [c99]Pushmeet Kohli, Michael J. Kearns, Yoram Bachrach, Ralf Herbrich, David Stillwell, Thore Graepel:
Colonel Blotto on Facebook: the effect of social relations on strategic interaction. WebSci 2012: 141-150 - [i7]Kareem Amin, Michael J. Kearns, Umar Syed:
Graphical Models for Bandit Problems. CoRR abs/1202.3782 (2012) - [i6]Kuzman Ganchev, Michael J. Kearns, Yuriy Nevmyvaka, Jennifer Wortman Vaughan:
Censored Exploration and the Dark Pool Problem. CoRR abs/1205.2646 (2012) - [i5]Kareem Amin, Michael J. Kearns, Peter B. Key, Anton Schwaighofer:
Budget Optimization for Sponsored Search: Censored Learning in MDPs. CoRR abs/1210.4847 (2012) - 2011
- [c98]Michael Brautbar, Michael J. Kearns:
A Clustering Coefficient Network Formation Game. SAGT 2011: 224-235 - [c97]Tanmoy Chakraborty, Michael J. Kearns:
Market making and mean reversion. EC 2011: 307-314 - [c96]Kareem Amin, Michael J. Kearns, Umar Syed:
Graphical Models for Bandit Problems. UAI 2011: 1-10 - [c95]J. Stephen Judd, Michael J. Kearns, Yevgeniy Vorobeychik:
Behavioral Conflict and Fairness in Social Networks. WINE 2011: 242-253 - [c94]Kareem Amin, Michael J. Kearns, Umar Syed:
Bandits, Query Learning, and the Haystack Dimension. COLT 2011: 87-106 - [i4]Michael J. Kearns, Diane J. Litman, Satinder Singh, Marilyn A. Walker:
Optimizing Dialogue Management with Reinforcement Learning: Experiments with the NJFun System. CoRR abs/1106.0676 (2011) - [i3]Michael J. Kearns, Michael L. Littman, Satinder Singh, Peter Stone:
ATTac-2000: An Adaptive Autonomous Bidding Agent. CoRR abs/1106.0678 (2011) - [i2]Sanjeev Goyal, Michael J. Kearns:
Competitive Contagion in Networks. CoRR abs/1110.6372 (2011) - 2010
- [j33]Kuzman Ganchev, Yuriy Nevmyvaka, Michael J. Kearns, Jennifer Wortman Vaughan:
Censored exploration and the dark pool problem. Commun. ACM 53(5): 99-107 (2010) - [j32]J. Stephen Judd, Michael J. Kearns, Yevgeniy Vorobeychik
:
Behavioral dynamics and influence in networked coloring and consensus. Proc. Natl. Acad. Sci. USA 107(34): 14978-14982 (2010) - [c93]Mickey Brautbar, Michael J. Kearns, Umar Syed:
Private and Third-Party Randomization in Risk-Sensitive Equilibrium Concepts. AAAI 2010: 723-728 - [c92]Mickey Brautbar, Michael J. Kearns:
Local Algorithms for Finding Interesting Individuals in Large Networks. ICS 2010: 188-199 - [c91]Tanmoy Chakraborty, J. Stephen Judd, Michael J. Kearns, Jinsong Tan:
A behavioral study of bargaining in social networks. EC 2010: 243-252 - [i1]Mickey Brautbar, Michael J. Kearns:
A Clustering Coefficient Network Formation Game. CoRR abs/1010.1561 (2010)
2000 – 2009
- 2009
- [j31]Michael J. Kearns, J. Stephen Judd, Jinsong Tan, Jennifer Wortman:
Behavioral experiments on biased voting in networks. Proc. Natl. Acad. Sci. USA 106(5): 1347-1352 (2009) - [c90]Tanmoy Chakraborty, Michael J. Kearns, Sanjeev Khanna:
Network bargaining: algorithms and structural results. EC 2009: 159-168 - [c89]Kuzman Ganchev, Michael J. Kearns, Yuriy Nevmyvaka, Jennifer Wortman Vaughan:
Censored Exploration and the Dark Pool Problem. UAI 2009: 185-194 - 2008
- [j30]Koby Crammer, Michael J. Kearns, Jennifer Wortman:
Learning from Multiple Sources. J. Mach. Learn. Res. 9: 1757-1774 (2008) - [j29]Eyal Even-Dar, Michael J. Kearns, Yishay Mansour, Jennifer Wortman:
Regret to the best vs. regret to the average. Mach. Learn. 72(1-2): 21-37 (2008) - [c88]Michael J. Kearns, Jennifer Wortman:
Learning from Collective Behavior. COLT 2008: 99-110 - [c87]J. Stephen Judd, Michael J. Kearns:
Behavioral experiments in networked trade. EC 2008: 150-159 - [c86]Tanmoy Chakraborty, Michael J. Kearns:
Bargaining Solutions in a Social Network. WINE 2008: 548-555 - [c85]Michael J. Kearns, Jinsong Tan:
Biased Voting and the Democratic Primary Problem. WINE 2008: 639-652 - 2007
- [c84]Eyal Even-Dar, Michael J. Kearns, Yishay Mansour, Jennifer Wortman:
Regret to the Best vs. Regret to the Average. COLT 2007: 233-247 - [c83]Michael J. Kearns, Jinsong Tan, Jennifer Wortman:
Privacy-Preserving Belief Propagation and Sampling. NIPS 2007: 745-752 - [c82]Eyal Even-Dar, Michael J. Kearns, Siddharth Suri:
A network formation game for bipartite exchange economies. SODA 2007: 697-706 - [c81]Eyal Even-Dar, Michael J. Kearns, Jennifer Wortman:
Sponsored Search with Contexts. WINE 2007: 312-317 - [c80]Kuzman Ganchev, Alex Kulesza, Jinsong Tan, Ryan Gabbard, Qian Liu, Michael J. Kearns:
Empirical Price Modeling for Sponsored Search. WINE 2007: 541-548 - 2006
- [j28]Charles Lee Isbell Jr., Michael J. Kearns, Satinder Singh, Christian R. Shelton
, Peter Stone, David P. Kormann:
Cobot in LambdaMOO: An Adaptive Social Statistics Agent. Auton. Agents Multi Agent Syst. 13(3): 327-354 (2006) - [c79]Eyal Even-Dar, Michael J. Kearns, Jennifer Wortman:
Risk-Sensitive Online Learning. ALT 2006: 199-213 - [c78]Yuriy Nevmyvaka, Yi Feng, Michael J. Kearns:
Reinforcement learning for optimized trade execution. ICML 2006: 673-680 - [c77]Koby Crammer, Michael J. Kearns, Jennifer Wortman:
Learning from Multiple Sources. NIPS 2006: 321-328 - [c76]Eyal Even-Dar, Michael J. Kearns:
A Small World Threshold for Economic Network Formation. NIPS 2006: 385-392 - [c75]Eyal Even-Dar, Sham M. Kakade, Michael J. Kearns, Yishay Mansour:
(In)Stability properties of limit order dynamics. EC 2006: 120-129 - [c74]Michael J. Kearns, Siddharth Suri:
Networks preserving evolutionary equilibria and the power of randomization. EC 2006: 200-207 - 2005
- [c73]Sham M. Kakade, Michael J. Kearns:
Trading in Markovian Price Models. COLT 2005: 606-620 - [c72]Koby Crammer, Michael J. Kearns, Jennifer Wortman:
Learning from Data of Variable Quality. NIPS 2005: 219-226 - [c71]Yuriy Nevmyvaka, Michael J. Kearns, Amy Papandreou, Katia P. Sycara:
Electronic Trading in Order-Driven Markets: Efficient Execution. CEC 2005: 190-197 - [e5]John Riedl, Michael J. Kearns, Michael K. Reiter:
Proceedings 6th ACM Conference on Electronic Commerce (EC-2005), Vancouver, BC, Canada, June 5-8, 2005. ACM 2005, ISBN 1-59593-049-3 [contents] - 2004
- [c70]Sham M. Kakade, Michael J. Kearns, Luis E. Ortiz
:
Graphical Economics. COLT 2004: 17-32 - [c69]Sham M. Kakade, Michael J. Kearns, Luis E. Ortiz, Robin Pemantle, Siddharth Suri:
Economic Properties of Social Networks. NIPS 2004: 633-640 - [c68]Sham M. Kakade, Michael J. Kearns, Yishay Mansour, Luis E. Ortiz
:
Competitive algorithms for VWAP and limit order trading. EC 2004: 189-198 - 2003
- [j27]Michael J. Kearns, Luis E. Ortiz
:
The Penn-Lehman Automated Trading Project. IEEE Intell. Syst. 18(6): 22-31 (2003) - [c67]Sham M. Kakade, Michael J. Kearns, John Langford:
Exploration in Metric State Spaces. ICML 2003: 306-312 - [c66]Michael J. Kearns, Luis E. Ortiz:
Algorithms for Interdependent Security Games. NIPS 2003: 561-568 - [c65]Sham M. Kakade, Michael J. Kearns, John Langford, Luis E. Ortiz
:
Correlated equilibria in graphical games. EC 2003: 42-47 - [c64]Michael J. Kearns:
Structured interaction in game theory. TARK 2003: 88 - 2002
- [j26]Satinder Singh, Diane J. Litman, Michael J. Kearns, Marilyn A. Walker:
Optimizing Dialogue Management with Reinforcement Learning: Experiments with the NJFun System. J. Artif. Intell. Res. 16: 105-133 (2002) - [j25]Michael J. Kearns, Yishay Mansour, Andrew Y. Ng:
A Sparse Sampling Algorithm for Near-Optimal Planning in Large Markov Decision Processes. Mach. Learn. 49(2-3): 193-208 (2002) - [j24]Michael J. Kearns, Satinder Singh:
Near-Optimal Reinforcement Learning in Polynomial Time. Mach. Learn. 49(2-3): 209-232 (2002) - [c63]Michael J. Kearns, Charles Lee Isbell Jr., Satinder Singh, Diane J. Litman, Jessica Howe:
CobotDS: A Spoken Dialogue System for Chat. AAAI/IAAI 2002: 425-430 - [c62]Eric Allender, Sanjeev Arora, Michael J. Kearns, Cristopher Moore, Alexander Russell:
A Note on the Representational Incompatibility of Function Approximation and Factored Dynamics. NIPS 2002: 431-437 - [c61]Luis E. Ortiz, Michael J. Kearns:
Nash Propagation for Loopy Graphical Games. NIPS 2002: 793-800 - [c60]Michael J. Kearns, Yishay Mansour:
Efficient Nash Computation in Large Population Games with Bounded Influence. UAI 2002: 259-266 - [e4]Rina Dechter, Michael J. Kearns, Richard S. Sutton:
Proceedings of the Eighteenth National Conference on Artificial Intelligence and Fourteenth Conference on Innovative Applications of Artificial Intelligence, July 28 - August 1, 2002, Edmonton, Alberta, Canada. AAAI Press / The MIT Press 2002 [contents] - 2001
- [j23]Peter Stone, Michael L. Littman, Satinder Singh, Michael J. Kearns:
ATTac-2000: An Adaptive Autonomous Bidding Agent. J. Artif. Intell. Res. 15: 189-206 (2001) - [c59]Peter Stone, Michael L. Littman, Satinder Singh, Michael J. Kearns:
ATTac-2000: an adaptive autonomous bidding agent. Agents 2001: 238-245 - [c58]Charles Lee Isbell Jr., Christian R. Shelton, Michael J. Kearns, Satinder Singh, Peter Stone:
A social reinforcement learning agent. Agents 2001: 377-384 - [c57]Michael J. Kearns:
Computational Game Theory and AI. KI/ÖGAI 2001: 1 - [c56]Michael L. Littman, Michael J. Kearns, Satinder Singh:
An Efficient, Exact Algorithm for Solving Tree-Structured Graphical Games. NIPS 2001: 817-823 - [c55]Charles Lee Isbell Jr., Christian R. Shelton, Michael J. Kearns, Satinder Singh, Peter Stone:
Cobot: A Social Reinforcement Learning Agent. NIPS 2001: 1393-1400 - [c54]Michael J. Kearns, Michael L. Littman, Satinder Singh:
Graphical Models for Game Theory. UAI 2001: 253-260 - 2000
- [j22]Michael J. Kearns, Dana Ron
:
Testing Problems with Sublearning Sample Complexity. J. Comput. Syst. Sci. 61(3): 428-456 (2000) - [c53]Charles Lee Isbell Jr., Michael J. Kearns, David P. Kormann, Satinder Singh, Peter Stone:
Cobot in LambdaMOO: A Social Statistics Agent. AAAI/IAAI 2000: 36-41 - [c52]Satinder Singh, Michael J. Kearns, Diane J. Litman, Marilyn A. Walker:
Empirical Evaluation of a Reinforcement Learning Spoken Dialogue System. AAAI/IAAI 2000: 645-651 - [c51]Diane J. Litman, Michael S. Kearns, Satinder Singh, Marilyn A. Walker:
Automatic Optimization of Dialogue Management. COLING 2000: 502-508 - [c50]Michael J. Kearns, Satinder Singh:
Bias-Variance Error Bounds for Temporal Difference Updates. COLT 2000: 142-147 - [c49]Kary L. Myers, Michael J. Kearns, Satinder Singh, Marilyn A. Walker:
A Boosting Approach to Topic Spotting on Subdialogues. ICML 2000: 655-662 - [c48]Michael J. Kearns, Yishay Mansour, Satinder Singh:
Fast Planning in Stochastic Games. UAI 2000: 309-316 - [c47]Satinder Singh, Michael J. Kearns, Yishay Mansour:
Nash Convergence of Gradient Dynamics in General-Sum Games. UAI 2000: 541-548
1990 – 1999
- 1999
- [j21]Michael J. Kearns, Yishay Mansour:
On the Boosting Ability of Top-Down Decision Tree Learning Algorithms. J. Comput. Syst. Sci. 58(1): 109-128 (1999) - [j20]Michael J. Kearns, Dana Ron:
Algorithmic Stability and Sanity-Check Bounds for Leave-One-Out Cross-Validation. Neural Comput. 11(6): 1427-1453 (1999) - [c46]Diane J. Litman, Marilyn A. Walker, Michael S. Kearns:
Automatic Detection of Poor Speech Recognition at the Dialogue Level. ACL 1999: 309-316 - [c45]Michael J. Kearns, Daphne Koller:
Efficient Reinforcement Learning in Factored MDPs. IJCAI 1999: 740-747 - [c44]Michael J. Kearns, Yishay Mansour, Andrew Y. Ng:
A Sparse Sampling Algorithm for Near-Optimal Planning in Large Markov Decision Processes. IJCAI 1999: 1324-1231 - [c43]Satinder Singh, Michael J. Kearns, Diane J. Litman, Marilyn A. Walker:
Reinforcement Learning for Spoken Dialogue Systems. NIPS 1999: 956-962 - [c42]Michael J. Kearns, Yishay Mansour, Andrew Y. Ng:
Approximate Planning in Large POMDPs via Reusable Trajectories. NIPS 1999: 1001-1007 - [e3]Michael J. Kearns, Sara A. Solla, David A. Cohn:
Advances in Neural Information Processing Systems 11, [NIPS Conference, Denver, Colorado, USA, November 30 - December 5, 1998]. The MIT Press 1999, ISBN 0-262-11245-0 [contents] - 1998
- [j19]Michael J. Kearns:
Efficient Noise-Tolerant Learning from Statistical Queries. J. ACM 45(6): 983-1006 (1998) - [c41]Michael J. Kearns, Dana Ron:
Testing Problems with Sub-Learning Sample Complexity. COLT 1998: 268-279 - [c40]Michael J. Kearns:
Theoretical Issues in Probabilistic Artificial Intelligence. FOCS 1998: 4 - [c39]Michael J. Kearns, Satinder Singh:
Near-Optimal Reinforcement Learning in Polynominal Time. ICML 1998: 260-268 - [c38]Michael J. Kearns, Yishay Mansour:
A Fast, Bottom-Up Decision Tree Pruning Algorithm with Near-Optimal Generalization. ICML 1998: 269-277 - [c37]Michael J. Kearns, Lawrence K. Saul:
Inference in Multilayer Networks via Large Deviation Bounds. NIPS 1998: 260-266 - [c36]Michael J. Kearns, Satinder Singh:
Finite-Sample Convergence Rates for Q-Learning and Indirect Algorithms. NIPS 1998: 996-1002 - [c35]Michael J. Kearns, Yishay Mansour:
Exact Inference of Hidden Structure from Sample Data in noisy-OR Networks. UAI 1998: 304-310 - [c34]Michael J. Kearns, Lawrence K. Saul:
Large Deviation Methods for Approximate Probabilistic Inference. UAI 1998: 311-319 - [p1]Michael J. Kearns, Yishay Mansour, Andrew Y. Ng:
An Information-Theoretic Analysis of Hard and Soft Assignment Methods for Clustering. Learning in Graphical Models 1998: 495-520 - [e2]Michael I. Jordan, Michael J. Kearns, Sara A. Solla:
Advances in Neural Information Processing Systems 10, [NIPS Conference, Denver, Colorado, USA, 1997]. The MIT Press 1998, ISBN 0-262-10076-2 [contents] - 1997
- [j18]Yoav Freund, Michael J. Kearns, Dana Ron
, Ronitt Rubinfeld, Robert E. Schapire, Linda Sellie:
Efficient Learning of Typical Finite Automata from Random Walks. Inf. Comput. 138(1): 23-48 (1997) - [j17]Michael J. Kearns, Yishay Mansour, Andrew Y. Ng, Dana Ron:
An Experimental and Theoretical Comparison of Model Selection Methods. Mach. Learn. 27(1): 7-50 (1997) - [j16]Michael J. Kearns:
A Bound on the Error of Cross Validation Using the Approximation and Estimation Rates, with Consequences for the Training-test Split. Neural Comput. 9(5): 1143-1161 (1997) - [c33]Michael J. Kearns, Dana Ron:
Algorithmic Stability and Sanity-Check Bounds for Leave-one-Out Cross-Validation. COLT 1997: 152-162 - [c32]Michael J. Kearns, Yishay Mansour, Andrew Y. Ng:
An Information-Theoretic Analysis of Hard and Soft Assignment Methods for Clustering. UAI 1997: 282-293 - 1996
- [j15]David Haussler, Michael J. Kearns, H. Sebastian Seung, Naftali Tishby:
Rigorous Learning Curve Bounds from Statistical Mechanics. Mach. Learn. 25(2-3): 195-236 (1996) - [c31]Michael J. Kearns:
Boosting Theory Towards Practice: Recent Developments in Decision Tree Induction and the Weak Learning Framework. AAAI/IAAI, Vol. 2 1996: 1337-1339 - [c30]Thomas G. Dietterich, Michael J. Kearns, Yishay Mansour:
Applying the Waek Learning Framework to Understand and Improve C4.5. ICML 1996: 96-104 - [c29]Michael J. Kearns, Yishay Mansour:
On the Boosting Ability of Top-Down Decision Tree Learning Algorithms. STOC 1996: 459-468 - [e1]Avrim Blum, Michael J. Kearns:
Proceedings of the Ninth Annual Conference on Computational Learning Theory, COLT 1996, Desenzano del Garda, Italy, June 28-July 1, 1996. ACM 1996, ISBN 0-89791-811-8 [contents] - 1995
- [j14]Henry A. Kautz
, Michael J. Kearns, Bart Selman:
Horn Approximations of Empirical Data. Artif. Intell. 74(1): 129-145 (1995) - [j13]Sally A. Goldman, Michael J. Kearns, Robert E. Schapire:
On the Sample Complexity of Weakly Learning. Inf. Comput. 117(2): 276-287 (1995) - [j12]Sally A. Goldman, Michael J. Kearns:
On the Complexity of Teaching. J. Comput. Syst. Sci. 50(1): 20-31 (1995) - [j11]Michael J. Kearns, H. Sebastian Seung:
Learning from a Population of Hypotheses. Mach. Learn. 18(2-3): 255-276 (1995) - [j10]Michael J. Kearns, Umesh V. Vazirani:
Computational Learning Theory. SIGACT News 26(1): 43-45 (1995) - [c28]Michael J. Kearns, Yishay Mansour, Andrew Y. Ng, Dana Ron:
An Experimental and Theoretical Comparison of Model Selection Methods. COLT 1995: 21-30 - [c27]Yoav Freund, Michael J. Kearns, Yishay Mansour, Dana Ron, Ronitt Rubinfeld, Robert E. Schapire:
Efficient Algorithms for Learning to Play Repeated Games Against Computationally Bounded Adversaries. FOCS 1995: 332-341 - [c26]Michael J. Kearns:
A Bound on the Error of Cross Validation Using the Approximation and Estimation Rates, with Consequences for the Training-Test Split. NIPS 1995: 183-189 - 1994
- [b2]Michael J. Kearns, Umesh V. Vazirani:
An Introduction to Computational Learning Theory. MIT Press 1994, ISBN 978-0-262-11193-5, pp. I-XII, 1-207 - [j9]Michael J. Kearns, Leslie G. Valiant:
Cryptographic Limitations on Learning Boolean Formulae and Finite Automata. J. ACM 41(1): 67-95 (1994) - [j8]Michael J. Kearns, Ming Li, Leslie G. Valiant:
Learning Boolean Formulas. J. ACM 41(6): 1298-1328 (1994) - [j7]Michael J. Kearns, Robert E. Schapire:
Efficient Distribution-Free Learning of Probabilistic Concepts. J. Comput. Syst. Sci. 48(3): 464-497 (1994) - [j6]David Haussler, Michael J. Kearns, Robert E. Schapire:
Bounds on the Sample Complexity of Bayesian Learning Using Information Theory and the VC Dimension. Mach. Learn. 14(1): 83-113 (1994) - [j5]Michael J. Kearns, Robert E. Schapire, Linda Sellie:
Toward Efficient Agnostic Learning. Mach. Learn. 17(2-3): 115-141 (1994) - [c25]David Haussler, H. Sebastian Seung, Michael J. Kearns, Naftali Tishby:
Rigorous Learning Curve Bounds from Statistical Mechanics. COLT 1994: 76-87 - [c24]Avrim Blum, Merrick L. Furst, Jeffrey C. Jackson, Michael J. Kearns, Yishay Mansour, Steven Rudich:
Weakly learning DNF and characterizing statistical query learning using Fourier analysis. STOC 1994: 253-262 - [c23]Michael J. Kearns, Yishay Mansour, Dana Ron
, Ronitt Rubinfeld, Robert E. Schapire, Linda Sellie:
On the learnability of discrete distributions. STOC 1994: 273-282 - 1993
- [j4]Sally A. Goldman, Michael J. Kearns, Robert E. Schapire:
Exact Identification of Read-Once Formulas Using Fixed Points of Amplification Functions. SIAM J. Comput. 22(4): 705-726 (1993) - [j3]Michael J. Kearns, Ming Li:
Learning in the Presence of Malicious Errors. SIAM J. Comput. 22(4): 807-837 (1993) - [c22]Henry A. Kautz, Michael J. Kearns, Bart Selman:
Reasoning With Characteristic Models. AAAI 1993: 34-39 - [c21]Michael J. Kearns, H. Sebastian Seung:
Learning from a Population of Hypotheses. COLT 1993: 101-110 - [c20]Avrim Blum, Merrick L. Furst, Michael J. Kearns, Richard J. Lipton:
Cryptographic Primitives Based on Hard Learning Problems. CRYPTO 1993: 278-291 - [c19]Michael J. Kearns, Leslie G. Valiant:
Cryptographic Limitations on Learning Boolean Formulae and Finite Automata. Machine Learning: From Theory to Applications 1993: 29-49 - [c18]Yoav Freund, Michael J. Kearns, Dana Ron
, Ronitt Rubinfeld, Robert E. Schapire, Linda Sellie:
Efficient learning of typical finite automata from random walks. STOC 1993: 315-324 - [c17]Michael J. Kearns:
Efficient noise-tolerant learning from statistical queries. STOC 1993: 392-401 - 1992
- [c16]Michael J. Kearns:
Oblivious PAC Learning of Concept Hierarchies. AAAI 1992: 215-222 - [c15]Michael J. Kearns, Robert E. Schapire, Linda Sellie:
Toward Efficient Agnostic Learning. COLT 1992: 341-352 - 1991
- [j2]David Haussler, Michael J. Kearns, Nick Littlestone, Manfred K. Warmuth:
Equivalence of Models for Polynomial Learnability. Inf. Comput. 95(2): 129-161 (1991) - [c14]David Haussler, Michael J. Kearns, Robert E. Schapire:
Bounds on the Sample Complexity of Bayesian Learning Using Information Theory and the VC Dimension. COLT 1991: 61-74 - [c13]Sally A. Goldman, Michael J. Kearns:
On the Complexity of Teaching. COLT 1991: 303-314 - [c12]David Haussler, Michael J. Kearns, Manfred Opper, Robert E. Schapire:
Estimating Average-Case Learning Curves Using Bayesian, Statistical Physics and VC Dimension Methods. NIPS 1991: 855-862 - 1990
- [b1]Michael J. Kearns:
Computational complexity of machine learning. ACM distinguished dissertations, MIT Press 1990, ISBN 978-0-262-11152-2, pp. I-IX, 1-165 - [c11]Sally A. Goldman, Michael J. Kearns, Robert E. Schapire:
On the Sample Complexity of Weak Learning. COLT 1990: 217-231 - [c10]Sally A. Goldman, Michael J. Kearns, Robert E. Schapire:
Exact Identification of Circuits Using Fixed Points of Amplification Functions (Abstract). COLT 1990: 388 - [c9]Michael J. Kearns, Robert E. Schapire:
Efficient Distribution-Free Learning of Probabilistic Concepts (Abstract). COLT 1990: 389 - [c8]Sally A. Goldman, Michael J. Kearns, Robert E. Schapire:
Exact Identification of Circuits Using Fixed Points of Amplification Functions (Extended Abstract). FOCS 1990: 193-202 - [c7]Michael J. Kearns, Robert E. Schapire:
Efficient Distribution-free Learning of Probabilistic Concepts (Extended Abstract). FOCS 1990: 382-391
1980 – 1989
- 1989
- [j1]Andrzej Ehrenfeucht, David Haussler, Michael J. Kearns, Leslie G. Valiant:
A General Lower Bound on the Number of Examples Needed for Learning. Inf. Comput. 82(3): 247-261 (1989) - [c6]Michael J. Kearns, Leonard Pitt:
A Polynomial-Time Algorithm for Learning k-Variable Pattern Languages from Examples. COLT 1989: 57-71 - [c5]Michael J. Kearns, Leslie G. Valiant:
Cryptographic Limitations on Learning Boolean Formulae and Finite Automata. STOC 1989: 433-444 - 1988
- [c4]David Haussler, Michael J. Kearns, Nick Littlestone, Manfred K. Warmuth:
Equivalence of Models for Polynomial Learnability. COLT 1988: 42-55 - [c3]Andrzej Ehrenfeucht, David Haussler, Michael J. Kearns, Leslie G. Valiant:
A General Lower Bound on the Number of Examples Needed for Learning. COLT 1988: 139-154 - [c2]Michael J. Kearns, Ming Li:
Learning in the Presence of Malicious Errors (Extended Abstract). STOC 1988: 267-280 - 1987
- [c1]Michael J. Kearns, Ming Li, Leonard Pitt, Leslie G. Valiant:
On the Learnability of Boolean Formulae. STOC 1987: 285-295
Coauthor Index
aka: Jennifer Wortman

manage site settings
To protect your privacy, all features that rely on external API calls from your browser are turned off by default. You need to opt-in for them to become active. All settings here will be stored as cookies with your web browser. For more information see our F.A.Q.
Unpaywalled article links
Add open access links from to the list of external document links (if available).
Privacy notice: By enabling the option above, your browser will contact the API of unpaywall.org to load hyperlinks to open access articles. Although we do not have any reason to believe that your call will be tracked, we do not have any control over how the remote server uses your data. So please proceed with care and consider checking the Unpaywall privacy policy.
Archived links via Wayback Machine
For web page which are no longer available, try to retrieve content from the of the Internet Archive (if available).
Privacy notice: By enabling the option above, your browser will contact the API of archive.org to check for archived content of web pages that are no longer available. Although we do not have any reason to believe that your call will be tracked, we do not have any control over how the remote server uses your data. So please proceed with care and consider checking the Internet Archive privacy policy.
Reference lists
Add a list of references from ,
, and
to record detail pages.
load references from crossref.org and opencitations.net
Privacy notice: By enabling the option above, your browser will contact the APIs of crossref.org, opencitations.net, and semanticscholar.org to load article reference information. Although we do not have any reason to believe that your call will be tracked, we do not have any control over how the remote server uses your data. So please proceed with care and consider checking the Crossref privacy policy and the OpenCitations privacy policy, as well as the AI2 Privacy Policy covering Semantic Scholar.
Citation data
Add a list of citing articles from and
to record detail pages.
load citations from opencitations.net
Privacy notice: By enabling the option above, your browser will contact the API of opencitations.net and semanticscholar.org to load citation information. Although we do not have any reason to believe that your call will be tracked, we do not have any control over how the remote server uses your data. So please proceed with care and consider checking the OpenCitations privacy policy as well as the AI2 Privacy Policy covering Semantic Scholar.
OpenAlex data
Load additional information about publications from .
Privacy notice: By enabling the option above, your browser will contact the API of openalex.org to load additional information. Although we do not have any reason to believe that your call will be tracked, we do not have any control over how the remote server uses your data. So please proceed with care and consider checking the information given by OpenAlex.
last updated on 2024-11-20 21:01 CET by the dblp team
all metadata released as open data under CC0 1.0 license
see also: Terms of Use | Privacy Policy | Imprint